AI in Meteorology: How Google's DeepMind Enhances Forecasting
Insights | 12-12-2023 | By Robin Mitchell
As the power of AI continues to increase, a recent announcement from Google has demonstrated how their AI-powered weather prediction tool can perform better than current models. What challenges does weather prediction introduce, what did Google demonstrate, and what does this mean for AI in weather prediction going forward?
What challenges does weather prediction face?
For as long as humans have existed, predicting the weather has been one of the most important aspects of life. The ability to predict weather allows for not only immediate life-saving decisions to be made (such as avoiding flooded areas) but also those that span decades. Good weather allows for crops to grow, bad weather can kill those same crops, and disastrous weather can wipe out food stores, homes, and, if you believe the tales of Atlantis, an entire civilization.
However, forecasting the weather is a task laden with challenges rooted in the inherent complexity of the Earth's atmosphere. Understanding why predicting weather is difficult involves dissecting various factors that contribute to the intricate nature of atmospheric dynamics.
At its core, weather is a product of numerous interacting elements within the atmosphere. Temperature, pressure, humidity, and wind patterns are just a few of the variables that influence weather conditions. The challenge arises from the fact that these elements don't act in isolation; they are part of a dynamic and interconnected system where changes in one variable can trigger a cascade of effects on others.
The concept of chaos theory, introduced by Edward Lorenz, adds another layer of complexity to weather prediction. Small variations in initial conditions can lead to significantly different outcomes, making long-term forecasts particularly challenging. This inherent sensitivity to initial states, often referred to as the butterfly effect, underscores the difficulty of precisely predicting the future state of the atmosphere.
Spatial and temporal variability further complicates the forecasting landscape. Weather systems unfold on different scales, from local weather patterns to larger climatic trends, and capturing this variability requires sophisticated models and computational power. While technological advancements have improved our ability to simulate atmospheric processes, achieving the granularity needed for accurate predictions remains an ongoing challenge.
Observing the atmosphere presents its own set of difficulties. Despite advancements in satellite and radar technologies, there are still gaps in our observational network, particularly in remote or inaccessible areas. The accuracy of weather predictions hinges on the availability of comprehensive and precise observational data.
Meteorologists also grapple with the inherent uncertainty of probabilistic forecasting. Weather conditions exist on a spectrum of possibilities rather than a deterministic outcome. Communicating this uncertainty to the public requires a delicate balance to avoid causing unnecessary alarm while providing useful information.
Overall, the challenges of weather prediction stem from the intricate interplay of atmospheric variables, the sensitivity to initial conditions, the spatial and temporal variability of weather systems, limitations in observational capabilities, and the inherent uncertainty in predicting complex, dynamic systems.
Google’s DeepMind AI outperforms conventional weather prediction models
Recognising the challenges faced with current weather prediction tools, Google has recently developed a new climate prediction tool whose accuracy is greater than that of existing conventional tools in use.
The AI, called GraphCast, was trained on vast amounts of climate data from 1979 to 2017, including physics models. By training on this data, the AI can identify links between numerous climate factors, including air pressure, wind direction, and temperature, without being explicitly told how these factors interact with each other. Once trained on this dataset, the model then examines the past 6 hours of live weather data, uses that data to make a prediction 6 hours ahead, and then gathers real-world results to fine-tune its capabilities.
Now, the team have been able to demonstrate accurate prediction results up to ten days in advance, but what makes this ability impressive isn’t its accuracy but the fact that it can make these predictions in a few minutes on a desktop machine instead of a large data centre taking hours. In fact, around 90% of the time, the AI was able to outperform the ECMWF’s High RESolution forecasting system (HRES), which itself takes hours to compute.
GraphCast's efficiency is not its only remarkable feature. Its ability to predict extreme weather events earlier than traditional models is a game-changer. For instance, GraphCast can accurately track cyclone movements and identify atmospheric rivers associated with flood risks. This early warning capability is crucial for disaster preparedness and could potentially save lives. Furthermore, GraphCast's proficiency in predicting extreme temperatures is increasingly vital in our warming world, offering a new tool in anticipating and managing heatwaves.
What does this mean for AI going forward?
This demonstration of AI in action yet again proves that AI is hard to beat when given enough data. Furthermore, it also goes to show that in extremely complex systems where equations cannot be easily used to describe the behaviour can do extremely well with AI.
But it is not just climate prediction where AI will become essential; AI could be massively beneficial across many industries, including medical diagnosis, construction, electronics design, and even the design of future AI systems.
Right now, the capabilities of AI are being limited by two main factors: the lack of data and processor performance. As time progresses, these two factors will diminish in importance, and AI will continue to accelerate, eventually becoming the dominant technology on Earth.
The implications of AI advancements in weather forecasting extend beyond mere predictions. By open-sourcing GraphCast's code, Google DeepMind has paved the way for global collaboration and innovation in this field. This move allows scientists and forecasters worldwide to tailor the model for specific weather phenomena and optimize it for different regions. As AI continues to evolve, its integration into weather forecasting heralds a new era of precision and efficiency, fundamentally transforming our approach to understanding and predicting the Earth's complex weather systems.
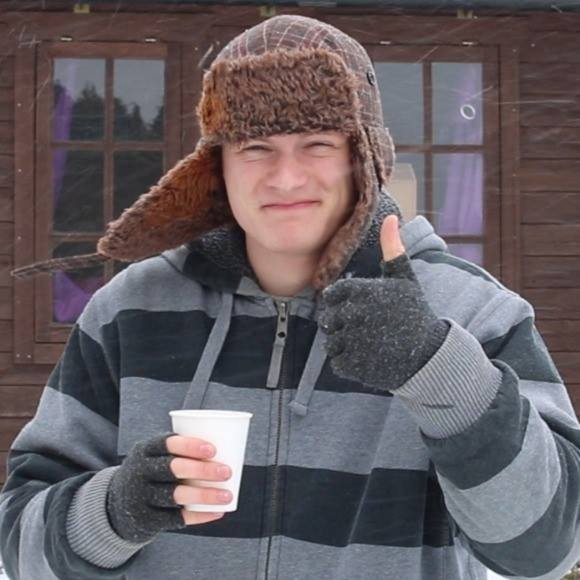