AI in Healthcare: How Deep Learning Detects CHD with Precision
11-03-2024 | By Liam Critchley
Things to Know:
- CHD is a leading cause of morbidity and mortality in infants, with early detection being crucial for effective treatment and increased survival rates.
- Traditional diagnostic methods are complex and inaccessible in many regions, highlighting the need for simpler, more affordable solutions like paediatric ECGs.
- Recent studies, such as the CHDdECG model, demonstrate the potential of deep learning to improve CHD detection, offering accuracy superior to that of experienced cardiologists.
- The integration of AI in paediatric healthcare promises to improve diagnostic processes, making early and accurate CHD detection more accessible worldwide.
Congenital heart disease (CHD) remains a significant challenge in paediatric healthcare, affecting millions of infants worldwide. Despite advancements in medical technology, early detection and diagnosis of CHD are fraught with complexities, often leading to delayed interventions and reduced chances of successful treatment. This article delves into the innovative use of paediatric electrocardiograms (ECGs) and the groundbreaking application of deep learning algorithms, such as the CHDdECG model, to revolutionise the early detection of CHD. By integrating cutting-edge technology with traditional diagnostic methods, we explore how these advancements offer new hope for timely and accurate CHD diagnosis, potentially saving countless young lives.
The Critical Importance of Early CHD Detection
Congenital heart disease (CHD) is one of the most common types of birth defects in infants and is one of the leading causes of children’s morbidity and mortality today. While CHD is a serious condition, the chances of survival can be increased if the disease is caught early, but that is unfortunately not often the case, with some low-income countries reporting that the delay diagnosis rate can be up to 85.1%.
If the disease is caught early on, there are a number of medical interventions that can be put into place, and it allows surgical procedures to be performed to try and treat the condition before it causes infant death. The longer it goes unchecked, the less likely that medical interventions are going to be possible to save a child with CHD.
Early and accurate identification of paediatric patients with CHD is however something that has been a challenge in health services around the world. This is because common examination methods—such as transthoracic echocardiography (TTE), X-ray, cardiac magnetic resonance imaging (MRI), and dual-source CT examinations—are complex, time consuming, expensive, and require the use of a skilled/experienced operator.
These issues with the current diagnostic techniques mean that diagnoses take longer than is ideal, and this can be for a number of reasons—e.g. the lack of a skilled operator in one hospital or procedures are too expensive for a country’s health system to perform regularly. Across many and low- and middle-income countries, the diagnosis is often delayed—even for critical cases—leading to sub-optimal clinical interventions. If CHD is to be caught early and treated early, then developing lower-cost and easier-to-use techniques is going to be key to saving more children’s lives. One of the options that has been touted recently is the use of non-invasive paediatric electrocardiograms (ECGs).
ECG as an Option
CHD is typically caused by structural abnormalities in the heart. This includes holes and leaky valves between the chambers. These abnormalities change the electrocardiovectors, which can manifest as abnormal signals in an ECG. Using these abnormal signals, ECGs can provide insights into a patient's cardio-electric activity. ECGs are also easy to use, used by many medical professionals, are of low cost, and are highly effective at detecting changes in electrical signals in the heart.
Recent advancements in deep learning have shown promising results in enhancing the accuracy of CHD detection through paediatric ECGs. A study highlighted in Nature Communications demonstrated a deep learning-based approach, known as CHDdECG, which integrates raw ECG-waveform data, human-concept features, and wavelet features. This integration allows for direct probabilistic predictions for CHD, showcasing superior performance over traditional methods and even experienced cardiologists. Such innovations could significantly lower the barriers to early and accurate CHD diagnosis, particularly in settings where access to advanced diagnostic tools is limited.
It’s thought that ECGs could be used to help detect if a patient has CHD; however, research into the area has primarily focused on correlating the signals with adult ECGs. Given that the signals for CHD measured in clinical setting are paediatric signals, research to date has not provided useful information for CHD interventions. There have not been many studies that use ECG signals from children, and this is where more research currently needs to be done to make ECG a more robust technique for the early detection of CHD.
The significance of leveraging paediatric ECG signals for early CHD detection cannot be overstated. The CHDdECG model's ability to accurately identify CHD from paediatric ECG data underscores the potential of deep learning in revolutionising CHD diagnostics. By focusing on paediatric signals, this approach addresses a critical gap in current research, offering hope for more effective early intervention strategies.
Turning to Deep Learning for Early Intervention
Obtaining more data from child ECGs is only one-half of the battle. The other side of the coin is that you then have to interpret that data accurately. Obtaining a lot of data to build models to show when a patient is likely to have CHD over being healthy can be a time-consuming process if done manually. This is where AI, especially deep learning algorithms, can come in.
Deep learning has been used through the medical and life science sectors to provide more robust analyses to different biological challenges—including detecting cancer cells from healthy cells using data based on the morphology of the healthy and cancerous cells. Deep learning has also been shown to be effective and reliable for ECG analysis, including having the ability to identify features that are often missed by human cardiology experts.
Studies have shown that deep learning models can automatically extract features from ECG waveform data and wavelet transformation, as well as conceptual features that are typically provided by human experts. As it stands, all these features have been possible with deep learning models, but there haven’t been many approaches that have analysed all of these features simultaneously in an end-to-end deep learning architecture to conduct automatic and efficient analyses. Additionally, while numerous studies have used deep learning models to analyse ECG data, not much attention has been directed towards developing specific models for detecting CHD—especially on large-scale paediatric datasets.
The development of the CHDdECG model represents a significant leap forward in paediatric healthcare. By analysing 65,869 paediatric ECG cases, the model not only demonstrates the feasibility of using deep learning for CHD detection but also highlights the importance of integrating diverse data types, including human-concept features and wavelet transformations. This approach ensures a comprehensive analysis, potentially setting a new standard for paediatric CHD diagnostics.
Advancing CHD Diagnosis with CHDdECG: A Deep Learning Approach
A recent study set out to fill this gap at the interface of ECG analysis and deep learning by creating an end-to-end deep neural network-based approach for paediatric ECG cases known as Congenital Heart Disease diagnosis via Electrocardiogram (CHDdECG). The CHDdECG integrated multiple features, including ECG waveform data, human-concept features, and wavelet features, to predict if a patient had CHD.
To visualise the innovative approach of the CHDdECG model, Figure 1 provides a detailed workflow of how AI-enabled CHD detection is achieved through the analysis of pediatric ECG data. This diagram showcases the integration of raw ECG-waveform data, human-concept features, and wavelet features, culminating in a model that offers a new level of accuracy and efficiency in diagnosing congenital heart diseases in young patients.
Custom-crafted features based on human concepts (a) were derived using specific algorithms (detailed formulas are available in the Supplementary Materials) from pediatric ECG waveform data (b). Simultaneously, characteristics of wavelet coefficient energy (referred to as wavelet features (c)) were extracted through wavelet transformation applied to the pediatric ECG waveform data. These three distinct feature sets were then input into the designated AI model (d) for seamless integration and the purpose of detecting CHD. The model's ability to identify CHD was depicted through the receiver operating characteristic curves and precision-recall curves (e and f) across a primary test set and two additional external test sets. Furthermore, the impact of CHD detection (using the net reclassification index, NRI) by the AI model, both independently and in conjunction with cardiologist consultation, was compared against the baseline performance of cardiologists without AI assistance (g), across ten randomly chosen test data groups from the Center-A test set. Detailed NRI analysis (NRI(+) and NRI(−)) can be found in the Supplementary Materials. All underlying data are accessible in the Source Data file. (Click to enlarge)
To obtain the data, potential paediatric patients underwent different examinations, but mainly transthoracic echocardiography and ECG. Additional tests were only required for certain cases and were at the discretion of the doctor. The doctors then manually analysed all the examinations to determine final outcomes as to whether they thought a potential patient had CHD. These diagnoses were compared against the results of the deep learning model.
The deep learning model used only the paediatric data to identify any potential CHD cases by integrating the features extracted from the ECG waveform wavelet data with human concept features. 65,869 ECG cases from children aged 2.12 ± 1.50 years were used to develop the model so that it could distinguish between healthy children and those with CHD.
As for the test itself, a real-world test set of 12,000 cases was evaluated alongside two test data sets composed of 7137 and 8121 cases. The comparison between the two approaches in the study showed that the deep learning model outperformed the ECG cardiology experts and was more accurate at predicting who had CHD/structural heart defects and who didn’t.
Conclusion: The Future of CHD Detection with Deep Learning
Deep learning models have been used in recent years to improve clinical outcomes and take the workload of medical professionals by providing an accurate and automatic analysis of large biological datasets. For detecting patients with CHD, it seems like the deep learning models trialled also fall into similar situations where they are more useful at predicting clinical outcomes than humans who do it manually. The study also suggested that the model may have some knowledge beyond human cognition to detect who has CHD and who doesn’t. Similar models have been successful in providing clinical outcomes, and this new study demonstrates the potential for using easy and cheap ECG tests with deep learning to better diagnose CHD in children.
The implications of the CHDdECG study extend beyond the immediate benefits of improved CHD detection. By outperforming cardiologists in identifying CHD from paediatric ECG data, the model illustrates the untapped potential of AI in healthcare. This breakthrough underscores the importance of continuing to explore the integration of AI and deep learning in medical diagnostics, potentially transforming the landscape of paediatric cardiology and improving outcomes for children worldwide.
Reference:
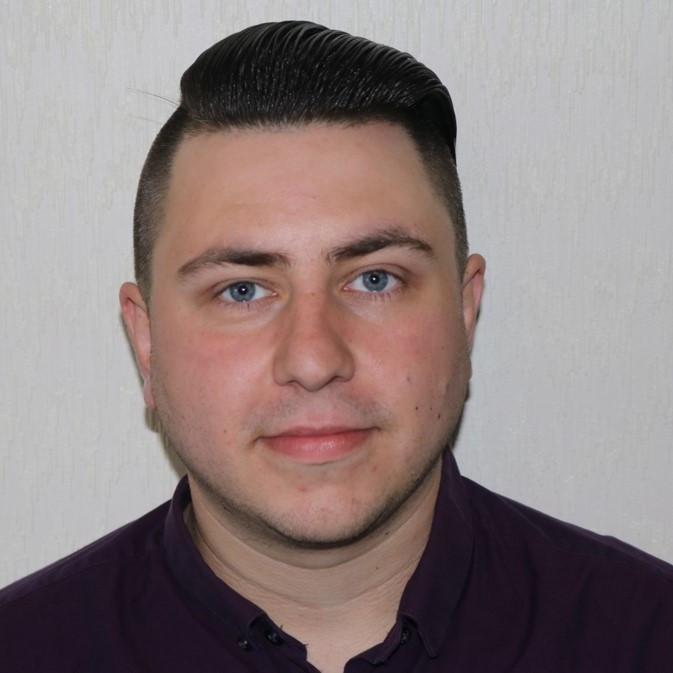