Do I Know You? How Flawed is Facial Recognition?
Privacy | 22-08-2022 | By Paul Whytock
I Live in London, which means that when I move around the city, be it for business meetings, shopping or sightseeing, I will be photographed over 200 times in one day.
This will be by a variety of devices that can be government or police surveillance, business security, or private security systems used by property owners.
Surveys have put the number of CCTV cameras in London at around 870,000, although getting a precise figure is difficult because not all cameras are officially registered.
So, do I mind this invasion of my privacy? The blunt answer is that it doesn’t matter how I feel; it’s still going to happen. However, a consoling thought is that if I behave myself, then so what? Nothing is going to happen to me. The problem is that’s not always true, thanks to facial recognition identity errors. And there’s been plenty of them.
The reason for that is the operational failures of the artificial intelligence (AI) software running many of the security and police surveillance cameras that process and identify the facial recognition images they capture and store, all of them without our prior permission.
Let’s forget the privacy and individual rights issues for the moment. What about these AI inadequacies?
There are operational aspects relative to facial recognition that make developing a reliable AI-operated system challenging, and these are to do with the infinite number of factors that can influence a human face.
Amongst these are emotional changes to expression that affect facial muscles, eye shapes and skin contours. Add to those the changes made by the ageing process, variations in ambient lighting or lack of it and, finally, a technical and socially challenging factor, different skin pigmentations.
To try and deal with these, sensors divide the face into several reference points. These include dimensions such as the distance between each eye pupil, the centre line and width of the nose, the position of the mouth relative to the eyes and nose and many others.
Ideally, these measurements should create a facial specification as individual as your fingerprint. It’s fair to say these facial prints are a pretty accurate way of recognising a human face but not so smart at cross-referencing it to an identity picture, such as could be on criminal files or passports and driving licenses. This is particularly crucial regarding the security and criminal applications of AI.
So AI can and does make mistakes, but it has one significant advantage over humans when it comes to learning from them. Whereas humans, on occasion, can be defensive about admitting errors, AI absorbs the mistake and self-corrects, which is one of the principles of deep learning.
It’s also true that AI is only as good as the diet it is fed to ensure that its deep-learning abilities are fully exploited. It must absorb a varied and accurate diet of data examples or datasets as they are known. AI will fail in certain applications without a diverse input of data, but more on that later.
Every time AI accurately matches two facial images, it remembers the process, so when it comes to accuracy, AI facial recognition can score close to 100%.
How accurate are facial recognition systems?
The National Institute of Standards and Technology (NIST) in America conducted tests on facial recognition systems that digitally capture facial images and then cross-reference them against a photograph such as a passport or driving licence picture, indicating an accuracy of 99.3%.
But don’t forget this level of accuracy can only be achieved in ideal conditions, and as already mentioned, a flexible, constantly moving human face, variations in lighting that is altering from bright to dull and then shadowed and then glaring will undoubtedly bring that accuracy figure down. This is where facial recognition used on the streets by the police to identify criminals has real problems.
NIST’s Face Recognition Vendor Test (FRVT) discovered that Middle-tier algorithms can demonstrate substantial error rates when comparing pictures taken up to twenty years apart.
Middle-tier algorithms are a well-known part of three-tier software architectures and are responsible for processing data. A significant advantage of three-tier architecture is that because each tier runs on its own infrastructure, each one can be developed simultaneously by a separate development team and can be updated when needed without impacting the operation of other tiers.
The Middle-tier is the key central processor of an application where the information collected by the Presentation-tier is processed and compared with other information held in the Data-tier. The Middle-tier can also add, delete or modify data in the Data tier.
The Middle-tier is usually created using Python, Java, Perl, PHP or Ruby and communicates with the Data-tier using application programming interfaces (APIs).
APIs simplify software development by allowing applications to exchange data and functionality efficiently and securely.
Back to the NIST FRVT tests. The results demonstrated a further major issue with facial recognition accuracy—the wide variation between suppliers. NIST’s checks on image verification algorithms found that many facial recognition providers may have error rates several orders of magnitude higher than other known leading companies in this field.
It’s fair to say that many developers can supply very accurate facial recognition algorithms, but many average providers on the market struggle to consistently achieve high levels of accuracy.
Doubts about the accuracy and reliability of facial recognition systems exist not only amongst the general public but also in major corporations. IBM’s CEO, for example, informed the US Congress that IBM firmly opposes the use of facial recognition technology for mass surveillance and racial profiling.
IBM’s withdrawal was followed by Amazon’s announcement of implementing a one-year moratorium on police use of its technology Rekognition. And Microsoft has said it is banning police from using its facial recognition technology until suitable Federal Regulations are established.
So many moral and legal doubts haunting AI facial recognition systems boil down to an inaccuracy.
A further study performed by NIST on 90 commercial facial recognition algorithms showed errors in matching digitally applied face masks with photos of the same person without a mask.
It is essential to understand how facial recognition technology performs
Given the problems of inaccurate facial recognition systems, it is essential to understand how facial recognition technology performs when deployed in a new scenario.
Basically, machine learning is accurate but only within a given sphere of work. Good performance on a specific image data type does not necessarily translate to working well on another type of image data.
When it comes to facial recognition technology (FRT), domain changes are created from the difference between the types of images used by vendors and third-party auditors to train models and test performance and the types of images used by FRT consumers to perform their desired tasks.
While the datasets used by vendors are not disclosed, there is reason to believe that there are substantial differences between vendor and user images, and these may include different face properties, like skin colour, hair colour, hairstyles, glasses, facial hair and age, lighting, blurriness, cropping, quality and the extent of face-covering garments.
Relating to this was a study on the Viola-Jones Face Detection algorithm’s performance on skin pigmentation images. The Viola-Jones algorithm is an object-recognition framework that allows the detection of image features in real-time and is one of the most powerful algorithms of its time.
However, the tests’ results were disappointing regarding the accuracy levels relative to light and dark skin pigmentation. The tests concluded that facial recognition technology is less accurate when trying to identify darker-skinned faces.
A different study which measured how the technology worked on people of different races and gender found three leading software systems correctly identified white men 99% of the time, but the darker the skin, the more often the technology failed.
Darker-skinned women were the most misidentified group, with an error rate of nearly 35% in one test, according to the research conducted by Joy Buolamwini, a computer scientist at the Massachusetts Institute of Technology’s Media Laboratory.
As mentioned previously, AI systems such as facial recognition tools rely on machine-learning algorithms trained on sample datasets.
Therefore if black people are under-represented in benchmark datasets, the facial recognition system will be less successful in identifying black faces.
Given that AI has the ability to learn from its mistakes and absorb the correct operational procedures into its repertoire via its deep-learning characteristics, it would appear to be a major oversight by developers not to incorporate sufficient sample datasets that contained a wide variety of darker skin pigmentation samples when developing AI recognition systems.
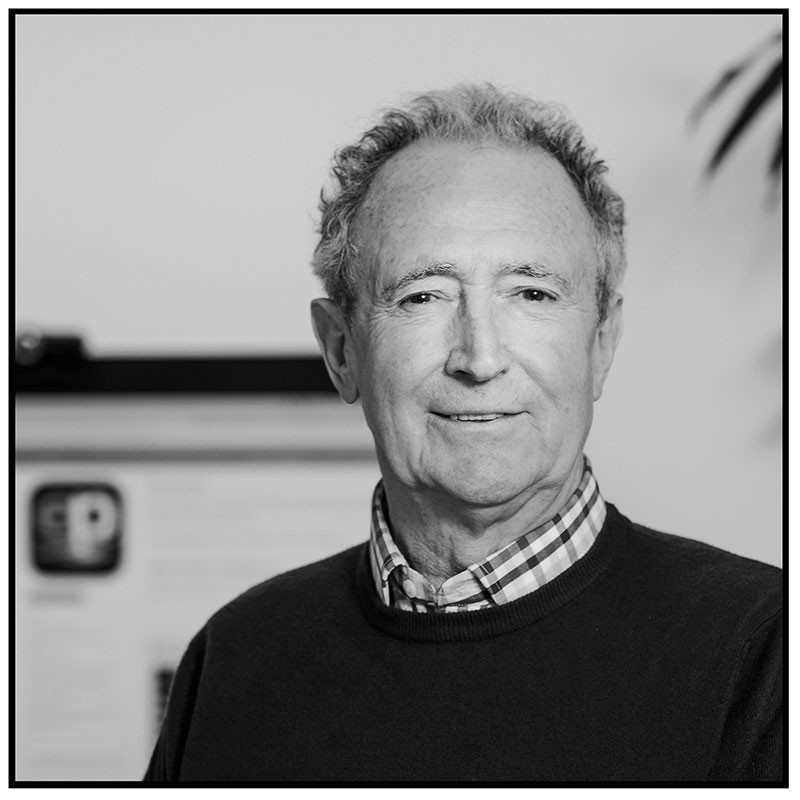