What Makes Smart Factories Smarter? Robotics, AI, and IoT
16-10-2024 | By Gary Elinoff
Key Takeaways about What Makes Smart Factories So Smart?
- Robotics, AI and IoT are among the key enabling technologies for smart factories
- Machine learning puts the “smart” into Smart Factories
- Decision-making is pushed down as close as possible to the actual physical action
- The robots are coming, but they won’t completely replace humans on the factory floor
Introduction
As described in an article by Forbes[1], the term Fourth Industrial Revolution was introduced by a team of German scientists and made mainstream by Klaus Schwab, the founder and executive chairman of the World Economic Forum (WEF). The first three eras, as defined by the WEF, were:
- First Industrial Revolution: 1784. Powered by the Steam Engine and Water Power
- Second Industrial Revolution: 1870. The Division of Labor, Electricity, Mass Production
- Third Industrial Revolution: 1969. Electronics, Information Technology, Automated Production
For Smart Manufacturing and the 4th Generation Smart Factories that are at its heart, robotics, AI (artificial intelligence) and the IoT (Internet of Things) are critical enablers. These factors are also the building blocks of some decidedly non-manufacturing endeavors, as varied as smart homes and inventory control.
The original term used to describe the Internet as a means for non-human communication, as described in our article The Industrial Internet of Things (IIoT), Because Machines Get Lonely, Too, was, in fact, the IIoT.
Since then, the proliferation of non-factory applications has caused the less specific IoT designation to take precedence. Electropages has published numerous articles concerning these game-changing endeavors, including AI and Robotics to Play Key Roles in Space Exploration and Agricultural Artificial Intelligence: Because it’s a Hungry World Out There.
However, this article will zero in on the 4th Industrial Revolution’s dramatic effect on manufacturing.
How IoT Sensors and Connectivity Transform Industrial Monitoring
It all begins with electronic sensors. Inexpensive, power stingy and reliable, these devices can measure vibration, heat, humidity, voltage, current and many more important parameters. They can do it many times per second, or they can “sleep” most of the time, drawing even less power. Sensors can be placed anywhere in the factory, on any machine.
But how do the sensors know if and when to take readings? How is all that information garnered and brought to one or more central points where the combined wealth of information can be processed and analyzed? That’s where the IoT comes in, and it takes many forms.
The sensors can connect, through various modalities, directly to the Internet. Then, data centers located anywhere in the world can command any sensor to take a reading or a sensor that logs a reading that exceeds per-programmed limits can itself initiate a “dialog”.
Digitized information that reaches a data center can be interpreted by powerful analytical engines to determine the “health” of the machine. And, of course, if a dangerous situation is observed, digital commands can be sent back down to cause, for instance, a shutdown of electricity to a subsystem, or cause a vital valve to open or shut.
More often, analysis also takes place locally, at the factory. One reason for this is that it takes a finite amount of time for signals to reach a data center located far away and more time for the response to reach back to the factory. In an emergency, that may be too late.
The outputs of many different sensors might be analyzed to determine how well a piece of capital equipment is performing or if a perilous situation is brewing. To make local analysis possible, each sensor must be able to communicate with its peers and with local analytical engines. It might be, for instance, that the output of many different sensors must be analyzed to determine how well a piece of capital equipment is performing or if a perilous situation is brewing.
That communication takes place on one of the many modalities of the IoT, and using the IoT for machine monitoring means that devices can be moved with no need for new cabling. We analyze the possibilities in our article IoT Wireless Communication: The Core of Industrial IoT Connectivity. Spoiler Alert! 5G may not offer a big advantage to ordinary smartphone users, but it’s playing an ever larger role in smart factories.
Harnessing Machine Learning for Smarter Factory Automation
Machine Learning (ML) puts the “smart” into smart factories. A subset of AI, it’s what allows computers to learn from experience, in a manner that mimics living organisms. Machine learning allows computer systems to respond autonomously to new situations and most importantly, to situations for which they have never been specifically programmed for.
For example, a troublesome situation may occur on the factory floor, and at the same time, a number of sensors may report some specific values. The system then “learns” that those specific patterns may presage that negative situation. We say “may presage” because if the negative event does not occur, ML looks for other sensor responses that may modify the earlier original preliminary answer. Over time, ML builds up an ever more specific library of patterns that can point out dangers that might never have occurred to human planners.
Significantly, these patterns are discovered by ML with no specific input from any human agency. In effect, the machine has learned on its own.
IBM’s Jeff Crume explains these difficult concepts in a simple, straightforward manner in his masterful video AI, Machine Learning, Deep Learning, and Generative AI Explained [2].
How Predictive Maintenance Reduces Downtime and Enhances Efficiency
Even without ML, large numbers of sensors located throughout a critical machine can make any number of measurements as often as desired. These readings can be digitally transferred to a central location, where they are available to analysts. But it doesn’t end there.
As described in the previous section, machine learning data engines can build up ever more extensive libraries of patterns. Engineers and managers will automatically learn if a component in a machine is likely to fail, and they will be informed of the reasons why. This is known as predictive maintenance, and it is one of the hallmarks of the smart factory.
This happens with no need for in-person inspections with voltmeters, oscilloscopes, or any other type of test equipment or maintenance tool. Technicians and inspectors can be spared from this boring and occasionally dangerous work, and indeed, smart manufacturing makes it possible to find more potential faults before they happen. The result is that downtime and costs are reduced, and the information gleaned from the process makes it possible to unearth previously hidden pathways to improve overall efficiency.
In a previous Electropages article, Predictive Maintenance: Outperform Technicians with IoT, we delve deeper into this process.
The Role of Edge Computing in Smart Factory Efficiency and Security
Smart manufacturing relies heavily on powerful AI engines residing on remote servers, but it’s now technologically possible to install AI chips right onto or close to the smart factory machines being monitored. This won’t eliminate the role of server-based analytical engines but rather supplement them.
Edge computing. Image source: FSP
Modern Western organizations, both military and commercial, emphasize the benefits of local management in making decisions rather than deferring to remote authorities. In the case of smart factories, the reasons, according to Intel[3], for preferring local decisions over decisions made by remote servers include:
- Operational speed and efficiency
- Cost-effectiveness
- Energy conservation
- Security and data sovereignty
The first reason holds true because decisions made by edge-based AI are not subject to possible internet latency. The second is that controlling and monitoring actions on the factory floor involves the generation and transmission of enormous amounts of information. The amount of IIoT bandwidth required doesn’t come cheaply, and handling as much as possible remotely saves on that cost. The third reason is because much power hungry network hardware is eliminated.
The fourth reason is perhaps the most important. The less information transmitted over the Internet means that there is less of an opportunity for malefactors to hack or spoof the system.
How Robotics and Cobots Transform Smart Manufacturing
Robotics applications have bloomed in smart factories. The levels of observation and control that are defining characteristics of smart manufacturing make it possible to entrust more complex or dangerous tasks to robots.
Following the unmistakable smart manufacturing trend of allowing more and more decision making to take place as close to the action as possible, industrial robots are often equipped with their own local AI as well as machine vision.
As noted by Active Silicon[4], there are two schools of thought about the future of robots in the factory. One is the dystopian notion that the machines take over. The other is that robots will only be assigned the most dangerous, boring or repetitive task.
But there is a middle ground. One of the most active areas of robotic research is in the field of collaborative robotics. These devices, aka Cobots, are designed to work in close proximity to human coworkers in a manner that is completely safe for the human. In this manner, human intuition and versatility combined with robotic solidity can achieve more than either actor can on their own.
Challenges and Opportunities
Smart manufacturers will want as much of the necessary AI-based decision-making to take place as close as possible to the machines being monitored and controlled. Vendors will respond, and the steady movement towards edge computing will continue.
Manufacturers face a greater challenge than this in that they will need a whole new type of manufacturing worker. This new person will be more technician than anything else, and they’ll have little tolerance for repetitive work. Indeed, part of their job will be to detect mindless repetitive tasks and work with engineers to find ways to delegate them to a machine.
But where will these smart factory workers come from? Postsecondary education in the US is not responding to this challenge. Additionally, the practical minded souls who are suited to this kind of work will not be willing to spend vast amounts of money for training as were their more academically minded peers in times past.
All sides in the US are committed to bringing more manufacturing home to the US, but it can’t happen unless people are trained.
Wrapping Up
It is AI that puts the smart into smart factories. Without AI, a not-so-smart factory can only respond to the set number of situations for which it has been pre-programmed. It is AI that allows the factory to essentially learn from experience. Through AI, the factory gets constantly smarter and is able to react more wisely to ever-changing circumstances and challenges.
The IoT, or the IIoT if you prefer, is the glue that links together the varied components of the smart factory. It is also what connects the smart factory to server-based decision engines and remote monitoring ports. Without the IoT. Smart factories could still be smart, but the intelligences would be “islands of automation”, unable to collaborate effectively.
In a manner similar to Western militaries and industrial organizations, smart manufacturing stresses the ability to make decisions as close to where “the rubber meets the road” as possible. This ability is greatly enhanced through the use of edge computing.
And finally, as evinced by the continued development of Cobots, it is clear that human beings still have a future on the factory floor – for now!
References
- Future-Proof Your Business With Smart Manufacturing. Forbes
- AI, Machine Learning, Deep Learning and Generative AI Explained. IBM Technology
- Edge AI. Intel
- Active Silicon’s AI Series. Active Silicon
Glossary of Terms
- Machine Learning (ML). A branch of AI that enables computer systems to respond autonomously to situations for which they have never been specifically programmed for.
- Collaborative robot. Also known as Cobots, these devices are designed to work safely and collaboratively with humans with no safety barriers required.
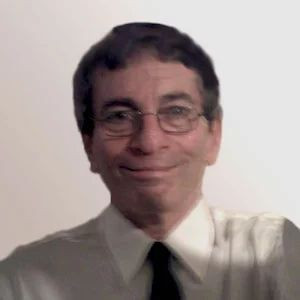