Can neuromorphic vision accelerate the arrival of intelligent robots?
10-05-2021 | By Paul Whytock
We all know that when it comes to processing gargantuan amounts of data computers are unbeatable and that industrial robots are champions at coping with mind-numbingly repetitive tasks requiring unrelenting accuracy and energy.
However, despite all that, humans remain dominant at one critical thing that neither of those technologies can handle and that is the ability to recognise, interpret, analyse and intelligently learn from information gathered via data and real-time practical experiences.
But could all that change if the potential promises that neuromorphic vision technology purports to offer become working realities? This form of vision is defined as the development of artificial systems and circuits that exploit information typically found in the human eyes biological systems.
Scientists believe progress in neuromorphic technology relative to artificial vision will improve how robots understand and use visual information via image segmentation, visual attention and object recognition in a similar way to humans. But let’s be clear, such robotic vision capability can only be achieved if the robot’s “brain” has enormous computational power.
To understand how this could be achieved a wide variety of technologies and concepts are being explored and tested by numerous companies and scientific organisations.
And this has been going on since the 1980s when American scientist and electronics professor Carver Mead, a pioneer of modern microelectronics who contributed to the foundations of very-large-scale integration (VLSI) processor design, decided to focus on electronic modelling of human neurology and biology and explore the use of VLSI in electronic circuits that imitate the neuro-biological architectures present in human.
But in addition to VSLI-based technologies there are many other sciences involved such as anisotropic diffusion, neuromorphic vision sensors, asynchronous transmission of signals protocols and the development of algorithms to handle the information generated. Also considered is the use of non-volatile memristors.
One of the difficulties in developing neuromorphic systems is the von Neumann Bottleneck. This is where data flow through a computer’s architecture is held up. Whereas processor speeds have increased significantly the developments in memory technology have been about density, the ability to store more data. This is fine, but what has not happened is the development of memories that hold greater amounts of data and can transfer it rapidly. The bottleneck comes therefore when processors are held up by slow data transfer from memory.
However, recent developments in transistor-less all-analogue networks may solve the von Neumann bottleneck inherent in today’s digital computers.
Researchers Suhas Kumar of H-P Laboratories, R. Stanley Williams with Texas A&M University and the late Stanford PhD student Ziwen Wang recently introduced a paper looking at an isolated nanoscale electronic circuit element that can perform non-monotonic operations and transistor-less all-analogue computations. With input voltages, it can output simple spikes and an array of neural activity such as bursts of spikes, self-sustained oscillations, and other brain activities. Industry experts consider the development of transistor free networks as a key breakthrough in neuromorphic computing.
In addition to that, substantial work has been ongoing to make neuromorphic vision a reality. For example, back in 2011, MIT researchers created a computer chip that mimics the analogue, ion-based communication in a synapse between two neurons using 400 transistors and standard CMOS manufacturing techniques. A year later Purdue University presented the design of a neuromorphic chip using lateral spin valves and memristors. This particular architecture mimicked neurons and can replicate the human brain's processing methods.
A research project with implications for neuromorphic vision is the Human Brain Project that is attempting to simulate a complete human brain in a supercomputer using biological data. It is made up of a group of researchers in neuroscience, medicine, and computing and the project has been funded to the tune of $1.3 billion by the European Commission.
Large corporations are also keen to develop neuromorphic technologies. For example, Intel has its neuromorphic research chip called Loihi. The chip uses an asynchronous spiking neural network (SNN) to implement adaptive self-modifying event-driven fine-grained parallel computations used to implement learning and inference with high efficiency.
Intel believes the computational building blocks within neuromorphic computing systems are logically analogous to neurons. Therefore, SNNs are a suitable model for arranging those elements to emulate natural neural networks that exist in biological brains. For example, each neuron in the SNN can act independently of the others and send pulsed signals to other neurons in the network that directly change the electrical states of those neurons.
By encoding information within the signals themselves and their timing, SNNs simulate natural learning processes by dynamically remapping the synapses, where the between artificial neurons in response to stimuli. The synapse is a small gap at the end of each neuron that allows a signal to pass from one neuron to the next.
To provide functional systems for researchers to implement SNNs, Intel Labs designed Loihi, its fifth-generation self-learning neuromorphic research test chip. This 128core design is based on a specialised architecture that is optimised for SNN algorithms and fabricated on 14nm technology. This chip supports the operation of SNNs that do not need to be trained in the conventional manner of a convolutional neural network.
It also includes 130,000 neurons and developers can access and manipulate on-chip resources by means of a learning engine embedded in each of the 128 cores. Furthermore, because the hardware is optimised specifically for SNNs it supports accelerated learning in unstructured environments for systems that require autonomous operation and continuous learning with extremely low power consumption coupled with high performance and capacity.
IBM are also an avid competitor in the neuromorphic vision and computing race. The company’s TrueNorth device is created on a CMOS circuit and has 4096 cores and each of these has 256 programmable simulated neurons making over a million neurons.
Each neuron has 256 programmable synapses that permit a neuron to pass an electrical signal to other neurons. This creates 268 million programmable synapses. What is attractive about the TrueNorth development is its power efficiency. IBM claim a power consumption of 70 milliwatts.
In Finland, the research centre VTT is coordinating an EU project working on a neuromorphic related technology. Called the MISEL (Multispectral Intelligent Vision System with Embedded Low-Power Neural Computing) project the aim is to develop a fast, reliable and energy-efficient machine vision system that could be used in devices such as drones, industrial and service robots and surveillance systems.
In addition to algorithms, the project is developing new devices for perception and analysis work. The first component in the MISEL system is a photodetector built with quantum dots that is sensitive to visible and infrared light and just like the human retina the sensor selects and compresses data and forwards it to the next component in the MISEL system that emulates the human cerebellum and provides instructions to create suitable reflex responses. The third component in the chain is a processor imitating the cortex. This will provide a deeper analysis of data and instructs the sensor to focus on objects of interest. The project aims to employ machine learning methods to anticipate chains of events.
But to return to the headline question on whether or not neuromorphic technology could help create the thinking robot, I believe it’s still a very long way off and may in fact never happen. However, there is no doubt that neuromorphic computing could boost current artificial intelligence (AI) capabilities. AI currently has a developmental weakness because it is reactive. It depends on being able to take enormous quantities of data and then algorithms are developed to work with that data to produce a desired end result.
Neuromorphic vision and computing would change all that and allow AI to develop its own reaction to any given information. It would replicate the human brain and start to analyse and react to what it sees.
The really big question is not whether the technology is a feasible ambition. It's whether we humans really want robots that think, react and adapt to situations in a distinctly faster and superior way than we do?
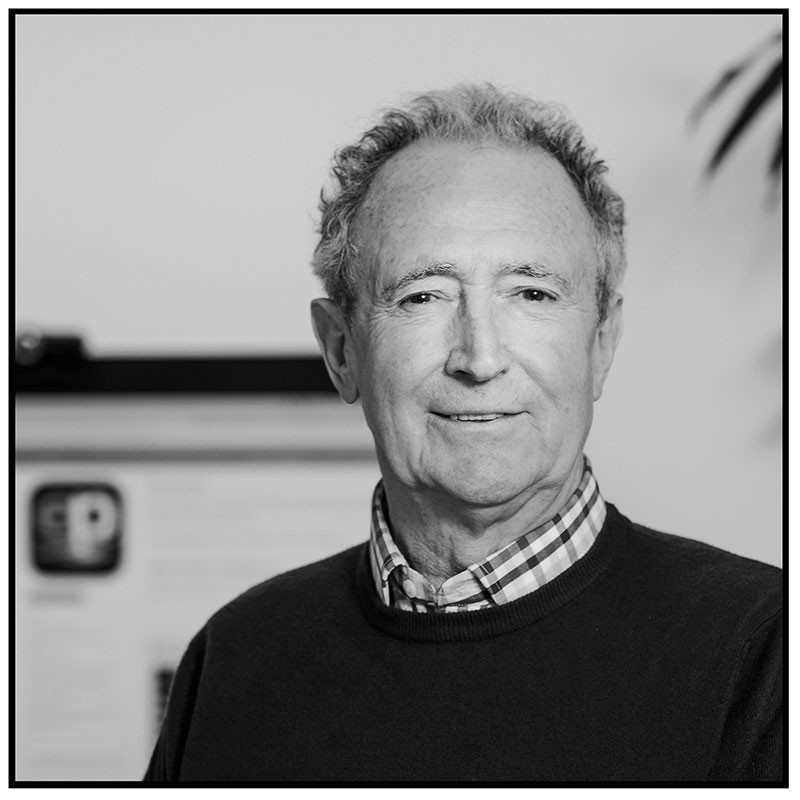