2D Transistors Mimic Locust Brains for Collision Avoidance
30-05-2024 | By Robin Mitchell
Researchers from the Indian Institute of Technology Bombay and King's College London have developed an ultra-low power 2D transistor that mimics the collision-avoidance neurons of locusts, aiming to enhance autonomous robots and vehicles. It is hoped that this breakthrough could significantly reduce the energy costs of AI technologies, thus enabling AI to be integrated into low-energy applications. What challenges does AI face in embedded designs, how might these super-efficient 2D transistors impact the development and deployment of autonomous technologies, and how could the application of this technology extend beyond obstacle avoidance to other AI functionalities?
- Efficient energy consumption remains a key challenge in AI-embedded designs, necessitating innovative solutions inspired by biological systems to optimise performance and sustainability.
- Energy-efficient 2D transistors mimic locust neurons for improved collision avoidance in AI technologies, enhancing autonomy and sustainability.
- Innovative 2D transistors inspired by biological systems are transforming AI technology, paving the way for energy-efficient and intelligent systems that mimic human brain functions.
Challenges of Implementing Artificial Intelligence in Embedded Systems
The field of AI has seen notable advancements in recent years, with applications ranging from virtual assistants to autonomous vehicles. Furthermore, advances in LLMs (such as ChatGPT) have enabled the creation of all kinds of content, including emails, stories, artwork, and even music. However, as AI technologies become more prevalent in embedded designs, a new set of challenges has emerged, threatening to slow progress in this area.
One of the most pressing issues facing AI-embedded designs is the high energy consumption associated with these systems. The demand for processing power in AI applications often leads to increased energy usage, which can drain batteries quickly and limit the overall efficiency of the device. For devices connected to mains power, this high energy usage is quickly reflected in high electric bills (in my case, a room heated to 55 degrees Celsius and a very painful bill).
Additionally, the limited battery life of embedded AI devices poses a significant hurdle in their widespread adoption. Users expect seamless functionality from their devices without the constant need for recharging, making it crucial for AI designs to find a balance between performance and energy efficiency. In such applications, heat dissipation further adds to these challenges, as the continuous operation of AI systems can generate significant amounts of heat, potentially leading to overheating and performance degradation.
Furthermore, the size constraints inherent in embedded designs present a unique set of obstacles for AI technologies. The need to fit complex AI algorithms and processing units into compact devices calls for creative solutions to optimise space utilisation without compromising performance. While existing solutions have attempted to address these challenges by prioritising energy efficiency over raw processing power, this approach often results in a trade-off that limits the full potential of AI applications.
The Impact of Super-Efficient 2D Transistors on Autonomous Technologies
A recently collaboration between the Indian Institute of Technology Bombay and King's College London has led to a significant development in the field of AI technology. Researchers from these distinguished institutions have successfully developed ultra-low power 2D transistors that mimic the collision-avoidance neurons of locusts, with the goal of improving the capabilities of autonomous vehicles and robots.
Moreover, the application of these 2D transistors can significantly enhance the performance of embedded systems in autonomous technologies. By integrating these transistors into existing frameworks, engineers can develop more responsive and efficient AI-driven solutions, thus reducing the overall energy footprint of such systems.
This achievement is notable for its potential to enhance the energy efficiency of AI technologies, especially concerning autonomous systems. These 2D transistors provide a novel solution for reducing power consumption in autonomous technologies, making them more sustainable and efficient for practical applications.
Advancing Sustainable AI Technologies with 2D Transistors
Researchers highlighted that this breakthrough aligns with the increasing demand for sustainable AI technologies, particularly in the automotive and robotics sectors. This development not only offers a practical approach to energy efficiency but also supports the broader goal of achieving sustainability in technology.
The research centred on collision avoidance, a key aspect of autonomous navigation necessary for the safety and reliability of these technologies. By replicating the LGMD (lobula giant movement detector) neuron found in locusts, which activates in response to nearby large objects, the scientists developed a transistor that mimics this functionality with significant energy savings. The transistor uses less than 100 picojoules of energy, demonstrating its potential for minimal power consumption.
The innovative design of these transistors leverages the unique properties of two-dimensional materials, which allow for scalable and tunable functionalities. This adaptability makes it feasible to implement these transistors in a variety of AI applications, from simple sensors to complex autonomous navigation systems.
Versatility and Scalability of 2D Transistors in AI Applications
This new 2D transistor is not only cost-effective but also versatile, capable of being reprogrammed to detect various types of movement and effectively avoid obstacles with high precision. Although the current transistor design is simple, focusing on activation when movement is detected within a certain range, the researchers anticipate that this technology could be expanded and incorporated into more complex AI systems in the future.
Looking ahead, the integration of these 2D transistors into broader AI frameworks could revolutionise the way autonomous systems operate. By providing a reliable and energy-efficient solution, this technology has the potential to enhance the capabilities of smart devices and systems, making them more effective and environmentally friendly.
Future Prospects of 2D Transistors in Autonomous Systems
Further advancements in this technology could lead to the development of multi-functional AI systems capable of real-time decision-making and adaptive learning. Such systems would benefit from the low-power consumption and high efficiency provided by these 2D transistors, enabling more intelligent and autonomous operations in a wide range of environments.
The possible uses for these highly efficient transistors go beyond collision avoidance in robots and autonomous vehicles. Professor Bipin Rajendran from King's College London mentioned that the spiking neuron circuit developed in this study could be applied in other neuromorphic applications that require low-energy solutions. This suggests a wide range of potential for integrating this technology into various analog or mixed signal systems that mimic human brain functions, offering a versatile approach to energy-efficient AI operations.
This study underscores the importance of interdisciplinary collaboration in advancing AI technologies. By combining expertise from neuromorphic engineering and two-dimensional materials science, the researchers have paved the way for innovative solutions that address both performance and sustainability challenges in AI.
Exploring the Versatility of Technology in AI Applications
The introduction of 2D transistors inspired by locust neurons marks a notable advancement in electronics engineering, setting the stage for the development of neuromorphic systems that mimic human brain functions. These transistors have the capability to significantly change analog and mixed signal technologies by offering low-energy spiking neurons that can power various AI functionalities.
Beyond their initial use in obstacle avoidance, these 2D transistors are expected to be applied in a wide array of AI applications such as pattern recognition, decision-making, and adaptive learning. By utilising energy-efficient mechanisms inspired by biological systems, engineers can tap into the vast capabilities of these transistors in creating intelligent systems that closely resemble human cognitive processes.
The use of these highly efficient 2D transistors in electronics engineering could lead to the creation of energy-efficient AI systems across numerous industries. By reducing energy consumption and improving processing capabilities, these transistors have the ability to alter the landscape of AI technology, making it more sustainable and effective for practical applications.
Imagine a future where autonomous vehicles not only navigate obstacles seamlessly but also make split-second decisions based on complex patterns and data analysis, all powered by these transistors. The potential for these transistors to enhance AI functionalities is significant, opening up new possibilities for engineers to develop and innovate.
Additionally, the flexibility of these transistors allows for their use in various applications beyond obstacle avoidance. From healthcare diagnostics to financial forecasting, the adaptability of these transistors enables them to improve AI functionalities across different sectors, changing the way we interact with technology.
As the researchers continue to utilise the capabilities of these 2D transistors, the opportunities for creating intelligent systems that can learn, adapt, and make decisions autonomously are vast. The future of energy-efficient AI systems powered by bio-inspired technologies is on the horizon, offering a glimpse into a future where intelligent machines work seamlessly alongside humans to foster innovation and progress.
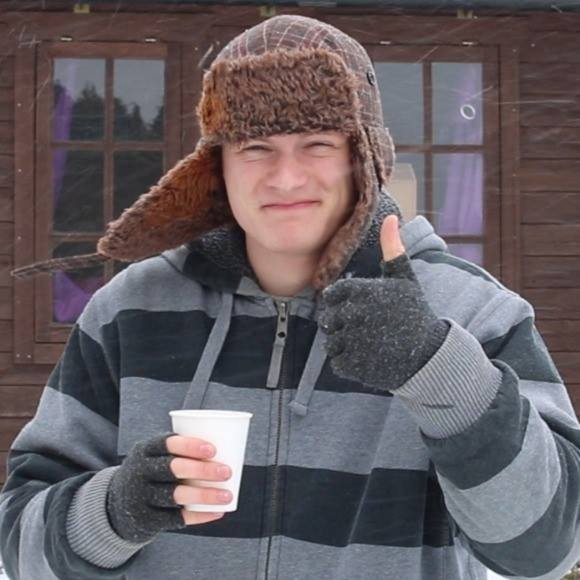