The Promise and Challenges of AI in Early Cancer Diagnosis
16-01-2023 | By Paul Whytock
Deep learning-based Artificial Intelligence (AI) can detect and diagnose certain cancers equally well as qualified humans, and in certain situations, it can do it better, faster and tirelessly.
Expert human radiologists and oncologists often have to look at hundreds of CT scans daily, and human eyesight and fatigue can cause missed or inaccurate diagnoses. AI systems thrive on high-volume repetitive applications, and they can detect tumours as small as 1mm in diameter, which is critical in early diagnosis.
But are they ready for real-world clinical applications? Put AI in a controlled environment where its results are closely monitored by teams of humans, and the answer is yes, but when it comes to the pressurised, mass-diagnosis environment of overworked and stressed hospitals, the answer remains no. And there are a lot of reasons why, including the limitations and ethical considerations surrounding the role of artificial intelligence in early cancer diagnosis.
Impact of Bias & Complexity on Deep-Learning AI in Medical Diagnosis
Deep-learning AI systems learn by consuming massive amounts of data, which can be a fundamental weakness in medical diagnosis. AI will only know what information and the subsequent decisions it makes from the data provided.
Simply put, deep-learning AI generates a system capable of predictive analytics, but it differs from the simpler machine learning systems. Within deep-learning AI systems, the algorithms are categorised and prioritised according to the complexity of the data the system has been fed.
However, if this data is from a specific database, such as a major London-based hospital, it will naturally be biased toward the patients treated at that hospital. Consequently, it may not be sufficiently educated to be applied in a hospital in the Far East where health trends, illnesses and lifestyles can be different.
So AI is only as good as the data it learns from. Fundamentally it doesn’t know what it doesn’t know.
But before going into more of the challenges facing AI when it comes to cancer diagnosis, let’s look at some of the many important positives.
Once fully proven, AI could improve cancer diagnosis and care by providing expert-level analysis to hospitals that, either through lack of finance or remote locations, lack the specialist staff to do the job.
As mentioned earlier, AI systems operating under laboratory-controlled conditions have shown an ability to identify early signs of cancer shown on CT scans more accurately than radiologists.
One case of this was demonstrated at the North Western University’s Feinberg School of Medicine in America.
AI Outshining Trained Medical Experts
Having trained an artificial intelligence system to identify early indications of lung cancer, the system was able to spot tumours in CT scans more accurately than trained medical experts.
But the surprises didn’t end there. The AI system was also fed images of old CT scans of patients who were not diagnosed with cancer at the time of the scan but still developed lung cancer at a later date. The AI detection system identified potential cancers that existed in these scans far earlier than the human experts did at the time... sometimes from CT scans that were two years old.
The University of Oxford is also researching the use of AI to improve lung cancer diagnosis. It is working with the National Health Service Lung Health Check Programme and, for the first time, clinical, imaging, and molecular patient data will be combined using AI algorithms.
It is hoped this way of creating the data that is employed to educate an AI system will accelerate faster and more accurate lung cancer diagnosis and reduce the amount of invasive clinical procedures the patient has to endure.
Uncovering High-Risk Individuals: The Power of Combining Primary Care Data
In addition, the University of Oxford will be linking data from primary care services such as general practice, community pharmacy, dental, and optometry services into the AI system.
The thinking here is this would allow large numbers of citizens to be assessed to identify those at higher risk of developing cancer and should, therefore, be regularly screened.
But there are situations where AI cannot compete with the human mind. This may sound like the start of a negative comment, but it’s not. Humans are incredibly adaptable thinkers with a greater propensity for independent thought and can quickly learn about highly unusual aspects of certain cancer cases. AI cannot do this spontaneously; it has to be taught...by humans.
But what it can do is give doctors time to investigate unusual and complex cases by removing the time-consuming and repetitive load of diagnosing the more routine, everyday cancers that occur.
Overcoming the Challenges
So all of that bodes well for the future of AI in diagnosing cancer, but currently, some serious and possibly insurmountable problems have to be resolved before it can truly enter the real medical world in a fully integrated and trusted way.
One concern is no one knows how long AI algorithms would retain the necessary accuracy for cancer diagnosis. For instance, if CT scanner technology changes and the images it produces are slightly different and perhaps more complex, AI systems would have to be re-educated to analyse them correctly.
The Black Box Question
Fundamentally, the AI Black Box scenario is a situation where a medical expert is provided with a cancer diagnosis by AI, but the AI system cannot provide the rationale behind how it made the diagnosis. This is a serious negative regarding AI cancer diagnosis and could impact getting the right treatments scheduled for patients needing a long-term care plan.
Experts also say this lack of system transparency prohibits critical checks for biases and inaccuracies.
Making AI decisions transparent is very difficult because of how AI makes its highly complex associations from the data it is fed. And it’s not just a case of cleaning the data it is provided.
Because AI deep learning uses neural networks, it’s far more complex than that.
The White Box Approach to AI Creation with Fully Validated Data
Enter the White Box concept of AI. This is where AI creation would only happen with fully validated data that programmers can explain and modify to suit changing AI system requirements is used. In this way, AI would become more reliable, trustworthy and ethical when it comes to human rights.
As mentioned earlier, AI systems cannot mimic the way in which humans absorb and analyse unusual data. This process is out of the AI system’s recognised decision-making zone, and AI cannot behave intuitively like humans.
In order to try and implement this ability into AI systems, there needs to be a lot of analysis performed on the data being fed into the AI system, which is compared to the data and decisions output its algorithms are making. In this way, the AI system’s reaction will be better understood by programmers, and then the AI system could be adjusted to better reflect human attributes.
Ensuring Standards & Fitting AI into Daily Hospital Life
As already mentioned, the successful development of an AI system relies on the substantial input of relevant data. It must be of suitable quality, and as things stand now, there are no recognised standards controlling data quality.
Certain clinical analysis, such as prognosis prediction, is generally more unstructured than typical deep-learning tasks, and the difficulty of this situation is compounded when patients’ data is missing critical clinical details, scans, and medical history.
Another vital issue for AI is how would its diagnostic role fit into daily hospital life. It would be almost impossible to run AI cancer diagnosis without experts, and AI should definitely not be considered an independent solution without any form of human endorsement.
In addition, several aspects of AI use include privacy and safety of patient data which must be considered before the technology is fully implemented into clinical practice.
AI Outclasses Human Experts in Cancer Identification & Characterisation
AI algorithms have been designed to identify and characterise specific types of cancers, and some studies indicate that these algorithms can perform equally and, on occasion, better than human experts.
One study found the ability of an AI system to identify breast cancer from mammograms was comparable to that of radiologists. In addition to that study, a series of tests were recently made to examine the speed and accuracy of AI diagnosis relative to secondary breast cancers. The results showed that AI diagnosis was better when operating under time pressure but was matched by medical clinicians when there were no time constraints.
In another case, LYNA, which is Google’s system for lymph node diagnosis, was equally accurate as oncologists in identifying lymph node metastasis. Not only that, LYNA showed a clear advantage in spotting extremely small cancers.
In tests relative to lung cancer, AI scored an accuracy of 90% when diagnosing common lung cancers, which indicates that AI could very successfully provide a system of a second opinion for medical professionals. Furthermore, the algorithm reliably observed genetic mutations in lung adenocarcinoma, which could help with decisions on early therapy.
Eliminating Bias in Cancer Diagnosis AI Algorithms
Because of the reliance on being fed the correct data, AI algorithms may develop racial or gender biases if the data itself is skewed. One industry example here is IBM’s AI clinical system. It was trained using clinical data sourced in the US, but the AI system is used in Asia. Consequently, its clinical analysis varies from 50 to 80% accuracy when compared to human experts’ analysis of the same patient data.
When it comes to human acceptance of AI cancer diagnosis, some medical experts and patients will naturally harbour feelings of no-confidence towards algorithmic-based diagnosis by AI systems that are newcomers to real-world hospital applications.
Navigating Data Protection & Ethics in AI Cancer Diagnosis
General Data Protection Law is well established and protects individuals who want their personal details removed from databases. Admittedly such requests are generally prompted by personal data security concerns or, perhaps, by a need to avoid being pestered by companies trying to sell them something. However, it’s fair to say that it would be a rare occurrence if a patient asked to be forgotten from a medical database. But nevertheless, data protection laws exist and are rigorously applied, especially in the European Union.
The previously mentioned AI Black box conundrum makes it very difficult for computer programmers to erase content from deep-learning AI systems. One well-known, very large company suffered substantial embarrassment caused by inappropriate and biased data being used to fuel its AI algorithms.
It created an AI system to evaluate the potential of employees to progress within the company. In other words, to spot the managers of tomorrow. Unfortunately, the bad data the AI system had been provided meant it unfairly rated male employees higher than females. Oops.
Most companies creating AI systems are well aware of the pitfalls of inadequate data and are fearful of unethical AI results. In view of this, several years ago, the EU issued its guidelines that defined the ethical AI use of information.
Named the Ethics Guidelines for Trustworthy AI, it is expected to continue to encourage businesses needing to develop an AI system to employ recommended specialist companies to create them rather than just general services providers that only have a superficial knowledge concerning the end application of the AI they create.
Such control on AI system development is crucial when it comes to people’s lives and can only help AI cancer diagnosis in its progression into the real world of medicine.
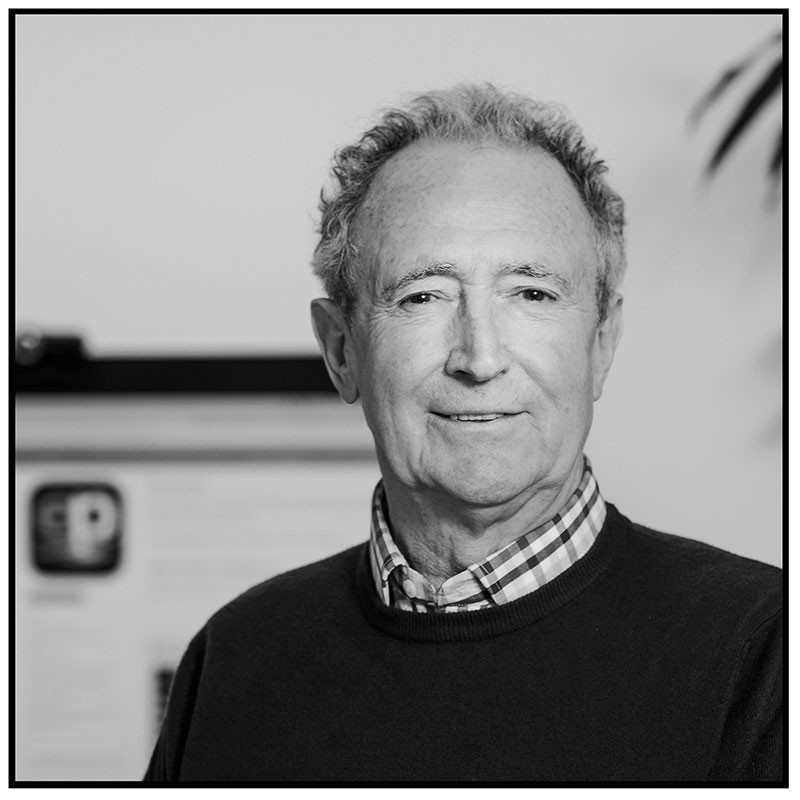