Will Doctors Ever Fully Trust Artificial Intelligence in Medicine?
15-08-2024 | By Paul Whytock
Key Things to Know:
- AI's Growing Role in Medicine: AI is increasingly being used in various medical applications, from diagnosis to long-term care planning.
- Doctors' Concerns: Despite AI's potential, doctors are cautious due to concerns about diagnostic errors, transparency issues (Black Box Syndrome), and the erosion of patient trust.
- Patient Trust Issues: Studies show that patients are often reluctant to trust AI, preferring human doctors for diagnosis and treatment.
- Ethical and Legal Considerations: Transparency is essential in AI's application in medicine, with doctors emphasising the need for informed consent and openness about AI's use.
Introduction
Artificial intelligence (AI) is making deep inroads in the field of medicine, and recent developments have demonstrated success in a range of clinical applications. But it's not all good news.
I recently had the opportunity to discuss the implications of medicinal AI with two young doctors at Kings College Hospital, London.
Interesting because this is the generation that will inevitably have to work with and manage AI in their everyday careers.
In summary, it's fair to say they were open-minded about the possibilities that AI had in medical applications such as diagnosis, drug and therapy developments, analysing digital images, patient monitoring, blood and sample analysis and planning long-term care.
But, they also had some notable concerns about its unchecked proliferation.
In a nutshell, their worries consisted of diagnostic errors, system errors that were not transparent (known as Black Box concerns where the application of AI is a closed automated system), erosion of the doctor/patient relationship trust, database errors or inadequacies when creating relevant algorithms, inadequate maintenance and system validation, and patient rejection of new ideas.
We'll take a look at some of those in more detail.
But before that, I put it to the doctors that when it came to the very important trust element between the doctor and patient, it would be entirely necessary to tell patients that the diagnosis you were presenting to them had been generated electronically. Particularly if the doctors had checked the results. Their answers were immediate and plainly stated.
Morally Wrong
In their views, critical ethical and legal considerations make it essential to be open about the use of AI, and morally, the doctors felt secrecy was wrong. Add to that the fact that patients have the absolute right to informed consent with regard to their own healthcare, and these doctors were spot on with their thinking.
The National Institutes of Health (NIH), part of the American Department of Health and Human Services and the nation's medical research agency, has an important analysis on the trust element.
In its opinion, AI has the potential to improve diagnostic accuracy. Yet, the study found that patients are often reluctant to trust automated systems.
Research shows patients prefer human doctors to AI-powered machines in diagnosis, screening and treatment. In some studies, patients were happier to follow medical advice from a doctor rather than a computer and were less trustful of computers as providers of medical advice. Add to that some patients are less trusting of the doctors themselves, that rely on digital decision-making.
More recently, in a series of studies, patients were less willing to schedule an appointment to be diagnosed by a robot, and they were willing to pay considerable money for a human provider.
Regarding AI systems, the NIH analysis also found that people believe AI is unable to account for patients' unique characteristics and personalities.
This is particularly important not only in initial diagnosis but in the care of long-term patients, how they face the illness, and the treatments that are applied.
So what about the doctor's other concerns? Recent studies have shown 53% of medical professionals believe AI isn't ready for medical use yet.
Meanwhile, 41% said they were both excited but cautious about the potential uses of AI.
The president of the American Medical Association (AMA), Jesse M. Ehrenfeld, MD, MPH, has been quoted as saying: "Whatever the future of health care, it is essential patients know there is a human being on the other end helping guide their course of care."
Transparency is Key
The AMA is influencing the development of AI health care by creating standards and a common language and has released a set of AI Principles (PDF).
Anaesthesiologist Doctor Ehrenfeld, co-chair of the Association for the Advancement of Medical Instrumentation's AI committee, has said the purpose of these principles is to provide continued guidance to physicians and developers on how to best engage with and design new AI-enabled technologies with the understanding that policy development related to AI will likely continue to develop given the rapid pace of change.
In Doctor Ehrenfeld's view, AI must be designed, developed, and deployed in an ethical, equitable, responsible, and transparent manner.
The AMA has also made clear that transparency is a key element, especially when insurers use AI or other algorithmic-based systems to make medical claim determinations or set coverage limits.
Trust and Black Box Syndrome
Trust is the keystone of the doctor/patient relationship. That trust is severely eroded if there are concerns over any AI system black box issues that throw the slightest suspicion of results.
According to The Lancet, an esteemed, peer-reviewed general medical journal in London, concerns over AI's unknown and unknowable black boxes have spurred a drive toward ways of building trust in AI.
However, The Lancet believes that no matter how well-intentioned, applying trust to AI is an error, mistakenly assuming that AI belongs to a category of things that can be trusted. Trust implies entrustment, placing something of value in the responsibility of another in circumstances of vulnerability.
The ideal patient-physician relationship reflects this definition. Patients place their health in physicians' voluntary responsibility and discretion and believe in physicians' benevolent motives. AI does not have voluntary agency and cannot be said to have motives or character.
This is a strongly worded condemnation, but exactly what are some of the trust issues? And what are the things where AI can fail?
Medical Data Inadequacies
Like all systems, AI requires testing and regular validation. Here lies a problem. Clinicians require high-quality data to achieve full system reliability and confidence in the AI analysis.
Because medical data can vary enormously between different health organisations, countries, ethnic groups, and software systems applied to that data, gathering patient information and images of quality and relevance to test AI algorithms becomes very difficult. This can result in lower levels of system accuracy.
According to the NIH, the safe translation of AI research into clinically validated and appropriately regulated systems that can benefit everyone is challenging. Robust clinical evaluation, using metrics that are intuitive to clinicians and ideally go beyond measures of technical accuracy to include quality of care and patient outcomes, is essential. More work is needed to identify themes of algorithmic bias and unfairness while developing mitigations to address these.
Research Flaws
According to the Emeritus Institute of Management, there are not enough established methodologies, research, or peer-reviewed studies of AI in healthcare. The majority of studies have been retrospective and based on historical patient medical records.
However, to realise the true value of AI diagnosis in real-world settings, physicians must study current patients over time, which means prospective research. To achieve reliable prospective research, doctors should monitor the health of their patients by combining physical examinations with telehealth visits and remote monitoring technologies.
Who's in Charge?
Currently, a substantial majority of medical opinion says AI cannot be left in charge.
Analysis comparing the performance of algorithm-based AI systems to human clinicians found that AI performance can already match the accuracy of human medics regarding certain diagnostic tasks. If AI succeeds in surpassing the performance of the human will this be detrimental to the authority of doctors?
There are contrasting views on this. Some predict that AI will eventually push doctors to one side by outperforming them in some critical clinical applications.
Others think AI will increase and improve upon the capabilities of human clinicians without replacing them. Part of the thinking on this is that AI systems lack the emotional intelligence and empathy that are key in the delivery of healthcare.
So human involvement remains essential, particularly because neural-network AI systems gain knowledge from correlations alone, which means they are restricted in how well they can detect underlying illnesses.
Analysing the Images
It's now well-recognised that analysing digital medical images is one of the true strengths of AI. Medical imaging is one of the most promising areas for the application and innovative use of AI. The use of AI in radiology has the potential to improve the efficiency and efficacy of medical imaging. Its use may also alleviate some of the burden and burnout experienced by radiologists who feel overwhelmed by the proliferation in the volume of imaging studies performed and are unable to devote sufficient time to providing meaningful, patient-centric care.
Developing the use of AI for imaging analysis has been an area of rapid change. AI algorithms look at images to identify patterns and then use pattern recognition to identify abnormalities.
AI doesn't tire like we humans do and is ideally suited to the repetitious task of analysing large numbers of images for each study from MRIs. Also, algorithm development advances will increase AI's role in examining pathology and radiology images.
Finally, while AI is intended to reduce diagnostic errors, there is the risk that the use of AI can introduce new potential errors. New potential errors have been detailed in the analysis by renowned AI expert Robert Challen.
In his view, there is potential for error resulting from discrepancies between the data used to train AI systems and the real-world clinical scenario due to the limited availability of high-quality training data. AI systems are not as equipped as humans to recognise when there is a relevant change in context or data that can impact the validity of learned predictive assumptions.
Therefore, AI systems may unknowingly apply a programmed methodology for assessment inappropriately, resulting in error.
The AI Risk elements
Firstly, the question of quality data. Clinicians require high-quality datasets for the clinical and technical validation of AI models. However, due to the fragmentation of medical data across several EHRs and software platforms, collecting patient information and images to test AI algorithms becomes challenging. Another obstacle is that the medical data from one organisation may not be compatible with other platforms due to interoperability problems...
Performance Metrics
The measures used to gauge an AI model's success are not necessarily transferable to clinical settings. The discrepancy between the clinical efficacy demonstrated in the real world and the technical precision of AI tests is referred to as the AI chasm. To avoid this gap, developers and clinicians should collaborate to investigate how AI algorithms enhance patient care. To do this, they can assess AI models for accuracy using decision curve analysis. This method enables them to evaluate the clinical usefulness of a prediction model by comparing the datasets and estimating the chances of an AI model's success in the real world.
Research Flaws
Insufficient methodologies of AI in healthcare create research errors. The majority of studies have been retrospective and based on historical patient medical records. However, to realise the true value of AI diagnosis in real-world settings, physicians must study current patients over time, which means prospective research.
The End Game
Despite all the developmental and application challenges, AI will continue to find ever-increasing use in medicine. And with careful regulated development it will be of benefit too medical professionals and their patients.
As always, there is, of course, a lot of money being poured into AI. Currently, the global market for medicine-related AI systems stands not far off €185 billion. So, there is no lack of incentive there.00:0008:41
References
- National Institutes of Health (NIH). https://www.nih.gov
- American Medical Association (AMA). https://www.ama-assn.org
- Association for the Advancement of Medical Instrumentation. https://www.aami.org
- The Lancet. https://www.thelancet.com
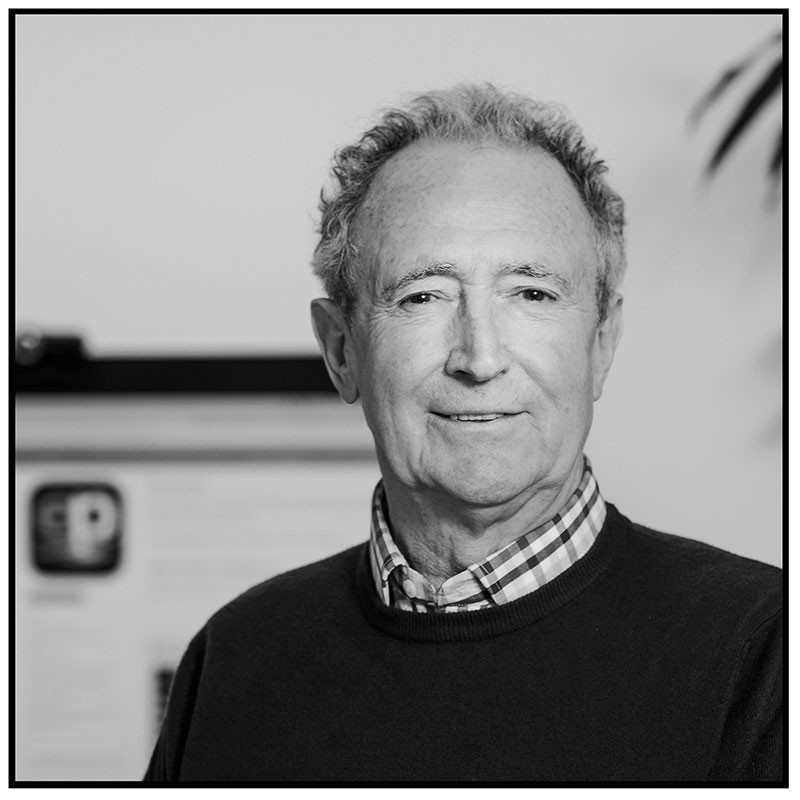