AI in Drug Development: Faster, Cheaper, More Effective
14-02-2025 | By Gary Elinoff
3 Dimensional Structure of Q8I3H7, a protein which may protect the malaria parasite against attack by the Immune system. Image source: AlphaFold
Key Takeaways about Drug Discovery Fast Tracked by Artificial Intelligence
- Bringing a candidate drug to market often takes 10 years and a $2 Billion Dollar investment.
- The application of AI in drug development leads to more cost-effective drug discovery processes.
- Implementing AI in drug development has resulted in over 80% of these drugs successfully passing phase 1 clinical trials.
- In drug development, AI facilitates a more efficient and cost-effective understanding of the three-dimensional structures of relevant proteins.
- AI in drug development works alongside laboratory efforts to enhance research efficiency
Introduction
As described by Genentech[1], "Making new medicines is complex. It is a time- and resource-intensive process that often results in limited success. Industry-wide, about 90% of drug candidates fail, and it can take more than 10 years to find out if they will even work." The integration of AI in drug development aims to streamline this complexity, offering more efficient pathways to new treatments.
Not a very encouraging scenario and the scope of the problem is incredibly daunting. The article points out that there are about 20,000 medical conditions that need to be addressed, and worse still, the number of small molecules that must be considered as possible therapeutic agents "far exceeds the number of stars in the universe."
There aren't enough grad students in the world, working 80 hours a week, 50 weeks a year, to test every possible small molecule against every medical condition. This treasure hunt is only made possible through Artificial Intelligence and Machine Learning.
How AI Smooths the Path towards Drug Development
As described by the Association of Cancer Care Centers[2], "Traditional drug discovery is a complex, time-intensive, and costly undertaking. To bring a single drug to market, efforts typically span over a decade and incur an average cost exceeding $2 billion. Each stage—from target identification and validation to preclinical testing and clinical trials—is marked by immense trial-and-error experimentation with huge sunk costs along the way."
Only 40% to 65% of traditionally developed drugs pass phase one clinical trials. The odds greatly improve for AI-developed drugs, with 80% to 90% passing this first vital hurdle.
The article goes on to enumerate three ways in which AI streamlines drug development.
- Target Identification and Validation. The starting point is a complete analysis of the human genetic codes of all 23 chromosomes. Once this is done, machine learning (ML) can predict the interaction of the candidate drug with the human genetic target.
- ML can be used to identify toxicological patterns such as potential heart or liver damage. This will serve to either eliminate the drug candidate or perhaps limit its use only to non-affected humans.
- Drug Repurposing. Sometimes, a drug can be found to be useful for treating conditions that it wasn't initially designed for. AI can scrutinize huge datasets far too vast for human analysis to search for totally unanticipated efficiencies.
But, as we'll see, the first step in the process of employing AI methodologies is to gain knowledge of the three-dimensional structure of the target molecule.
The Three-dimensional Structures of Proteins
Analyzing a protein and determining the order in which perhaps thousands of amino acids connect to each other is no simple feat. But that's only the beginning because protein chains fold in on each other, forming very complex three-dimensional arrays. And, because real-life proteins exist in three dimensions, their three-dimensional structure, as well as that of the potential drugs that will target them, must be plotted and understood.
Here's why.
The relationship between errant proteins and the drugs that combat them can be thought of as a three-dimensional "lock and key" arrangement. The left-hand side of the image below is a simple two-dimensional illustration of a key (top) fitting into a lock (bottom).
Image source: Brookhaven National Labs
More interesting, from our perspective, is the illustration on the right. Here a three-dimensional "lock", the errant, target protein, is shown as exactly matching up with the three-dimensional "key", the potential drug. The "Key" has found its "lock".
AlphaFold is an AI program developed by Google and its DeepMind subsidiary. This groundbreaking program is able to predict the 3D structure of a protein from nothing more than its linear amino acid sequences. As stated in an article by Google DeepMind[3], "Before AlphaFold, predicting the structure of a protein was a complex and time-consuming process."
And that's not all. "AlphaFold's predictions, made freely available through the AlphaFold Protein Structure Database, have given more than 2 million scientists and researchers from 190 countries a powerful tool for making new discoveries."
For their work on AlphaFold, Google DeepMind's Sir Demis Hassabis and Dr. John Jumper were co-awarded to the 2024 Nobel Prize in Chemistry.
The Lab In a Loop – a Powerful Synergism
As described in an article by Roche[4], Genentech, a member of the Roche Group, describes its "lab in a loop" methodology, whereby "data from the lab and clinic are used to train AI models and algorithms designed by their researchers, and then the trained models are used to make predictions on drug targets, therapeutic molecules and more. Those predictions are tested in the lab, generating new data that also helps retrain the models to be even more accurate. This streamlines the traditional trial-and-error approach for novel therapies and improves the performance of the models across all programmes."
Lab in a Loop. Image source: Roche
As illustrated above, a "virtuous circle" between the lab, the AI model, real-world testing and then back to the lab.
Additionally, with AI and ML, it is possible to test thousands of neoantigens (proteins generated by tumour-specific mutations) as targets for potential cancer vaccines. And by choosing an individual patient, drugs aimed at the specific individual can be developed, opening up the exciting possibility of personalized medicine.
Outside the Lab
As noted by the US Army Medical Research and Development Command[5], "AI can also be used to streamline patient recruitment for clinical trials by using algorithms to scan genomic data and health records to match eligible patients, which can take years using traditional labor-intensive data collection and analysis techniques."
And, on the bureaucratic side, AI can also scan for regulatory hurdles that must be dealt with and to help smooth the pathway towards FDA regulatory approval.
There are also security and concerns about bias. "We need to ensure that AI algorithms are free from bias and discriminatory outcomes, that privacy and security measures are in place to protect sensitive information, that there is transparency in AI-driven discoveries, that strategies developed by AI platforms are accessible and affordable, and that there is a robust framework for regulatory oversight."
Challenges and Opportunities
There are hurdles to overcome, not the least of which is bad input data. There is also the issue of cooperation between universities, governments, and particularly private, for-profit drug companies. The latter, of course, are in business to make money and will be loath to share proprietary data that cost them tens of millions of dollars to develop.
As for opportunities, as of early February in 2025 the largest one looming on the horizon is DeepSeek. This Chinese development is said to be able to achieve AI goals without the use of expensive, massively power-hungry chips such as those available from NVIDIA. Pundits have pointed out possible limitations to DeepSeek, so only time will tell whether it will have applicability to drug development.
There is a thin line separating the use of open-source information to reverse engineering, but we'll leave that issue to the lawyers. If Deepseek is merely a simple fork of existing US technology, the question is, why didn't Elon and Zuck et al. see the possibility - were they asleep at the switch?
No matter, because what's good for the goose is good for the gander, there is no doubt that US-based powerhouses will soon have similar offerings.
Wrapping Up
In previous Electropages articles, we've explored AI's impact on space, the military, E-commerce and agriculture. This new technology's impact on AI in drug development is already proving to be equally dramatic.
The time and money it takes to ascertain the 3D structure of a complex protein or a patient's genome has been vastly sped up, which in turn smooths the pathway to drug development. It also can predict the impact of a potential solution on other body systems, leading to the early elimination of solutions that have unexpected toxicities.
It can also analyze a safe, tested drug to see if it will have a beneficial effect on human disease conditions for which it wasn't originally purposed. In essence, drug companies and patients enjoy what amounts to two solutions for the price of one.
Most importantly, AI doesn't eliminate laboratory work; rather, it creates a "virtuous cycle." Results from the lab are "fed" into the AI model, improving it. The lab scientists incorporate those results into their research, which results in still more enhancements for the AI. Eventually, once safety is assured, patients and their data become part of the "loop", and still greater results are obtained.
And, of course, the possibilities enabled by DeepSeek are as yet unknown, but there is reason to be very optimistic.
AI has come a long way. For a broader perspective on how it all began—and where it’s going—check out our feature on the history of AI.
References
- Redefining Drug Discovery with AI. Genentech
- Harnessing Artificial Intelligence in Drug Discovery and Development. Association of Cancer Care Centers
- Demis Hassabis & John Jumper were awarded the Nobel Prize in Chemistry. Google DeepMind
- AI and machine learning: revolutionising drug discovery and transforming patient care. Roche
- Artificial Intelligence Can Help Researchers Develop New Drugs, MRDC Regulatory Experts Forecast. US Army Medical Research and Development Command
Glossary of Terms
- Phase 1 Clinical Trials. Determines safety, side effects and dosage of a drug being tested.
- Silico Modeling. Where computer-based analysis is employed to develop biological models.
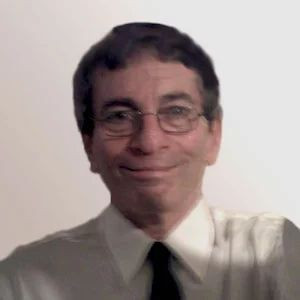