How IIoT and Machine Learning Transform Energy Management
07-11-2024 | By Gary Elinoff
IoT – Transforming Energy Industry.
Key Takeaways about The IIoT and Machine Learning enable effective Energy Management:
- Smart meters, the Internet of Things and AI-based analytic engines are key technologies that enable energy management.
- There are many similarities between energy management and efficient factory management.
- In order to make it possible to employ a greater percentage of renewable energy in the national power grid, greater progress in energy management will be essential.
Introduction
The goal of Energy Management is not only to use less energy, but also to use it more effectively. This is a critical part of the goal towards enhanced sustainability and the eventual goal of net carbon neutrality.
Sensors such as smart meters can measure actual energy usage at any point at specific times, and analytical engines, often powered by AI, are employed to analyze the data. The data points can come from multiple locations located anywhere and the analytical engine may be thousands of miles away. The critical glue that holds it all together is the IIoT.
The Industrial Internet of Things
The Industrial Internet of Things (IIoT) is often referred to as the Internet of Things (IoT). For a detailed description of how this came about, see "The Industrial Internet of Things (IIoT), Because Machines Get Lonely, Too." For our purposes here, suffice it to say that the IoT, or IIoT if you prefer, is simply a way of referring to the internet when it's used primarily for machine-to-machine communication rather than by human beings.
Smart Meters are a key technology for Energy Management
An electronic sensor is a device that measures a physical parameter, such as electrical current, into a digital representation. And, as defined by IoT Analytics [1], "A smart electricity meter is an electronic IoT device used in measurement systems deployed by utility service providers (USPs) to gauge various parameters in distributing electricity to consumers.
Smart electricity meters have enjoyed wide application. Image source: IoT Analytics
Smart meters can make commercial users of electricity more aware of the amount of electricity they use and when they use it. This knowledge may enable them to move the most energy intensive portions of their production cycle to times when energy is less expensive, enabling them to cut costs.
The Role of Data Analytics in Energy Management
Raw data collected from the sensors and transported via the IoT is still just raw data. Sure, an undercurrent at one point is actionable information, but it isn't always that simple. For energy management to be useful, patterns have to be analyzed, and insights need to be drawn. That's the job of data analytics.
As described by SAP[2], "Data analytics is the process of analyzing, interpreting, and visualizing large, complex datasets to derive meaningful insights and make informed decisions. It encompasses a variety of techniques from statistical analysis to machine learning, aimed at analyzing past and current data to predict future trends."
The process begins with the IoT gathering the information from the sensors. Presented with the data, it is AI's job to glean useful information from what, to a human, would be a bewildering array of patterns. However, can useful information be garnered from this jumble?
In a previous Electropages article, What Makes Smart Factories Smarter? Robotics, AI, and IoT we describe how AI, and particularly Machine Learning (ML), achieves the goal of deriving useful information from masses of seemingly unrelated data.
An unwanted power surge may appear at one point. An instant earlier, two conditions may appear unexpectedly at other points. Then it happens again a day later. A pattern indicating a fault? Maybe, since the same pattern again occurs at another time.
But not so fast! What if the same two "input" conditions occur again without the expected bad output? The AI may note that another unexpected condition occurs elsewhere that may have forestalled the negative result. And so it goes on, but even at this point, energy efficiency analysts have information that will prove useful.
The important thing to keep in mind is that in a complex energy system, there are far too many data points, with data streaming out of them perhaps hundreds of times per second, for a human analyst to discern useful patterns. But it's not too much or too fast for the electronic machine learning system.
Use Cases for Data Analytics in Energy Management
As described by Lemberg Solutions[3], a partner of STMicroelectronics, there are eight important use cases for Data Analytics in Energy Management.
Eight Use Cases for Data Analytics in Energy Management. Image source: Lemberg Solutions
- Grid Modernization. By monitoring the power grid's performance, utilities can detect anomalies and weak spots, leading to an optimized energy management strategy. This will eliminate potential capacity constraints and minimize the likelihood of network outages.
- Smart Energy Management Systems. Based on inputs from smart meters and sensors, sustainable and efficient ways to control and intelligently distribute energy in home and industrial settings are derived.
- Predictive Asset Management. Predictive maintenance for factory settings was described in detail in Electropages' article Predictive Maintenance: Outperform Technicians with IoT. It works similarly in the realm of energy management.
- Demand Forecasting. Data analytics can analyze inputs as varied as past consumer consumption patterns and weather patterns and can thus predict demand periods, enabling utility companies to fine-tune their energy distribution strategies.
- Outage Prediction. Employing predictive models to anticipate disruptions or outages due to weather or natural disasters.
- Renewable Energy integration. Data analytic methods are employed to predict the availability of intermittent renewable inputs. This will ultimately support the optimal geographic placement of solar panels and wind turbines, with the goal of utilizing as little nuclear or carbon based power sources as possible.
- Theft detection. With data points constantly monitored and analyzed, patterns suggesting theft can be more easily detected.
- Load management. Effective load management is critical to grid stability and for avoiding blackouts. Analytics can uncover inefficient or overly congested power distribution routes. Peak demand periods and consumption patterns can be highlighted for improved grid efficiency.
Challenges and Opportunities
Because of the intermittent availability of wind and solar power, no field has more to benefit from IoT based data analytics than does the utilization of renewable energy. In North America, the wind and the sun will never all be blowing and shining at the same time in all locations, so the key to their effective utilization is knowledge.
Systems can determine where excesses of power are available, and where there is a deficit. Peaker plants, natural gas based power generation plants that can be quickly turned on if more power is needed, can be made ready if a need is predicted. Analytic engines can determine what the most efficient electrical pathways are.
It must be kept in mind that as of Fall 2024, there is no effective way to store utility scale solar power for more than a few hours. If renewables are to play a greater role in the energy mix, this ability to transfer and redistribute energy on a continent wide basis instantly, along with an ever more muscular system of power lines, will be essential.
Wrapping Up
The process of managing energy is very similar to that of putting the "smart" into smart factories. Both depend on smart sensors to measure physical values, insert a time stamp and then to digitize the information. Then, the IIoT gathers the data from the manufacturing campus in the case of a factory complex or from the four corners of a nation or continent in the case of energy management.
Analytical engine(s), located anywhere, look for patterns and draw conclusions for a more efficient factory or for a more resilient power grid.
In the case of the energy grid, it will not only cause energy to be used more efficiently, but it will also make it possible to use a greater percentage of renewable energy. Despite what the dreamers believe, net carbon neutrality is not possible today, but energy management based on the IIoT will make it possible to use less fossil fuel, and hopefully no nuclear energy at all.
References
- Smart electricity meter market 2024: Global adoption landscape. IoT Analytics
- What is Data Analytics? SAP
- Data Analytics in the Energy & Utilities Sector: 8 Business Use Cases. Lemberg Solutions
Glossary of Terms
- Machine Learning (ML). A major branch of AI that allows computational systems to actually learn from experience. Through this discipline, digital systems can respond with high degrees of certainty to conditions for which they haven't been explicitly programmed for.
- Predictive Maintenance. Using the IIoT to garner inputs from smart meters and from sensors, analytic engines, often based on AI, can make predictions of trouble.
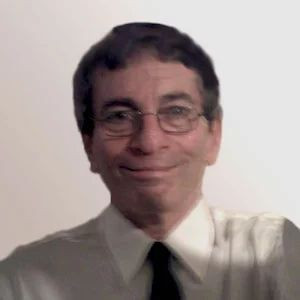