AI Solves 10-Year Problem Using Co-Scientist Technology
03-04-2025 | By Robin Mitchell
While early AI systems were once limited to basic data analysis and pattern recognition, the surge in demand for more efficient and comprehensive research solutions has propelled scientists to explore new frontiers in AI technology. Recently, a breakthrough at Imperial College London illustrated AI’s remarkable potential, where a novel tool from Google replicated a decade’s worth of microbiology research in just two days—showcasing the transformative power of AI-driven discovery.
Key Thing to Know:
- AI co-scientist tool by Google helped Imperial College London researchers replicate a decade of microbiology research in just two days, offering credible hypotheses and new lines of inquiry.
- Benchmarking the AI’s accuracy against known experimental data demonstrated its potential to accelerate discovery and reduce research dead ends.
- Researchers see game-changing potential in using AI for complex biomedical challenges like antimicrobial resistance (AMR), with early trials integrated into the Fleming Initiative.
What challenges does the scientific community face in adopting AI, what innovative methods have researchers introduced to address these obstacles, and what are the broader implications of these next-generation AI tools for the future of scientific inquiry?
The Evolution of AI in Scientific Research: A Path of Growth and Challenges
The integration of Artificial Intelligence (AI) into scientific research has undergone a major change over the years, evolving from its early beginnings as a novelty in research methodologies to a mainstream tool that has transformed the way scientists conduct their research. From its initial applications to its current widespread use, AI has not only enhanced the efficiency and accuracy of scientific research but has also opened up new avenues for discovery and exploration.
Historically, AI has been employed in various scientific disciplines, including astronomy, biology, and physics, to aid in data analysis and pattern recognition. In the early 1990s, AI was primarily utilised for simple tasks such as data mining and predictive modelling. However, as computing power increased and algorithms improved, AI began to take on more complex tasks, such as image recognition and speech processing. The development of machine learning algorithms in the 2000s further accelerated the integration of AI into scientific research, enabling researchers to create sophisticated models that could learn from large datasets and make predictions based on complex patterns.
Energy and Environmental Trade-offs of AI Adoption
Despite the numerous benefits offered by AI, its widespread adoption in scientific research has also raised concerns about its high energy demands and environmental impact. As AI systems rely on complex algorithms and large amounts of data to operate, they require substantial computational resources, resulting in significant energy consumption. This, in turn, contributes to greenhouse gas emissions and climate change, making it a pressing issue for researchers to develop energy-efficient AI technologies. Moreover, the high energy requirements of AI systems also pose a challenge for researchers working in remote or resource-constrained environments, where access to computing infrastructure may be limited.
Another significant challenge facing AI in scientific research is its limitations in learning and adaptability. While AI systems have made significant strides in recognising patterns and making predictions based on historical data, they often struggle to adapt to new situations or learn from novel data without human intervention. This limitation is particularly problematic in research settings, where data may be constantly changing or evolving.
As a result, researchers must often rely on human intuition and expertise to guide AI systems and ensure that they remain relevant and accurate in their predictions. Furthermore, the complexity of designing AI systems capable of autonomous learning and adaptation presents a significant technical challenge, requiring researchers to develop new algorithms and techniques that can effectively leverage large datasets and complex computational models.
Ethical and Societal Risks in AI-Driven Research
The integration of AI into scientific workflows also raises significant ethical and societal implications that need to be carefully considered. The use of AI in research can have far-reaching consequences, from influencing public policy to impacting individual lives, and this can be potentially disastrous if the underlying AI is flawed.
For instance, AI-driven research in genetics and biotechnology could raise concerns about privacy and data protection, while AI-generated research results in the fields of medicine and healthcare could have serious implications for patient outcomes and treatment options.
AI Does 10 Years of Research in 2 Days
This week, Imperial College London researchers have discovered that a new AI tool, developed by Google, has been able to solve a decade-long problem in microbiology in just two days. The tool, called "co-scientist," is designed to help researchers find potential solutions to complex problems, and the researchers from Imperial College London have described it as "spectacular."
Benchmarking AI Against a Decade of Scientific Research
Imperial researchers adopted an unconventional method to evaluate the AI’s effectiveness, posing a question for which they already knew the answer through years of laboratory research. This approach allowed them to benchmark the system’s accuracy in generating credible hypotheses—demonstrating not only computational capability but also contextual relevance in a real-world biomedical research setting.
The researchers, led by Professor José R Penadés, had spent over a decade trying to understand why some superbugs are resistant to antibiotics. After years of research, they finally discovered that the superbugs are able to form a tail from different types of viruses, which allows them to move between species. This tail, or "key," as Professor Penadés described it, enables the superbugs to move from one host to another, making them highly infectious.
According to Dr Tiago Dias da Costa, a co-lead in the experimental work, the challenge in traditional biomedical discovery lies in navigating ‘experimental dead ends’. The AI's ability to converge on the correct conclusion, while offering viable alternative interpretations, represents a substantial leap in accelerating discovery and minimising wasted research effort.
Beyond Validation: AI as a Generator of New Scientific Hypotheses
However, what was even more surprising to the researchers was that the AI tool was able to arrive at the same conclusion in just two days. In fact, the AI tool provided not only the correct hypothesis but also several alternative explanations that all made sense. One of these alternative explanations was something that the researchers had never considered before, and they are now working on it.
This alternative explanation, now forming the basis of new investigative work, exemplifies how AI may contribute not just to validation but to ideation—suggesting new lines of inquiry that researchers may not have previously considered. Such capabilities may foster interdisciplinary collaboration, as AI systems can access vast literature and datasets across fields.
The researchers have expressed their amazement at the tool's capabilities, with Professor Penadés describing it as "the opportunity to be playing a Champions League match." He also mentioned that he was shopping with someone when he discovered the AI tool's findings, and he was so shocked that he asked Google if they had access to his computer. Google confirmed that they did not.
The Future Role of AI in Global Biomedical Collaboration
The AI platform operates by synthesising inputs from published studies, research papers, internal datasets, and search-indexed content to propose hypotheses. Google’s researchers emphasise that the system is not designed to replace human scientists but to augment their workflow—helping them 'do more with less' by optimising research processes.
The researchers have also stated that the tool could have saved them years of work if they had access to it at the beginning of their project. They have also expressed their enthusiasm for the potential of the tool, with Professor Penadé saying that it will "change science."
Imperial College London has stated that the research forms part of its ongoing partnership under the Fleming Initiative, focusing on antimicrobial resistance (AMR)—a pressing global health threat. The integration of AI in this domain is expected to expedite the discovery of diagnostics and therapeutics, contributing to strategic international efforts in combating AMR-related mortality and hospital burden.
Envisioning AI's Next Frontiers in Scientific Discovery
The integration of AI into scientific discovery has been one of the most significant advancements in recent years. From replicating years of research in a few days to unlocking new discoveries in fields such as disease prevention, AI has proven to be a game-changer in the scientific community. As researchers continue to push the boundaries of AI, engineers are pioneering innovative hardware and software designs to refine algorithms and computational infrastructure capable of handling unprecedented scales of data analysis across all scientific domains.
However, with the increasing reliance on AI comes a range of emerging issues and risks that must be addressed. For instance, the heightened dependency on automated systems introduces vulnerabilities such as data security threats and misinterpretations, making it crucial to implement responsible planning and transparent processes to maintain public trust.
The transformation of research methodologies by uncovering overlooked patterns and alternative explanations also necessitates a shift towards more collaborative research models that combine AI's efficiency with human creativity. By harnessing AI's predictive power, researchers can unlock groundbreaking treatments and inventions, advancing healthcare, environmental stewardship, and the broader pursuit of solving humanity's most pressing challenges.
As AI continues to evolve, it is essential for researchers to navigate the complexities of AI-driven research, addressing challenges such as energy efficiency, data privacy, and algorithmic transparency. By doing so, scientists can unlock the full potential of AI in driving scientific discovery and pushing the boundaries of human knowledge. The future of scientific inquiry will be shaped by the successful integration of AI, and it is imperative that researchers, engineers, and policymakers work together to ensure that AI is developed and deployed responsibly, maximising its benefits while minimising its risks.
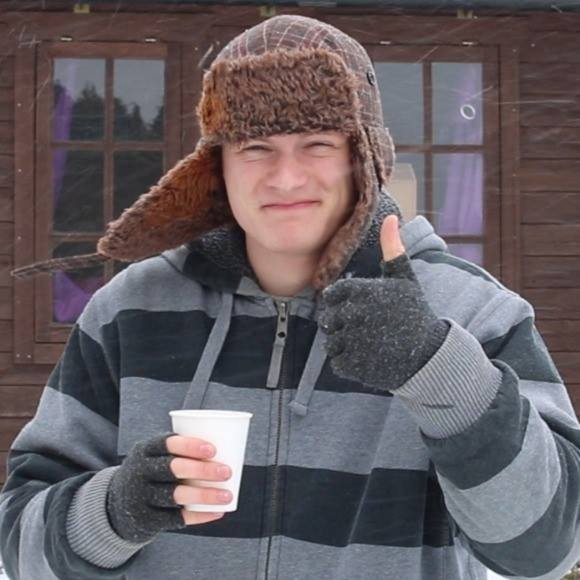