The Power of AI: Exploring ChatGPT's Performance Degradation Over Time
11-08-2023 | By Robin Mitchell
Recently, OpenAI shut down its AI detection tool due to low accuracy. This event highlights the challenge of distinguishing AI-generated content from human-written material. Moreover, a new study has shown a decline in the performance of ChatGPT over time. This article explores the challenges AI faces in explaining itself, the insights from the new report, and the implications for the future of AI. What challenges does AI face when it comes to explaining itself, what did the new report reveal, and is this it for the power of AI?
What challenges does AI face when explaining itself?
As the world enters a new era of technology dominated by AI, no one can be sure of how the future will look. Some believe that AI and automation will give rise to a time of plenty and abundance, eliminating the human factor in work. Others believe that AI be controlled by a minority of society and effectively make massive numbers of people unemployed.
So far, it seems that AI is providing massive benefits to the workplace by performing routine tasks that lack imagination, sparking human creativity by seeding ideas and being able to perform complex analyses on data that traditional algorithms struggle with (such as language tasks). While there is evidence that AI is eliminating the human factor in some jobs, this is still yet to become a dominating factor in the AI industry.
However, there are plenty of concerns when it comes to using AI, such as the legality of results if trained on copyrighted data or the potential to breach individual privacy when given access to personal data. Another concern that continues to grow is the inability of AI to explain its answer and convey how it arrived at its conclusion.
At its core, AI consists of many interconnected layers that link to specific points called nodes. As the AI receives training data, these connections are fine-tuned until the AI's output aligns with the expected result.
For AI to work accurately with new data, it needs varied training data. This helps the AI identify similarities between different data sets. For example, if you show an AI the same picture of an apple one hundred times, it will only recognise that specific picture.
Once trained, an AI can recognise pictures of apples, but unlike humans, it's nearly impossible to ask the AI how it reached its conclusion.
Furthermore, the visualisation of an AI’s neural network would also be incomprehensible to a human, with thousands of connections, weighted nodes, and equations all simultaneously being calculated. Trying to explain an AI’s conclusion would likely be something akin to “because matrix number 734 resulted in a value of 0.23 which caused matrix number 865 to produce a value of 0.56, containing no context whatsoever. This complexity in understanding AI leads us to recent developments in AI detection tools.
But why is this inability to explain itself such a problem for AI? Well, the problem with this is that it becomes extremely difficult to determine if the result from an AI is correct (as it cannot justify its answer). Worse, if the answer is known to be wrong, it is very hard to diagnose the response and fix it without large amounts of training data that can help steer the neural network away from that answer.
Understanding the Complexity: How AI Works and Why It Can't Explain Itself
The inability of AI to explain its answers is rooted in the complex way AI systems function. Essentially, an AI consists of multiple layers of interconnected pathways and weighted nodes. As the AI receives training data, these connections are fine-tuned until the AI's output aligns with the expected result.
To make AI work with new data, it must be trained on a variety of examples. If an AI is shown the same picture of an apple many times, it will only recognise that specific image. But if trained on different pictures of apples, it learns to recognise the common features of an apple.
Once trained, an AI can identify apples in future images, but it's nearly impossible to ask the AI how it reached its conclusion. The complexity of its internal workings, with thousands of connections and calculations, makes it difficult for humans to understand. This inability to explain itself is a significant challenge for AI, making it hard to determine if a result is correct or how to fix it if it's wrong.
OpenAI closes AI detector, and performance falters
Recently, OpenAI announced that it will be closing down its AI detector tool, which it developed to help academics and researchers identify AI content. When ChatGPT was first released, it didn’t take long for students around the world to take advantage of its writing capabilities, and this immediately overwhelmed teachers. As ChatGPT has become extremely human-like, teachers have often struggled with identifying such content and, in some cases, have falsely accused students of cheating when they didn’t.
OpenAI’s recent decision to close down its AI detector tool is a significant development in the field. According to the official announcement, the AI classifier is no longer available due to its low rate of accuracy. The classifier faced challenges in identifying AI-written text, with a true positive rate of only 26% and a false positive rate of 9%. These limitations highlight the complexity of distinguishing between human and AI-generated content, especially with short texts and predictable content.
But while the tool was published with the goal of catching AI content, it has now been shut down due to its poor accuracy. Simply put, the ability of ChatGPT to produce realistic human content has become so good that even OpenAI’s own tools can’t spot the difference. According to OpenAI, they are redeveloping their own checking tools to try and find new methods for spotting AI content but have thus far failed to produce a viable tool.
However, this isn’t the only challenge faced by OpenAI. A new report published by researchers from Stanford University has published verifiable data showing how the performance in ChatGPT has actually degraded over the past year. In their report, they compared the abilities of ChatGPT 4.0 and 3.5 over the past year by testing the AI with various challenges, including identifying prime numbers.
Figure 1: A comparison of the performance between March 2023 and June 2023 versions of GPT-4 and GPT-3.5 across eight distinct tasks: (a,b) tackling mathematical challenges (Prime vs Composite numbers and Happy Numbers), (c) addressing sensitive inquiries, (d) participating in opinion surveys, (e) utilising a LangChain app for multi-step question answering, (f) creating executable code, (g) performing on the USMLE medical examination, (h) engaging in visual reasoning exercises. Within each task, an example is depicted in a purple box, with the total number of examples, denoted by 'n', included in the caption. Over time, the models' performance has shown significant fluctuations, occasionally deteriorating.
One such example was with calculating prime numbers. It was found that in March 2023, ChatGPT-4 was able to correctly guess if the number 17077 was prime, while ChatGPT-3.5 would often struggle. However, in June 2023, this result flipped where ChatGPT-3.5 would get the answer correct, but ChatGPT-4 would fail most of the time. It was also found that while ChatGPT-3.5 saw both performance improvements and reductions, ChatGPT-4 outright saw worse performance across the board.
How does this link back to the challenge of AI explaining itself? Well, as ChatGPT is unable to explain how and why it comes to its conclusions, there is no real way of knowing what training data resulted in the reduction in performance.
The Impact on Educators and the Future of AI Detection
The challenges of identifying AI-written text have sparked discussions among educators, journalists, and researchers. OpenAI has engaged with these communities to understand the implications and is actively working on developing more effective provenance techniques. The ongoing research and collaboration with affected communities reflect a commitment to deploying large language models safely and responsibly.
Recent findings from a Stanford University research paper have further emphasised the complexity of this challenge. The study demonstrated that the behaviour of GPT-3.5 and GPT-4 has varied significantly over a relatively short amount of time. This variation underscores the need to continuously evaluate and assess the behaviour of large language models (LLMs) like ChatGPT, especially as it is not transparent how they are updated over time.
The research also highlighted the difficulty of uniformly improving LLMs’ multifaceted abilities. Efforts to enhance the model’s performance on specific tasks can lead to unexpected side effects in other areas. For example, both GPT-3.5 and GPT-4 experienced declines in performance on some tasks while improving in others. These divergent trends and shifts in the models’ chain-of-thought behaviours and verbosity present unique challenges for developers and users alike.
The researchers have expressed their intention to continue monitoring GPT-3.5, GPT-4, and other LLMs on diverse tasks over time. They also recommend that users or companies relying on LLM services implement similar monitoring analyses for their applications. This ongoing vigilance and collaboration between researchers, developers, and users are essential for ensuring the responsible and effective deployment of AI technologies in various fields, including education.
Is this it for the power of AI?
There is no doubt that as computation power increases, the capabilities of AI will also increase, so the performance decline seen by ChatGPT is unlikely due to the physical limitations of AI. While the exact internal operations of ChatGPT are not exactly public, it is highly likely that user-submitted corrections and feedback help to train the language model, meaning that there are likely to be vast quantities of incorrect feedback that will only confuse the AI.
Furthermore, it should also be understood that ChatGPT has likely been artificially altered to produce outputs that conform to its rule set (such as not producing discriminating content). While this makes ChatGPT a more friendly AI, it does have the potential to impact its capabilities. As AI systems cannot explain their results, there is no way of telling how these rules impact the predictive system.
Overall, it is clear that AI is becoming an increasingly powerful tool, and tools designed to identify such content are becoming less capable. Even using AI to identify AI is failing due to the ability of AI to come across as human.
Conclusion
The ever-changing world of artificial intelligence presents both remarkable opportunities and complex challenges. Recent developments, such as the fluctuating performance of models like ChatGPT and the intricacies of AI detection tools, highlight the need for continuous research, understanding, and responsible management. As we embrace the power of AI, we must also recognise its limitations and work collaboratively to navigate them. The future of AI is filled with potential, but it requires a thoughtful approach to fully harness its benefits without succumbing to its complexities.
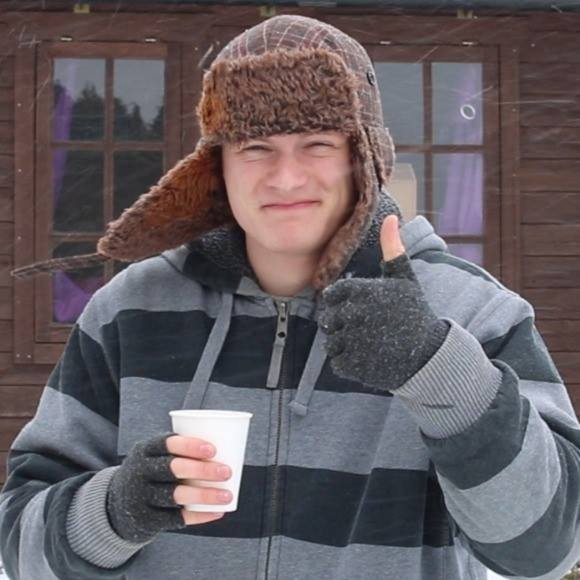