Predictive Maintenance: Outperform Technicians with IoT
03-09-2024 | By Gary Elinoff
IoT-Based Predictive Maintenance Architecture. Image source: ScienceSoft
Key Takeaways about Predictive Maintenance: Better than an Army of Technicians
- Predictive maintenance involves the use of a wide variety of sensors to capture key parameters, including heat, pressure and humidity measured over the totality of a system being monitored and controlled.
- These parameters are analyzed on a moment by moment basis by data engines, often including AI, that are generally accessible over the internet.
- The public is most aware of IIoT based predictive maintenance applied to factory systems. As we shall see, predictive maintenance can be applied to a much broader range of use cases.
Introduction
The Industrial Internet of Things (IIoT) is a network of industrial devices linked together over the same internet through which you are reading this article. Just as people use the internet to exchange ideas, the IIoT allows information regarding the health and function of those industrial devices to be remotely monitored and controlled from anywhere.
In a previous Electropages article entitled IIoT Basics and Key Benefits of Industrial Internet of Things, we covered IIoT in broad terms. In this article, we narrow our focus on how the IIoT can be used to achieve what we define as predictive maintenance.
Predictive Maintenance vs Preventive Maintenance
Preventive Maintenance is as old as the machines themselves. A factory worker will inspect a valve to see if it’s leaking. A technician checks to see if a part is overheating, which is always a sure sign of trouble. An electrician drags out a voltmeter, climbs a ladder, and checks the voltage and current going into or out of a remote system to see if it’s within spec. There are four obvious problems here.
- Preventive maintenance takes time and effort.
- Problems may occur between preventive maintenance checks.
- There may be no problem, and the effort yields no useful information.
- Some preventive maintenance tasks may be physically dangerous to people; our electrician might well fall off the ladder.
Predictive Maintenance is based on arrays of sensors attached to the components that are important parts of the overall system being monitored and controlled. As described by Intuz[1], examples of commonly used sensors include, Pressure, Gas, Temperature, Humidity, Vibration and Proximity Sensors.
Commonly employed IIoT Sensors. Image source: Intuz
Usually, the next step is for the readings for each sensor to be digitized and sent up to a monitoring point which can be located anywhere in the world, where the data is stored, and here’s where the fun starts.
Eventually, the device will fail. Analysts will look at the historical stored data, and compare it to the values observed on and about that failure point. After the device that was monitored is replaced, computer-based analytics will continuously monitor the sensor’s output, transmitted over the IIoT. When the sensor starts reporting values that correspond to failure, then and only then is a technician dispatched to replace it.
There are three major changes now made possible in how maintenance is conducted:
- Technicians do not have to conduct “witch hunts”, interrogating and inspecting devices that are not in danger of failing.
- The device is replaced before it fails, not after.
- Production is not halted due to unexpected component failures.
And it doesn’t end there.
Better than an Army of Technicians
Because now, engineers and management will have a minute by minute, blow by blow picture of what is happening inside the system being monitored and controlled. The system is literally under the microscope at all times, when it’s working and when it’s in trouble, and a minute by minute record of what every monitored point experiences is available in memory for analysis.
For example, what else was happening when component “A” failed? For example, what was happening in components “B” and “C” or any other monitored point? Or, as described by TIM (The Thread In Motion)[2], “This real-time data is transmitted to centralized systems, where advanced analytics and machine learning algorithms process the information. By continuously monitoring equipment conditions, these algorithms can detect subtle deviations from normal operation, indicative of potential issues.”
Predictive maintenance, driven by IoT technology, allows manufacturers to address issues precisely when they arise, ensuring smoother operations and cost savings.
To duplicate this with human operators, one operator would need to be placed at each operating point. The operator would have to complete the inhuman feat of making many measurements per second, perfectly coordinated in time with every other operator.
The possibilities are endless, limited only by the power of the analytical engines that are employed. The power of analytics is enhanced through the use of artificial intelligence (AI). As an example, AI can be used to help determine if an image caught by a camera corresponds to a situation that
portends trouble.
Edge Computing
We spoke about Edge Computing in a previous Electropages article entitled, What is Edge Computing? The Future of Real-Time Data Processing. Edge computing involves doing some, or even most computational analysis right at the source, where the IIoT interfaces with the system being monitored and controlled. This offers a number of critical advantages.
- Latency. Decisions can be made, and changes can be made right on the spot. There is no need to transmit all of the data over the internet to a remote server, which may be experiencing its own time-based issues, and then transmit the results back to the monitored system for an actuator to effect necessary changes. For example, if a valve fails, the system may need to be shut down immediately. Waiting for a round trip to a remote analytical engine may be too long a wait to avert disaster.
- Security. We discuss internet security in a recent Electropages article Industrial IoT Security: Top Priority for Industry 4.0 Systems. Anything being transmitted over the internet is in danger of being hacked. If decisions are made locally, at the edge, the data never leaves the system being monitored. Rivals and opponents never get a chance to access your system’s strengths – or its weaknesses.
As it stands now, estimates for the amount of energy used worldwide by internet servers range from 2% to 4% of the world’s total electrical power. AI computations are notoriously power-hungry, and the AI boom is just beginning.
Worse still, AI computations usually require multiple references to vast amounts of data held in server memory. Physical servers are often located where electricity is cheap and, to save on cooling costs, where the climate is cold. If multiple sensors located on devices to be monitored and controlled must make frequent references to servers located thousands of miles away, the operation of the internet as a whole will be seriously challenged.
With edge computing, references are only to small datasets located on the spot. Decisions are faster and safer, less electricity is used, and the need for critical internet bandwidth is greatly constrained.
Digital Twins
As described by Onlogic[3], “A Digital Twin is a virtual version of a physical object, process, or location that serves as a real-time digital counterpart.” Digital Twins are built by gathering all the available information and then recreating it in a digital space. “Every individual component, the ways those components interact and even the environment they exist in are digitally replicated.” Furthermore, “The digital twin then uses artificial intelligence to simulate and demonstrate the effects that changes in design, process or conditions would have, without having to subject the real-world object to those same changes.” This process can be defined as Predictive Analytics.
Onlogic cites some examples:
- Analysts can simulate the effect harsh conditions might have on a jet engine. This can be done, for example, simply by increasing the temperature on the digital twin and observing the result on the simulator without endangering the physical jet engine.
- In trying to optimize the traffic pattern around a new stadium, analysts can change traffic light patterns and one way street directions on the digital twin without making any changes (yet!) in the real world.
The sensors we described earlier are continuously monitored, and “with that constant flow of data, changes made in the real world are reflected in the digital twin, allowing it to evolve as the project does.”
Real World Examples
As mentioned at the very beginning of this note, the applications of predictive maintenance go well beyond the factory floor, even though the manufacturing application is the most well-known. That’s why, throughout this article, I’ve chosen to use terms like system being monitored and controlled to avoid suggesting exclusivity. Here are a few examples of non-manufacturing use cases.
- Renewable Energy. As noted in Forbes[4], IIot based predictive maintenance can be used to predict failures in wind turbines before they happen. The health of solar panels can be tracked, with AI being of particular relevance here. Hydropower plants can be monitored and controlled in a similar manner as factories, and battery energy storage systems (BESS), a frequent cause of problems, can be monitored and corrected as needed.
- Transportation and Logistics. Webby Lab[5] describes how “IoT sensors attached to trucks, containers, ships, and vehicles monitor cargo status, temperature, humidity, and location in real-time. Predictive maintenance, in this case, allows businesses to optimize routes, prevent freight damage, and speed up delivery times.”
- Jet Engines. Rolls Royce[6] has been installing a wide variety of sensors into its engines, actively monitoring them as they operate in aircraft. “The data these devices generate is aggregated and then analyzed in the cloud. Already, this information helps make predictions about maintenance and service needs of a particular engine based on the performance of many other engines of the same model.” The company gains even more value as they analyze them as a group, looking for commonalities, with AI aiding the efforts.
Challenges and Opportunities
The numbers of working age people the world over is in steep decline, but the need for manufactured goods is steadily rising. In the United States, the problem is especially acute due to the stated goal of returning more production to native shores. The challenge is not only to do more, but to do it with fewer employees.
IIoT preventive maintenance will mean less downtime. It will also mean less human time spent on maintenance, and with a complete digital map of facilities available to engineers and planners, more and more of the operations can be controlled automatically by decision engines, with correspondingly less need for humans on the front line.
There may well turn out to be another unexpected result – greater sustainability.
The bane of renewable energy is the inability, as of 2024, to store it. This makes it impractical to utilize renewables to power a factory, as the labor force would remain idle when the sun doesn’t shine, and the wind doesn’t blow.
With the IIoT, it may be possible to run a factory with fewer boots on the ground, with most decisions being made by digital decision engines. The computers could conceivably be backed up by a small core of shared engineers for those special instances where human judgment might be necessary. Hopefully, these instances will be increasingly rare, and their numbers might well be quite low, too.
Wrapping Up
Predictive maintenance employs sensors attached to critical points throughout a system being monitored, or being monitored and controlled. There is no possibility that any practical number of human observers could monitor so many data points, and even if they could, they couldn’t take multiple readings every second and properly record them.
Thanks to the IIoT, this information can be transmitted to remote servers that contain various types of analytical engines, including AI, that can pinpoint possible sources of problems or analyze the cause of existing problems. Through a process called Edge Computing, in many cases, this information can be analyzed at its source without waiting for decisions made thousands of miles away on remote servers.
The totality of this data, made available at the server, can aid in the production of a Digital Twin, essentially a simulator to analyze what will happen to the system as it is subjected to various conditions.
The public is well aware of predictive maintenance and its efficacy in the manufacturing environment, but it is also applicable for many other types of systems, as outlined in this report.
References
- Top IoT Sensors for Predictive Maintenance: https://patrickhq.medium.com/top-iot-sensors-for-predictive-maintenance-89e1391457e6
- The Role of IoT in Predictive Maintenance for Manufacturing Operations: https://www.threadinmotion.com/en/blog/the-role-of-iot-in-predictive-maintenance-for-manufacturing-operations
- Digital Twins: Examples and Use Cases: https://www.onlogic.com/blog/digital-twins-examples-and-use-cases/
- Practical Applications Of AI-powered Predictive Maintenance For Renewable Energy Infrastructure: https://www.forbes.com/councils/forbestechcouncil/2024/06/13/practical-applications-of-ai-powered-predictive-maintenance-for-renewable-energy-infrastructure/
- 5 Use Cases of Predictive Maintenance using IoT: https://webbylab.com/blog/use-cases-of-iot-based-predictive-maintenance/
- How AI scales up IoT capability in turbofan jet engines: https://www.rolls-royce.com/media/our-stories/discover/2020/intelligentengine-how-ai-scales-up-iot-capability-in-turbofan-jet-engines.aspx
Glossary of Key Terms
- Edge Computing. Allows for computational decisions to be made in the same physical location as the sensors that gather the input parameters, obviating the need for references to remote analytical engines accessible only through the internet.
- Predictive Analytics. The use of historical data and algorithms to predict future tendencies and likely events provides useful guidance for planners, enabling better strategic decisions.
- Digital Twin. A virtual version of a physical object, process, or location that serves as a real-time digital counterpart
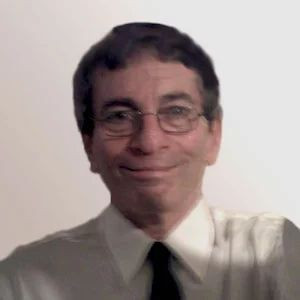