Intel Xeon 6 for AI: Enhanced CPU Inference & HPC Speed
11-11-2024 | By Robin Mitchell
Intel's latest advancements in AI technology have sparked significant interest and speculation within the tech community. With the introduction of the Xeon 6 AI Accelerator and discussions surrounding Gaudi 3 Pricing, the landscape of AI computing is poised for a potential transformation. As Intel explores the vast opportunities for AI integration with its Xeon processors, the industry eagerly anticipates the implications of these developments.
Key Things to Know:
- Intel's new Xeon 6 AI Accelerator is set to transform AI computing with enhanced core performance and efficiency, challenging established GPU-heavy solutions.
- The AI industry faces significant challenges with the high computational demands of AI inference and the limitations of traditional CPUs.
- Intel's entry into the AI market with Xeon processors aims to diversify hardware options and reduce dependency on GPU providers like NVIDIA.
- While NVIDIA remains a key player, Intel's Xeon range offers an alternative approach, potentially levelling the playing field in AI computing hardware.
How will Intel's Xeon 6 AI Accelerator redefine the capabilities of AI computing within existing systems, what competitive advantages does Intel's approach offer compared to Nvidia's solutions, and how might these innovations shape the future of AI-driven technologies across various sectors?
What challenges does AI inference face?
The integration of Artificial Intelligence (AI) into everyday life is burgeoning at an unprecedented pace, with new AI-centric companies emerging frequently. This rapid growth has led some observers to speculate that the sector might be experiencing a bubble similar to the dot-com bubble of the late 1990s. During that period, a large number of internet startups emerged rapidly and just as quickly vanished, leaving only a few survivors who then dominated the industry.
Technical Hurdles in AI Deployment
While it remains to be seen whether the AI industry will undergo a similar consolidation, the current phase of expansion presents numerous technical challenges, particularly for engineers tasked with integrating AI into applications and developing new models.
One of the most formidable challenges in the deployment of AI technologies is meeting the intensive computational demands required for AI training and operation. Traditional Central Processing Units (CPUs) are versatile and efficient at handling a wide range of computing tasks. However, they are not optimised for the highly parallel and computationally intensive tasks typical of AI processes, particularly in the training phase. This inadequacy stems from their architectural limitations, which do not allow them to process large blocks of data simultaneously—a key requirement for machine learning algorithms.
CPU Limitations in AI Training and Processing
To address these limitations, many engineers have turned to Graphics Processing Units (GPUs). Originally designed to handle the complex mathematical calculations required for rendering images and videos, GPUs are well-suited to the parallel processing demands of AI. They can perform many operations concurrently, dramatically speeding up the training and operation of AI models. However, this capability comes at a cost. GPUs are not only expensive but also require significant amounts of power, which can be a limiting factor in scaling AI applications.
Due to the high costs and power requirements of GPUs, many organisations rely on data centers equipped with these processors. While this arrangement offers the necessary computational power, it introduces other challenges such as data privacy concerns, high operational costs, and potential delays in deployment times. These factors can inhibit the agility and efficiency of AI projects.
Challenges of GPU-Driven Data Centres
In search of more sustainable solutions, some researchers and companies are exploring alternatives to CPUs and GPUs. One such alternative is the Neural Processing Unit (NPU). NPUs are specialised hardware designed explicitly for neural network computations. They offer efficient processing capabilities at lower power consumption than GPUs, making them particularly useful for edge computing applications where power availability and data privacy are critical concerns.
However, the development of Neural Processing Units (NPUs) is not without its own challenges. NPUs are costly to develop and are currently suitable only for specific types of AI tasks. This specialisation means that their adoption requires sacrificing silicon space that could otherwise be used for memory and other circuits, potentially limiting the versatility of the devices they are embedded in.
The choice between CPUs, GPUs, and NPUs involves a complex trade-off among processing power, energy consumption, cost, and the specific requirements of the AI application. Engineers must navigate these trade-offs, considering not only the technical capabilities of each processor type but also the broader implications for the scalability, efficiency, and privacy of AI systems.
Intel's Latest Xeon Range Could Empower Future AI
In a recent unveiling, Intel has introduced its sixth-generation Xeon server CPUs, the Xeon 6900P series, which marks a significant advancement in the field of AI computing. This new series, which scales up to 128 cores, is designed to optimise both performance and power efficiency, catering to the increasing demands of artificial intelligence applications.
Intel® Xeon® 6 processors with Performance-cores (P-cores) include integrated matrix engines designed to boost performance for demanding AI and high-performance computing (HPC) tasks.
The Xeon 6900P processors, with their substantial increase in core count and memory speed capabilities, are positioned as a pivotal development in CPU technology, particularly for tasks involving AI inferencing. Intel's approach with these processors is two-pronged: they are suitable for use in CPU-only servers for inferencing tasks, and they serve effectively as host CPUs in systems that are accelerated by more power-intensive GPUs, such as those from Nvidia.
P-Core and E-Core Flexibility for Diverse Applications
With its unique combination of P-cores and E-cores, the Xeon 6900P series offers flexibility for various AI use cases. P-cores are tailored to handle high-throughput computations, while E-cores manage lighter workloads, helping balance power efficiency and processing strength across a system. This adaptability allows IT teams to scale infrastructure according to their AI-driven tasks, ensuring cost-effective deployment while maintaining optimal performance.
Intel's latest processors also introduce several enhancements, such as support for DDR5 MRDIMM modules, which offer improved bandwidth and latency over standard memory modules. Additionally, the inclusion of Ultra Path Interconnect 2.0 links and up to 96 lanes of PCIe 5.0 and CXL 2.0 connectivity further augment the data handling capabilities of these CPUs, crucial for complex AI computations.
Moreover, the Xeon 6900P series supports the latest Intel QuickAssist Technology (QAT) and Data Streaming Accelerator (DSA), which streamline data encryption, compression, and transfer, enhancing the system's ability to handle large datasets swiftly. This is crucial for real-time AI analytics, where data processing speed can determine the success of an application, particularly in industries like finance and healthcare where latency reduction directly impacts operational efficiency.
Advanced Data Handling and Real-Time Analytics Capabilities
One of the standout features of the 6900P series is the support for the 16-bit floating point numerical format in the processor's Advanced Matrix Extensions. This feature is specifically tailored to accelerate AI workloads, which is increasingly relevant as businesses continue to integrate AI into their operational frameworks.
In addition to the advanced floating-point capabilities, Intel's Xeon processors support the bfloat16 and int8 data types, expanding the processors' AI performance range. These data formats are specifically optimised for AI applications, reducing memory consumption while maintaining model accuracy. This addition underlines Intel’s focus on optimising processors to meet the diverse needs of modern AI workloads, from smaller edge AI applications to large-scale data centre inferencing.
The company has also emphasised cost efficiency, particularly through innovations like the flat memory mode enabled by CXL 2.0. This allows data center operators to balance cost and performance by utilising a mix of DDR4 and DDR5 memory, thus optimising overall expenses without significantly impacting performance.
Cost-Effective Memory Solutions for AI Data Centres
Intel's flat memory mode, enabled by CXL 2.0, offers a strategic advantage for data centre operators looking to scale affordably. By allowing operators to mix DDR4 and DDR5 memory, it enhances cost-effectiveness without sacrificing memory performance, addressing one of the biggest challenges in AI infrastructure — cost scalability. This approach not only supports AI-driven environments but also extends the lifetime of existing hardware investments, a critical factor for long-term sustainability.
Intel's strategic focus with the Xeon 6900P series is not just on raw performance but also on the broader application in AI-driven markets. The processors are engineered to be a superior choice for hosting CPUs in systems accelerated by external GPUs or Intel's own upcoming Gaudi 3 accelerator chips, which are also tailored for AI applications.
From a competitive standpoint, Intel's new Xeon CPUs are positioned to outperform rivals such as AMD's EPYC processors in tasks related to AI, such as inferencing with large language models. This is a critical area of growth as AI continues to permeate various sectors, requiring more robust and efficient computing solutions.
Designed with data privacy in mind, Intel's Xeon 6900P series incorporates Intel Software Guard Extensions (SGX) and Intel Trust Domain Extensions (TDX), providing robust options for confidential computing. These features allow organisations to process sensitive data with assurance, a growing necessity as industries like healthcare and finance increase their reliance on AI for secure and private data analytics. This capability adds a layer of security that competitive processors may not offer at the same scale, enhancing Intel's appeal for privacy-conscious applications.
Could GPU Makers Such as NVIDIA Loose Out?
The artificial intelligence sector has grown exponentially, and with this growth, the demand for powerful computational hardware, particularly Graphics Processing Units (GPUs), has surged. NVIDIA, a leader in the GPU market, has become a pivotal player in this realm. The company's GPUs are highly sought after for their ability to efficiently handle the complex computations required in AI applications, from neural network training to data processing. This dependency on NVIDIA's technology is reflected in its rising stock prices, signalling strong market confidence in its continued dominance and innovation.
However, this reliance on a single supplier for GPUs raises concerns about market monopolies and the stifling of innovation. In response, engineers and developers are actively seeking alternative technologies that can provide competitive performance in AI applications. This search for alternatives is not just about finding different hardware but is also driven by the desire for more flexibility and freedom in the development of AI technologies.
Intel's Xeon Range Enters the AI Market
The introduction of Intel's Xeon range holds the promise of democratising the field of AI. By providing more options for AI hardware, Intel not only challenges the current market dynamics but also encourages a more competitive environment. Competition, as a rule, fosters innovation; with more players in the market, new features, optimisation techniques, and cost-effective solutions can emerge. This diversification of hardware options also prevents any single entity from monopolizing the AI market, thus ensuring that no single company can dictate terms or limit access to these crucial technologies.
Moreover, the availability of alternative platforms like Intel's Xeon could lead to broader experimentation and faster innovation within AI. Engineers and developers could tailor their hardware choices more closely to the specific needs of their projects without being constrained by the limitations of a single technology provider. The ability to choose from a wider range of hardware solutions also means that smaller companies and startups will have better access to the necessary tools to develop and deploy AI solutions, potentially levelling the playing field in an industry that is currently dominated by tech giants.
Despite these potential shifts, NVIDIA's GPUs continue to be a formidable force in the AI market. Their established reputation, coupled with continuous advancements in GPU technology, makes them a tough competitor to outpace. NVIDIA's deep learning and general AI capabilities are deeply integrated into the fabric of current AI development workflows, setting a high standard for any new entrants. As such, it is likely that NVIDIA will maintain a significant hold on the AI market for the foreseeable future.
In conclusion
hile NVIDIA's dominance in the AI GPU market is undisputed, the entry of Intel's Xeon range introduces a new dynamic to the industry. This development not only challenges the status quo but also holds the potential to enrich the AI landscape by providing more technological diversity and fostering an environment conducive to innovation. As the AI sector continues to evolve, the impact of such competition will undoubtedly play a critical role in shaping its future trajectory, driving both technological advancements and market growth.
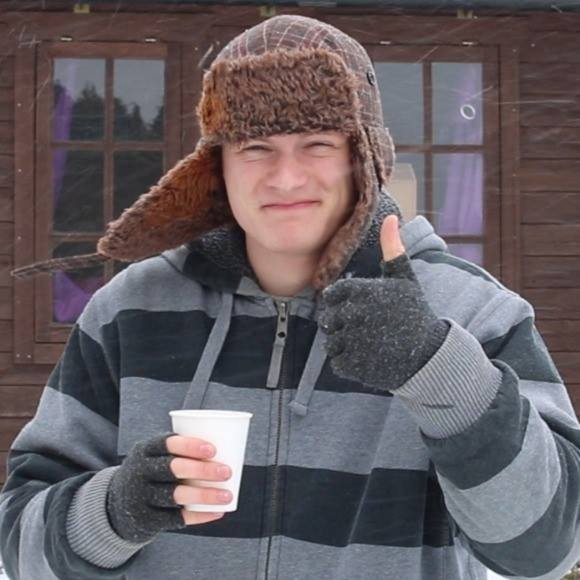