Ferroelectric Photosensor for Machine Vision Applications
19-04-2022 | By Liam Critchley
Machine vision is a type of technology that has been developed so that machines can ‘see’ and ‘understand’ the world around them. Machine vision technologies have already been applied to many different industries and applications that affect everyday life. Now, there is a drive to develop machine vision systems for autonomous driving applications.
Machine Vision Systems To Date for Autonomous Driving
Many machine vision systems are designed to capture the information being analysed by the machine using a photosensor array. The information is then transformed into a digital signal and transferred to a computing unit, where an image is recreated from the obtained digital signals. While many machine vision systems are used in everyday life, for them to be used in autonomous vehicles—and adapt in real-time to hazards—they need to be very responsive to changes in their surroundings.
However, in many machine vision systems, the shuttling of redundant data between the separated image sensing and processing units can cause issues, especially a high latency. This means that some machine vision systems are not quick enough for the real-time environment in autonomous driving applications as they can’t track nearby objects quickly enough. Beyond this, the shuttling of data can also cause a high energy consumption, making some of the systems commercially unfeasible in their current state.
In recent years, bio-inspired neuromorphic visual systems have been explored to overcome the latency and high energy consumption issues of machine vision systems by adopting near or in-sensor computing architectures to reduce the levels of data shuttling. There are already a number of different functions that neuromorphic systems have been designed for, ranging from contrast enhancement to adaptive imaging, and many more in between.
Across the class of neuromorphic visual systems, the ones which utilise a reconfigurable photosensor network alongside an in-sensor computing architecture have been the most successful because they can operate on their own using artificial neural networks (ANN) to simultaneously sense and process images in the local environment.
One of the key considerations when designing these photosensor networks is to use a photorsensor with a tuneable photoresponsivity. A number of materials have been trialled, including different nanomaterials, but many have consumed a lot of power or have been relatively slow and unreliable. So, new tuneable photoresistors are now starting to be designed for these neuromorphic machine vision systems, and ferroelectric photosensors are being trialled as a potential option.
The Emergence of Ferroelectric Photosensor Networks in Machine Vision Systems
Ferroelectric photosensors have emerged as a potential solution to the problem of finding a suitable reconfigurable photosensor for machine vision applications, especially for applications such as autonomous driving, where performance is key.
There are several reasons why ferroelectric photosensors are seen as the solution. Firstly, they have a remanent polarisation that can be utilised to tune the photovoltaic response in the photosensor, turning them into a gate voltage-free and self-powered reconfigurable photosensor. Moreover, the switching of the polarisation can also change the sign of the photoresponse (as well as its magnitude), so a single photosensor can perform both image capturing and processing operations, reducing the amount of hardware required. Ferroelectric photosensors also possess high controllability, non-volatility and ultrafast switching kinetics, which enable them to have high reliability and write speed. From this, photosensor networks can be built using an in-sensor circuit with multiple ferroelectric photosensors and offer a promising solution for real-time machine vision applications.
Until recently, only the memory side of these photoresistor networks had been investigated, but new research has emerged that has created ferroelectric photosensor networks that can perform both the sensing and the processing functions simultaneously. These ferroelectric photosensor networks have also been found to have another benefit. Once you start to connect multiple photosensors together into the same device, the photosensor networks begin to behave like an artificial neural network, so they have the potential to be self-regulating and self-operational as well.
In the specific system developed recently, the photosensor network utilised a platinum/lead/zirconium ferroelectric layer known as PZT. This material was chosen for its large remanent polarisation and controllable and robust photoresponse. The properties of the PZT film enabled the researchers to build photosensors that were highly reliable, self-powered and could exhibit both positive and negative photoresponsivities (which is the key property for reducing the amount of required hardware).
The researchers wired multiple ferroelectric sensors into a photosensor network and found that the network could perform in-situ multiply-accumulate (MAC) operations between an input image and a photoresponsivity matrix. The network was then developed further, and real-time image processing functionalities were implemented into the device, including binary pattern classification and edge detection. It was found that the network was faultless when real-time processing images of a binary classification (between ‘X’ and ‘T’ patterns) and had a 100% accuracy.
This new photosensor network is the first type of neuromorphic device that can perform both sensing and signal processing operations. The ability to control the photovoltaic operation via its polarisation, alongside an ultrafast photocurrent generation process, and in-sensor computing architecture, means that there is the potential to develop a neuromorphic device that doesn’t suffer from high energy and high latency issues. Studies such as this are now opening the door for developing new photosensor networks that could eventually be used to build the reliable, high-speed, and low-power hardware that is required for real-time machine vision systems in demanding application environments—such as in autonomous vehicles.
Reference:
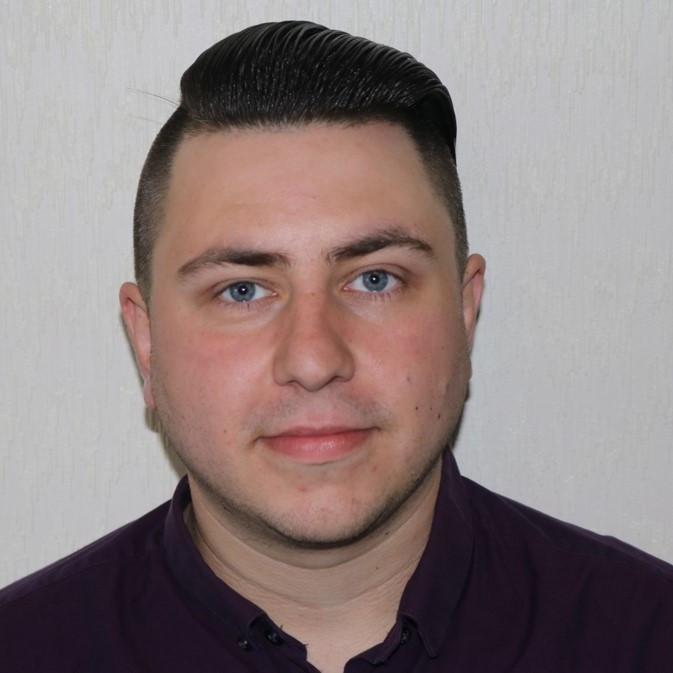