Cervical Cytology Screening: AI Boosts Accuracy and Sensitivity
23-07-2024 | By Liam Critchley
Cervical cancer is a global health issue for women, and this is why many countries (such as the UK) recommend that many women take a cervical smear test at regular time periods throughout their lives. There’s a good reason for this, and it’s because cervical cancer is one of the most common cancers globally and is the 4th leading cause of cancer-related deaths around the world.
Like many cancers, early screening is vital for catching tumours early on—and this is why there has been an insistence on regular testing for many years now. If cervical cancer is caught early, then the chance of surviving is much higher than if caught at later stages.
Key Things to Know:
- Cervical cancer is the fourth leading cause of cancer-related deaths among women globally.
- Early screening through methods like cervical cytology is crucial for improving survival rates.
- AI-based systems, such as the AICCS, enhance the accuracy and efficiency of cervical cancer screening.
- The AICCS system shows significant improvements in sensitivity and specificity compared to traditional diagnostic methods.
Challenges in Cervical Cancer Screening
While public campaigns, pressure from medical professionals, and regular reminders have led to more people being tested, there are still challenges with the analysis of the data that is collected. Current screening methods include cervical cytology, HPV testing, and DNA ploidy testing—with cervical cytology screening being the method that is used to screen large populations because it is cheap and cost-effective.
Screening for cervical cancer through cervical cytology has significantly contributed to early detection and reduced mortality rates. However, the effectiveness of this screening method is hindered by the availability of skilled cytopathologists, as highlighted in a recent study. The study notes that the shortage of cytopathologists in regions like China has led to substantial false negative rates in cervical cancer diagnoses, underscoring the need for improved diagnostic tools.
While there is a large uptake of people who take these tests in some countries (but it should really be as many as possible), analysing the data to detect cancer still poses a challenge. This is because there is a lack of cytopathologists around the world who can perform the analysis. Current estimates show that the lack of trained clinicians has led to over a 10% false negative rate during routine cervical cancer screening.
Artificial intelligence (AI) algorithms—such as machine learning, deep learning, neural networks and object detection models—are helping to expand how AI is being implemented in the healthcare sector. AI has already been used to improve the screening and diagnoses of other cancers, as well as other medical disease/ailments. There’s an interest now in using AI to improve the outcomes of cervical cancer testing due to the lack of clinicians available.
How AI Offers a More Effective Cervical Cancer Diagnosis
AI offers a lot of advantages for cervical cancer screening, including improving the consistency of results, improving the alignment with biopsy outcomes, improving the sensitivity of screening methods and reducing the chance of false negatives and misdiagnoses occurring—which, in turn, could help to save more lives.
According to the study, AI systems like the AICCS can standardise the diagnostic process, reducing the variability inherent in human analysis. This standardisation is crucial for maintaining high diagnostic accuracy across different healthcare settings, especially in under-resourced areas. The implementation of AI can also facilitate continuous monitoring and quality control, ensuring that screening programmes remain effective over time.
There have been other attempts to use AI for diagnosing cervical cancer and enhancing screening efforts, but a lot of models either cause the screening methods to become more complex or the algorithms have only focused on specific cell types so far, limiting their use in real-world healthcare scenarios.
The AICCS System
Researchers from China have looked at creating a new AI model for diagnosing cervical cancer. The system, known as an artificial intelligence cervical cancer screening (AICCS) system, was designed to diagnose cervical cytology grades (the ratio of healthy cells to abnormal cells that are graded by how many abnormal cells are present) and cervical cancer. China is a good place to perform the study because it suffers from a lack of trained clinicians who can diagnose cervical cancer. In 2022, China only had 20,400 registered pathologists when the actual demand was around 100,000.
The AICCS developed aimed to detect abnormal cells and cancer by analysing whole slide images (WSIs) of cervical cells. The systems were validated using multicentre, retrospective, and prospective population-based datasets, as well as randomised observational trial data. In total, the AICCS system was trained using a total of 16,056 participants and included data from two different hospitals.
The AICCS system utilises advanced AI algorithms to analyse whole slide images (WSIs) of cervical cells. Figure 1 provides an overview of this process, illustrating the workflow from image acquisition to the classification of cytology grades.
Figure 1: The digitization and preprocessing of WSIs begin with the digital conversion of cervical liquid-based preparation samples collected and preserved by the sedimentation liquid-based preparation method (A). WSIs that pass quality control undergo patch-level detection, which involves dividing a WSI into smaller patches using a sliding window approach and annotating abnormal cells based on the criteria defined by TBS 2014 (B). The output of the cell detection model serves as input for the WSI-level classification model. The WSI-level classification model utilises the results from the patch-level cell detection model to generate possible cytology grades according to TBS 2014: ASC-US, LSIL, ASC-H, HSIL, SCC, and AGC (C). The content of microscope readings in Section C classification at the WSI level originates from BioRender. The ‘Confirmation of Publication and Licensing Rights’ is provided. (Click to Enlarge)
The validation process for the AICCS system involved rigorous testing across diverse datasets to ensure its robustness and accuracy. The system's performance was benchmarked against traditional diagnostic methods, demonstrating superior sensitivity and specificity. This validation underscores the potential of AI to revolutionise cervical cancer screening by providing reliable and scalable diagnostic tools that can be deployed globally.
AI Models Used in AICCS
The AICCS system used two different AI models. One was used to detect the cells, and the second was used to classify the WSI. The process involved acquiring and processing WSIs of digitised images of liquid-based samples that were collected. The WSIs that passed quality control underwent patch-level detection where a WSI was divided into smaller patches and abnormal cells were annotated. The patch-level cell detection model was then used to determine the possible cytology grades. There are three cytology grades:
- Grade 1 (low grade): up to 1/3 of the lining thickness of the cervix has abnormal cells.
- Grade 2 (high grade): up to 2/3 of the lining thickness of the cervix has abnormal cells.
- Grade 3 (high grade): The full lining thickness is fully covered with abnormal cells.
Accuracy and Benefits of the AICCS System
As well as identifying the different cells, the AICCS was able to accurately predict the cytology grades across different datasets with a high degree of accuracy, sensitivity, and specificity, including in the randomised observational trial. The randomised observational trial itself also showed that the AI model was more accurate than using cytopathologists alone, with a 13.3% improvement in sensitivity over the cytopathologists.
In terms of specific statistical data, the area under curve (AUC) was taken to measure how well the AI performed. This is a standard approach to validate the accuracy, sensitivity and specificity of different diagnostic tests, where the ideal sensitivity is 1, and the false positive rate equals a 0 on the statistical scale (similar premise to an R-value that’s used to measure data correlation). The AI model achieved an AUC of 0.947, a sensitivity of 0.946, a specificity of 0.890, and an accuracy of 0.892.
To highlight the effectiveness of the AICCS system, Figure 2 presents its performance metrics, including accuracy, sensitivity, and specificity, compared to traditional diagnostic methods. This data underscores the system's superior diagnostic capabilities.
Figure 2: RetinaNet, a one-stage object detection method, was adopted as the anomaly cell detection model at the patch level, designed to differentiate between six classes of abnormal cells: ASC-US, LSIL, ASC-H, HSIL, SCC, and AGC (A). The creation of a WSI classification model involves generating features that encapsulate the statistical data derived from the patch-level detection model, which are then used to train the WSI classification model through the application of a random forest algorithm (B). (Click to enlarge)
Moreover, the AI system demonstrated high negative predictive values (NPV), crucial for ensuring that non-cancerous cases are not falsely identified as positive. This high NPV reduces unnecessary follow-ups and treatments, thereby alleviating the burden on healthcare systems. Additionally, the use of AI can streamline the diagnostic workflow, enabling faster decision-making and improving patient care outcomes.
The AICCS system was able to accurately identify different cytology grades to determine the level of abnormal cells in a person to see if they have cancer (or are likely to have it) and could do it with a higher sensitivity than trained clinicians alone. The ability of the model to differentiate healthy cells from abnormal cells could help not only lead to earlier detection and more accurate screening results but also help to take the pressure off clinicians who are stretched in terms of the number of available specialists. Like many areas of AI in the healthcare sector, the AI model has become a useful tool for clinicians to help improve patient outcomes and reduce the burden on healthcare systems.
Conclusion
The integration of AI in cervical cancer screening, as demonstrated by the AICCS system, offers significant improvements in accuracy, sensitivity, and efficiency. This advancement addresses the shortage of cytopathologists and enhances early detection, ultimately improving patient outcomes and reducing the burden on healthcare systems. The success of AI models like AICCS paves the way for broader applications of AI in medical diagnostics, promising a future of more reliable and accessible healthcare.
Reference:
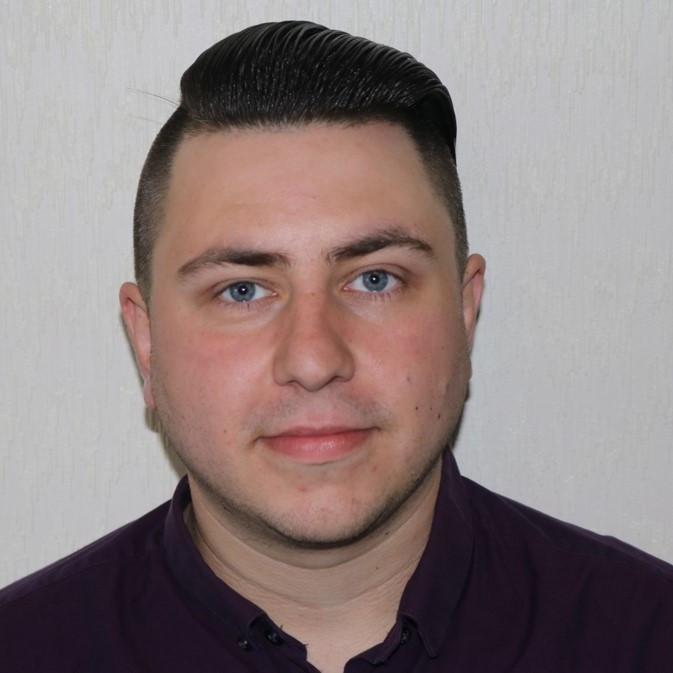