Brainoware: Pioneering AI and Brain Organoid Fusion
19-01-2024 | By Robin Mitchell
Things to know:
- Brainoware Integration: Researchers have developed 'Brainoware', a novel biocomputer that combines brain cells with electronic circuits, potentially revolutionising AI efficiency and processing power.
- AI vs. Human Brain: Traditional AI faces challenges in energy consumption and processing power, whereas Brainoware mimics the human brain's efficiency and complexity.
- Unsupervised Learning: Brainoware utilises unsupervised learning through adaptive reservoir computing, enhancing AI's learning and adaptability.
- Ethical Considerations: The use of living brain cells in AI raises ethical questions about consciousness and the moral implications of such technology.
AI has proven itself to be immensely important over the past few years, and while engineers continue to develop increasingly powerful models, a team of researchers have decided to go in a different direction by combining brain cells with electronic circuits. What challenges do typical AI systems face compared to the human brain, what did the researchers demonstrate, and what ethical considerations does this design face?
What challenges do typical AI systems face compared to the human brain?
The importance of AI and how much it has changed everyday life cannot be understated. What started out as a trivial system incapable of running the most basic tasks has now become so complex that it can be used to replicate voices, write stories, and even make decisions for us. In fact, as technology continues to progress, it is likely that AI's capabilities will also rapidly accelerate, and there is a very real chance that it could replace humans in most job positions by the end of the century.
But for all the benefits that AI systems present, they are still faced with numerous challenges that hold back AI development and capabilities. One such challenge is the concerns surrounding data access and privacy, with major AI companies facing numerous legal challenges as a result of collecting data from users without their full understanding and consent.
Another challenge faced by AI is the ethical considerations behind its use. While it has proven to be massively helpful, there are those who have serious concerns over its ability to replace humans in jobs. For example, AI has managed to replace those working in manual labour positions (such as factory workers and warehouse management) and also in some creative industries, including writing and artwork.
However, by far, the biggest challenge currently faced by AI is the amount of energy needed to make it work and the sheer processing power involved. Unlike traditional programming methods utilising linear code, AI systems are generally built around neural nets that behave similarly to the human brain, with nodes forming connections to other nodes and basic mathematical rules inside nodes determining how to respond to input signals.
Despite the trivial nature of individual nodes, the massive number of nodes and connections needed to make AI practical (along with the need for vast amounts of training data) means that practical AI systems require entire data centres to operate efficiently. This is why most AI applications found on computers, smartphones, and IoT devices almost always rely on an internet connection to a remote data centre where AI algorithms are executed in the cloud.
The nature of AI computation (being highly parallel) also means that traditional CPUs are not suitable for training and execution. Instead, GPUs are preferred due to their ability to perform multiple polynomial calculations in parallel, and such hardware is not only power-hungry but expensive. Thus, trying to replicate nature in hardware is not only a massive technical challenge but also a resource-intensive one.
Researchers create a biocomputer from brain tissue and circuits
It takes an entire data centre to power software such as ChatGPT, requiring megawatts of power, and yet, this article is written by a human brain, which only requires a fraction of the energy provided by a roast dinner the previous day. As such, is it possible for an AI to be powered not by artificial intelligence but by actual intelligence?
This question is at the heart of a pioneering study by Indiana University researchers. They have developed a novel biocomputer, 'Brainoware', which marries biological brain tissue with electronic components. This hybrid system represents a significant leap in AI development, potentially addressing the energy efficiency and processing power limitations of current AI models.
Well, a team of researchers believes so and has recently built a bio-computer that combines brain tissue with electronic circuits to create a hybrid system. The system, which has been given the name “Brainoware”, integrates bundles of lab-grown human brain cells into a device with thousands of electrodes, and by applying electrical signals to the growth, the neurons react to the signals and produce their own electrical pattern.
To better illustrate this groundbreaking development, let's take a closer look at 'Brainoware'. The following figure, Fig. 1, showcases the biocomputer's structure and its capability for unsupervised learning, a key component in its AI computing process. This visual representation helps in understanding how the integration of brain tissue and electronic circuits is achieved in this innovative system.
Brainoware's Role in Unsupervised AI Learning. a, Illustration of Brainoware as an adaptive reservoir computing system. b, Design overview of the Brainoware configuration, featuring a single brain organoid positioned on a high-density multielectrode array (MEA) to facilitate input reception and output transmission. c, Comprehensive immunostaining of cortical organoids reveals intricate three-dimensional neuronal networks encompassing diverse brain cell types (such as mature neurons indicated by MAP2, astrocytes by GFAP, early-stage neurons by TuJ1, and neural progenitor cells by SOX2). d, Conceptual diagram showing Brainoware's proposed mechanism for unsupervised learning, involving the reshaping of the brain-neural network (BNN) during training, and the suppression of this learning process when synaptic plasticity is inhibited. Scale bar set at 100 μm. Attribution: Nature Electronics (2023), DOI: 10.1038/s41928-023-01069-w.
Advancements in Brain-Inspired Computing
The 'Brainoware' system leverages the unique properties of brain organoids – three-dimensional cultures that closely mimic the complexity and functionality of the human brain. These organoids are capable of processing information in a way that traditional AI systems cannot, potentially leading to more efficient and advanced AI applications.
By recording the resulting patterns and decoding that pattern with the use of AI, it becomes possible to recognise how to match input signals to get a desired output signal. The use of electrical input pulses also trains the neurons to behave in a particular way, thus allowing the mass of neurons to retain data.
To demonstrate the capability of the device, the researchers trained the neurons on 240 recordings of 8 people speaking and turned each recording into a series of electrical impulses delivered to the neurons. After repeated training cycles, the resulting device was able to identify different speakers with an accuracy of 78%.
This achievement is not just a technical marvel but also a step towards understanding the intricate workings of the human brain. By integrating brain cells into computing, researchers can gain insights into neural processing and potentially unlock new methods to treat neurological disorders.
While the device has its drawbacks (such as the need to keep the cells alive), it demonstrates how brain cells can be used to process information using far less energy than current computation systems. Furthermore, it also introduces the possibility of providing researchers with deeper insight into how neurons work, possibly introducing the ability to identify new brain diseases and find cures.
Moreover, the 'Brainoware' project opens up possibilities for creating AI systems that are not only more energy-efficient but also capable of learning and adapting in ways that mimic human cognition. This could revolutionise how AI is used in various fields, from healthcare to environmental monitoring, offering solutions that are both sustainable and highly effective.
What ethical considerations does such technology face?
There is no doubt that what the researchers have demonstrated is brilliant with regard to its scientific insight and advancement of technology, but there are some serious ethical considerations that arise from such developments.
By far, the biggest concern from such a technology is the use of living brain cells to power AI applications. Despite such a mass having minimal processing capability, it is hard to judge if that mass of neurons can register and feel pain, think, and, to some degree, be self-aware. If any of these concepts exist in that mass of neurons, then using them for data processing could result in an unbelievable amount of torture.
There is also the other ethical concern that forcing a living organism to process data could amount to slavery. Again, such an organism would need to be aware of its situation and feel pain, which requires complex neural pathways. As such, a mass of neurons will unlikely experience any distress, thereby making it perfectly ethical to use such biocomputers.
Overall, it is clear that using neurons to process data is far more efficient than a typical processing mechanism, but whether or not this is ethical remains unclear.
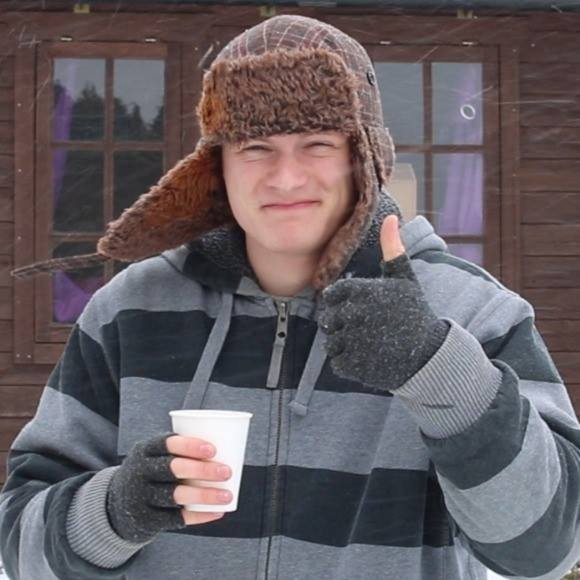