RoVi-Aug: Solving Challenges in Robotic Task Adaptation
12-12-2024 | By Robin Mitchell
RoVI-Aug utilises advanced diffusion models to augment robot images, generating synthetic visuals featuring diverse robots and viewpoints. Policies trained on this augmented dataset can be deployed on target robots without prior adjustments (zero-shot) or further fine-tuned, demonstrating resilience to variations in camera positioning.
The field of robotics has seen numerous advancements in recent years, but one challenge has consistently stood out: the difficulty in training robots to perform tasks. Unlike humans, who can learn from observation and adapt to new situations, robots require extensive programming and manual adjustments to perform even the simplest tasks. However, a team of researchers from UC Berkeley have recently developed a revolutionary framework that is changing the game for robot training, and the implications are enormous.
Key Things to Know:
- UC Berkeley researchers have developed ROVI-Aug, a groundbreaking framework enabling robots to train each other autonomously, eliminating the need for human intervention.
- The new system leverages advanced generative models and simulation-based augmentation to create diverse datasets, improving robots' ability to adapt to real-world complexities.
- ROVI-Aug addresses major challenges in robotic learning, such as data scarcity and the limitations of static datasets, making training processes faster and more efficient.
- While promising, the technology faces challenges such as ethical considerations, data transparency, and addressing training biases for future implementations.
What challenges do robots face with training, how has the new framework helped to address this, and what does the future of robotics look like?
The Challenges With AI Training
Training AI systems has never been a trivial issue. While the fundamentals of training an AI are actually somewhat simple, the vast amount of data needed and the need for specialist hardware makes it a time-consuming and expensive operation. However, even when trained, AIs can require improvement over time.
Complexities in Robotic Task Training
With regards to robots, training robotic systems to perform complex tasks introduces many challenges. Controlling robot actuators and limbs is easy, but trying to convey complex actions such as cracking open eggs, picking up delicate objects, and identifying things of importance is hard. There are many datapoints that need to be gathered, and modern sensors just don't have the resolution needed.
Furthermore, trying to identify the most important data points can be a challenge in its own right. To make matters worse, the vast majority of modern sensors are designed to be low-cost and mass-produced. While this is great for everyday consumer applications, it is not ideal for applications that require high precision and accuracy. In order to improve the capabilities of a robot, it is essential that the most important data points are identified and used to train the AI.
Data Quantity and Curation Issues
However, this is not a simple task, and there are many different challenges that need to be overcome. One such challenge is the need for large amounts of data. In order to train an AI, it needs to be presented with a large amount of data, and this data needs to be carefully curated. If the data is not carefully curated, it can be difficult to get the AI to learn the correct patterns and relationships. Another challenge is the need for high-precision sensors.
As mentioned earlier, modern sensors are not designed to be high-precision, and this can make it difficult to get accurate readings. In addition to the need for high-precision sensors, there is also the need for specialist hardware. While it is possible to train an AI using a standard computer, it is not the most efficient way to do so. This is because standard computers are not designed for AI training, and they can be very slow.
New Framework Allows Robots To Train Robots
In a new development that could alter the field of robotics, a team of engineers from UC Berkeley has introduced a novel framework that enables robots to train each other autonomously without human intervention. The advanced system, called ROVI-Aug, simplifies robot training by bypassing the usual manual adjustments and enhances the robots' ability to perform tasks more efficiently.
The ROVI-Aug system employs advanced generative models to achieve robust task generalisation. By using tools like ControlNet and ZeroNVS, it can synthesise diverse robotic embodiments and camera perspectives. This approach ensures that training data includes a wide range of scenarios, enabling robots to better adapt to real-world complexities.
Addressing Bottlenecks in Traditional Robotic Learning
According to the researchers, ROVI-Aug is a major improvement over previous methods that rely on static datasets and require test-time adjustments, which have been a major bottleneck in traditional robotic learning. The new framework addresses this limitation by focusing on how robots interact with tasks within their data, generating demonstrations from various robot types, and simulating different camera angles.
One unique feature of ROVI-Aug is its ability to leverage simulation-based segmentation and augmentation. This allows the framework to create synthetic datasets that mimic real-world conditions without extensive physical experimentation. The augmentation process ensures that tasks performed by robots in controlled settings can seamlessly transfer to unstructured environments.
Transferring Skills Across Robots
"We have developed a framework that can teach one robot to perform a task and then transfer that skill to a different robot," said Dr. Abhishek Gupta, the lead author of the research paper. "This is a major step forward in the field of robotics, as it allows for more efficient and autonomous learning."
The ROVI-Aug framework consists of two primary components: the Robot Augmentation (RO-Aug) module and the Viewpoint Augmentation (VI-Aug) module. The RO-Aug module generates demonstrations from various robot types, while the VI-Aug module simulates different camera angles. Together, these modules create a richer and more diverse dataset that allows robots to learn more efficiently and apply skills across a range of different models and tasks.
One of the major challenges in robot learning is the scarcity of diverse, high-quality data. Although scaling up data has been shown to improve generalisation in AI models for vision and language, robots face a unique challenge: gathering real-world robot data is slow and labour-intensive. ROVI-Aug addresses this limitation by focusing on how robots interact with tasks within their data, generating demonstrations from various robot types, and simulating different camera angles.
The new framework also overcomes the limitations of previous approaches that required precise robot models and struggled with camera angle variations. ROVI-Aug does not rely on known camera matrices and supports policy fine-tuning, making it more adaptable for complex tasks involving multiple robots.
Creating Autonomous Feedback Loops
Unlike traditional methods that rely heavily on manual fine-tuning, ROVI-Aug introduces an autonomous feedback loop in robotic learning. This loop enables robots to iteratively refine their skills by evaluating task performance in various simulated conditions. This continuous improvement mechanism bridges the gap between static training environments and dynamic real-world applications.
While the system shows great promise, the researchers note that there are still challenges to address, such as improving background handling and extending the framework to support more diverse grippers. However, ROVI-Aug represents a major step toward creating more autonomous and versatile robots.
Future iterations of ROVI-Aug aim to incorporate multi-modal inputs, such as tactile and auditory data, to further enhance task precision. By integrating these sensory modalities, robots can achieve a more nuanced understanding of their environment, paving the way for advanced applications in healthcare, logistics, and disaster response.
Expanding the Scope of ROVI-Aug
"We are excited about the potential of ROVI-Aug to change the field of robotics," said Dr. Pieter Abbeel, a co-author of the research paper. "By enabling robots to train each other autonomously, we can create more efficient and adaptable systems that can learn and improve over time." The team's research has been pre-published on arXiv and is currently under review for publication in a peer-reviewed journal. As the research continues to evolve, it is likely that ROVI-Aug will play a crucial role in shaping the future of robotics and automation.
As ROVI-Aug progresses, ethical considerations such as data privacy and bias mitigation are being actively addressed. By adhering to transparent development practices and including diverse datasets, the framework aims to set a benchmark for responsible robotics innovation. These efforts are crucial in building public trust and ensuring the technology benefits all stakeholders.
Future of Robots Training Robots
One of the most crucial benefits of this development is the potential for accelerated innovation. By allowing new models to build on the knowledge and experience of their predecessors, engineers can focus their time and resources on developing new features and capabilities rather than spending countless hours figuring out how to train a new system from scratch. This not only saves time and money but also enables engineers to focus on more complex and challenging projects, leading to faster advancements in the field.
Another major advantage of autonomous robotic training is the reduction of labour costs associated with training and maintenance. Engineers, who are highly skilled at their jobs, are also incredibly expensive, and their time is often better spent on high-level design and development work. By automating the training process, engineers can focus on higher-level tasks, such as developing new algorithms and protocols, while robots handle the grunt work of learning and adapting to new environments.
Challenges of Habit Persistence in Robotic Learning
However, as with any new technology, there are also potential challenges that need to be addressed. One of the biggest concerns is the potential for bad habits to persist in future designs, leading to unforeseen consequences. If a robot is trained to perform a task in a particular way and that training is then passed on to future models, it is possible that those models will also exhibit the same habits, even if they are no longer desirable.
For example, if a robot is trained to pick up objects in a certain manner but that training is later found to be flawed, future models might continue to exhibit the same behaviour, even if it is no longer optimal. This could lead to a situation where robots are performing tasks suboptimally simply because they have been trained to do so.
Transparency Issues in Autonomous Training
Another challenge that arises from the use of autonomous robotic training is the potential for a lack of transparency and understanding. If a robot is trained to perform a task without any human intervention, it can be difficult to understand why it is behaving in a certain way. This lack of transparency can make it challenging to identify and address potential issues, as well as to develop new training protocols that can help improve the robot's performance.
Overall, the ability of robots to train other robots presents a wide range of exciting possibilities but also introduces several challenges that need to be addressed. As the technology continues to evolve, it is likely that new applications and uses for autonomous robotic training will emerge, but it is also probable that challenges will need to be overcome.
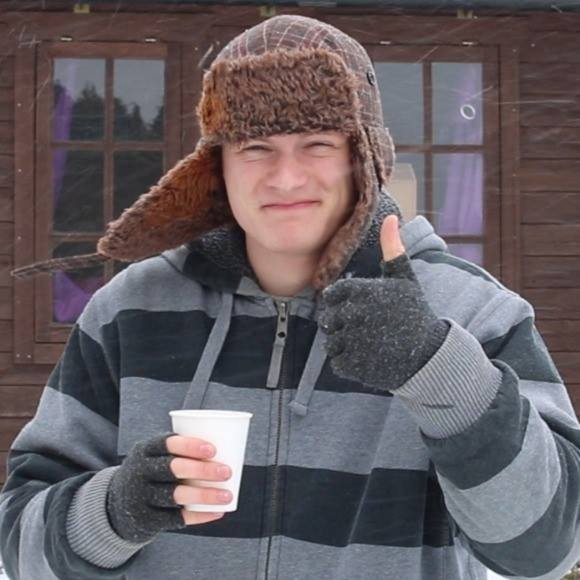