Raspberry Pi of AI: Affordable NPUs from Korean Innovators
Insights | 12-11-2024 | By Robin Mitchell
A Korean startup is making waves in the AI hardware industry with its ambitious goal of democratising access to high-performance Neural Processing Units (NPUs) at an unprecedented price point. By leveraging a proprietary quantisation technique, the company aims to drive the cost of NPUs down to an astonishing $1 per TOPS (Tera Operations Per Second), potentially opening the doors for AI integration in billions of devices worldwide. This disruptive approach could transform the AI hardware landscape, making advanced neural network processing power more accessible than ever before.
Key Things to Know:
- DeepX, a Korean AI technology company, is pioneering affordable Neural Processing Units (NPUs) designed for low-cost AI inference, aiming to bring high-performance AI processing to billions of devices.
- The company’s V1 and M1 chips, showcased at the 2024 Embedded Vision Summit, support advanced AI applications with energy-efficient designs, making them ideal for edge computing in fields like smart cities, robotics, and healthcare.
- In partnership with LG, DeepX is developing NPUs optimised for Large Language Models (LLMs), targeting mobile devices, automobiles, and home appliances to boost on-device AI capabilities while preserving user privacy.
- With plans for the V3 chip, which addresses regional security and compatibility needs, DeepX continues to evolve its product line to meet diverse global market demands for efficient, cost-effective AI solutions.
How does the startup's secret quantisation technique enable such a significant cost reduction for NPUs, what impact could the widespread adoption of ultra-cheap, high-performance NPUs have on various industries and applications, and what challenges might the company face in achieving its ambitious $1/TOPS target in a competitive market dominated by established players?
What challenges does AI face in SBC applications?
Artificial intelligence (AI) is currently being integrated into a vast array of applications, from autonomous vehicles navigating city streets to precision agriculture techniques optimising crop yields. This proliferation of AI technology promises to bring about significant efficiencies and advancements across numerous sectors. However, the integration of AI into single-board computers (SBCs) and small-scale applications such as the Internet of Things (IoT) presents a unique set of challenges, particularly in terms of computational demands and energy requirements.
One of the primary challenges is the energy consumption associated with running AI algorithms. AI processes, especially those involving machine learning and deep learning, require substantial computational power. For battery-powered devices, which many IoT devices are, the high energy demand makes it impractical to run AI directly on the devices' CPUs. CPUs, while versatile, are not particularly efficient at handling the parallel processing tasks that AI algorithms require.
Role of GPUs in Mitigating AI Processing Challenges
To address this inefficiency, GPUs (graphics processing units) are often employed as they are better suited for parallel processing tasks central to AI operations. GPUs can significantly reduce power consumption compared to CPUs when running AI applications. However, incorporating GPUs into small-scale devices like SBCs introduces new challenges. GPUs are not only more expensive, but they also add complexity to the hardware design. They typically require more space and better heat dissipation, which can be problematic in compact devices designed for simplicity and low cost.
Computational Limitations in Low-Power SBCs
Moreover, even for relatively simple tasks, AI can be computationally demanding, which poses a problem for SBCs and IoT devices that are typically low-powered and designed for efficiency rather than high performance. The limited processing power available in these devices can severely restrict the complexity of the AI tasks they can handle.
One commonly proposed solution to these challenges is to run AI computations off-chip, meaning that the data collected by IoT devices is sent to a remote server where the AI processing occurs. This approach can alleviate the computational load on the device itself and circumvent the issues related to power consumption and hardware limitations. However, off-chip AI processing introduces its own set of challenges, particularly related to connectivity and latency.
Latency and Network Requirements for Off-Chip AI
Latency is a critical issue, especially for applications requiring real-time processing, such as autonomous driving systems. The delay introduced by transmitting data to a remote server and receiving the processed data back can render real-time decision-making impossible. Additionally, maintaining remote connections requires robust network infrastructure, which can be costly. Local area networks managing numerous IoT devices need to handle significant amounts of data and may require complex network configurations with multiple subnets.
Historically, AI has been developed and trained in large data centers equipped with the necessary infrastructure to handle intensive computational tasks. These environments are designed to optimise AI processing with high-performance servers and ample electrical power. Deploying AI in such settings is a stark contrast to the environments where SBCs and IoT devices operate, which are constrained by power, size, and cost considerations.
Korean Startup Developing NPUs for low-cost AI inference
In a recent unveiling at the 2024 Embedded Vision Summit, DeepX, a South Korean AI technology company, showcased its innovative first-generation chips, the V1 and M1, marking a significant stride in the field of on-device artificial intelligence. DeepX, known for its deep learning solutions utilised in various sectors, including autonomous systems, robotics, and healthcare, also provided a glimpse into its future roadmap with the announcement of its next-generation chip aimed at enhancing AI capabilities in on-device and autonomous robot applications.
DeepX’s strategic entry into the AI semiconductor market is not only driven by demand for on-device AI but also by the limitations of cloud-based AI systems. Industries such as smart cities, healthcare, and autonomous robotics are increasingly seeking real-time processing capabilities to minimise data transmission delays and enhance security. By enabling on-device AI, DeepX aims to address these demands effectively while catering to a growing market for low-cost, high-efficiency AI chips.
V1 SoC: Balancing Power and Affordability
The V1 System on Chip (SoC), formerly known as L1, integrates the DeepX 5-TOPS (Tera Operations Per Second) Neural Processing Unit (NPU) with quad-RISC-V CPUs and a 12-MP image signal processor. Manufactured using Samsung's 28-nm process technology, the V1 SoC supports advanced convolutional neural network (CNN) algorithms and is tailored for products such as IP and CCTV cameras, robotic cameras, and drones. Notably, this chip is capable of running the YOLO v7 model at 30 frames per second while consuming only 1-2 watts of power, all under a budget-friendly sub-$10 price point.
In addition to its affordable pricing, the V1’s design demonstrates DeepX’s commitment to energy-efficient AI solutions. Leveraging a proprietary low-power architecture, the V1 operates within 1-2 watts, a key factor in applications like autonomous drones and CCTV cameras where battery life and thermal management are critical. This approach positions the V1 as a cost-effective yet powerful solution for AI-driven tasks at the edge, aligning with the needs of resource-constrained devices.
On the other hand, the M1 serves as a more robust accelerator designed to complement a host CPU. This chip excels in cost-efficiency, power efficiency, and performance efficiency, boasting an AI performance of 25-TOPS and a power consumption of just 5 watts. Its applications are broad, ranging from consumer and industrial robots to smart factories and edge computing.
The Versatile M1 Accelerator & AI-Powered Edge Computing for Industry 4.0
DeepX’s M1 chip further enhances AI integration in edge computing and robotics, where data processing is increasingly decentralised. By supporting over 30 frames per second on multiple video channels, the M1 enables real-time image recognition and analytics, making it a valuable asset for industrial applications and automated quality control in manufacturing. Such capabilities are particularly advantageous as industries move towards smart automation and Industry 4.0 standards.
A significant development in DeepX's strategy is its partnership with LG. According to DeepX CEO Lokwon Kim, this collaboration aims to integrate Large Language Models (LLMs) into DeepX's chips for deployment in mobile devices, automobiles, and white goods. This synergy is expected to optimise the NPU chips for LLMs, enhancing their functionality in on-device applications. However, it's anticipated that achieving a fully LLM-capable SoC will require an additional 3-5 years of development.
The integration of Large Language Models (LLMs) on DeepX’s NPU chips could transform on-device AI by enabling natural language processing tasks directly on end-user devices. This shift supports a broad range of applications, from voice-activated home appliances to smart automotive interfaces, without relying heavily on cloud services. As regulatory and consumer pressures for data privacy rise, localised processing of LLMs becomes a significant advantage, reducing data exposure to external networks.
Benefits of Localised AI Processing
Looking ahead, DeepX plans to release the V3 chip, which addresses specific customer feedback from the Chinese and Taiwanese markets. The V3 will feature a 15-TOPS dual-core DeepX NPU and quad-Arm Cortex A52 CPU cores, a shift from RISC-V to Arm architecture in response to customer demands for better security solutions and compatibility with the robot operating system. This chip will also include USB 3.1 and a more powerful image signal processor, operating below 5 watts on average.
The forthcoming V3 chip demonstrates DeepX’s responsiveness to regional needs, such as security compliance and compatibility with popular robotics platforms. By adopting Arm architecture, the V3 provides improved support for the Robot Operating System (ROS), a key requirement for robotics in industrial and logistics sectors. This shift reflects DeepX’s strategic adaptability and its commitment to addressing customer feedback to enhance product relevance in diverse markets.
Are NPUs the answer to future AI inference?
Unlike traditional central processing units and graphics processing units, NPUs are specifically designed to handle AI tasks, thereby offloading these tasks from CPUs and GPUs. This specialisation not only enhances processing efficiency but also significantly cuts down on the power and computational demands of systems, making NPUs particularly advantageous for designs where energy efficiency is paramount.
One of the most compelling uses of NPUs is in battery-powered systems, where conserving energy is critical. By minimising the need for constant connectivity, which is typically required for cloud-based AI processing, NPUs can operate more autonomously, relying less on external data centers and more on local processing. This capability is not only beneficial for power management but also enhances the functionality of devices in environments where connectivity is limited or unreliable.
Privacy and Security Benefits of Local NPU Processing
Moreover, the integration of NPUs can bolster privacy and data security—an increasingly vital concern in today's digital age. By processing data locally rather than sending it to remote servers in data centers, NPUs allow for sensitive information to be handled within the device itself. This architecture reduces the vulnerability of personal data to breaches and unauthorised access, a significant advantage given the growing scrutiny over data privacy practices globally.
However, it is important to recognise the limitations of current NPU technology. While NPUs are adept at accelerating the inference phase of AI—which involves applying a trained model to new data—they are generally not capable of training AI models themselves. Model training is a computationally intensive process that, as of now, still requires the robust capabilities of large data centers. Consequently, while NPUs can enhance the speed and efficiency of AI applications, they do not entirely eliminate the need for powerful CPUs and GPUs, which are capable of handling complex model training tasks.
Expanding NPU Capabilities for Low-Power Devices
Despite this limitation, the impact of NPUs on the technology landscape is undeniable. A Korean startup, for instance, is making significant strides in developing NPUs that promise to enhance the capabilities of single-board computers (SBCs) and other low-power devices. By enabling these devices to execute sophisticated AI tasks efficiently, NPUs are opening up new possibilities for smarter, more capable computing devices that maintain a low energy footprint.
Looking to the future, it is highly plausible that NPUs will become a standard component in a wide array of computational devices, including PCs, laptops, and smartphones. As AI becomes increasingly embedded in everyday technology, the demand for efficient, powerful, and energy-conserving AI processing will escalate. NPUs are poised to meet this demand, heralding a new era in which AI is both omnipresent and sustainably integrated into the fabric of technological innovation.
In conclusion
While NPUs currently support primarily AI inference rather than training, their role in reducing energy consumption, enhancing privacy, and enabling powerful AI capabilities in low-power devices underscores their critical importance in the evolution of computing technology. As such, the continued development and integration of NPUs will undoubtedly play a central role in shaping the future landscape of artificial intelligence and computational devices.
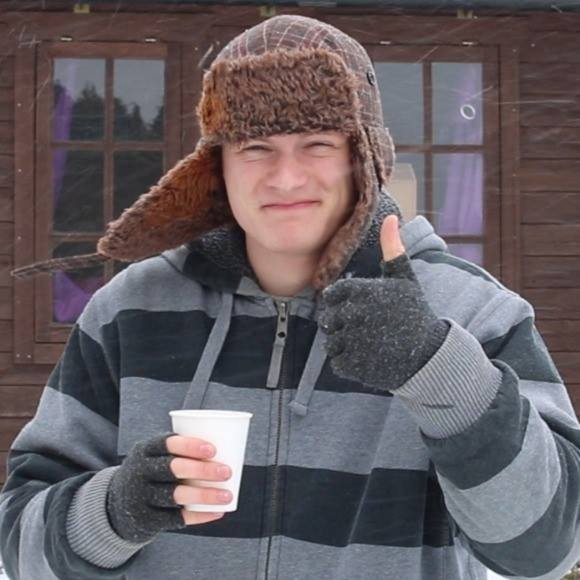