How Big Data Could Reduce Traffic Carbon Emissions
22-04-2025 | By Liam Critchley
Key Thing to Know:
- Urban road traffic is a major emitter, contributing 11.9% of global greenhouse gas emissions—around 5.88 billion tonnes annually—with congestion in cities like London and Paris generating millions of tonnes of CO₂ each year.
- China’s megacities face a growing challenge, with transport emissions rising from 96 million tonnes in 1990 to 996 million tonnes in 2021, threatening progress toward the country’s 2060 carbon neutrality goals.
- Adaptive traffic signals offer significant potential, reducing trip delays by up to 15% in Chinese pilot cities and improving urban traffic efficiency through real-time data-driven control systems.
- City-wide benefits outweigh the costs, with simulations showing that adaptive signal systems could cut vehicle trip times by 8–11% and reduce CO₂ emissions by over 31 million tonnes across China’s 100 most congested cities.
Urban road traffic is a big source of carbon emissions around the world, especially in big cities and built-up areas. Road traffic accounts for around 5.88 billion tonnes of greenhouse gas emissions (GHG) per year—which is 11.9% of total GHG emissions and third among all sectors. Urban congestion makes these emissions worse, and in major cities like London, congestion alone contributes around 2.2 million tonnes of carbon dioxide each year. To put it into perspective, it would require a forest of around 12,000 km2 (an area roughly the size of Sydney) to offset the traffic emissions in London.
Other major cities in Europe also have the same challenges with Paris congestion producing 2.8 million tonnes per year. Some other major cities produce smaller, but still high, levels of emissions with Berlin producing 0.42 million tonnes and Amsterdam producing 0.06 million tonnes per year.
The situation in Asia is also a growing issue. There is a lot of traffic congestion in major Asian cities, and road traffic emissions are starting to become a growing problem in the megacities that are emerging in China. The urbanisation rate in China is projected to increase from 62.5% in 2021 to 80% by 2050, and the increased migration to the megacities poses congestion challenges, but with so many people in one place, it does offer the chance for decarbonisation.
Currently, the situation in Chinese megacities is not looking good because congestion-related emissions have significantly increased due to higher traffic demands and constrained infrastructure that can't accommodate the increased number of vehicles on the road. Between the years of 1990 and 2021, transport related emissions in China increased massively from 96 million tonnes to 996 million tonnes—a compound annual growth rate of 7.83%—and is only set to increase in the coming years as more cars are used on the roads and the populations of megacities increase further.
The increase in urban traffic emissions is posing serious challenges to China's 2060 carbon neutrality targets and its progress towards the United Nations (UN) Sustainable Development Goals (SDGs). However, reducing the congestion in major cities could help China to work towards these goals more efficiently. For example, reducing congestion in Suzhou, Chengdu, and Harbin could reduce GHG emissions by up to 12.7%, 14.2% and 12.9%, respectively. It's thought that the advancements in data collection and processing methods could help to develop more practical adaptive traffic signals that could manage congestion more effectively and reduce urban transport emissions in the process.
Mitigating Urban Transport Emissions
There have been many different urban congestion and transport emissions mitigation approaches developed over the years that aim to reduce travel demand through more efficient urban planning policies. Common examples include traffic restrictions and congestion pricing, both of which have come into force in many major cities. These are 'avoid' strategies that try to invoke a shift in societal behaviour by encouraging people to take more sustainable travel options—such as cycling, walking and public transport—instead of driving their private vehicles. These approaches require a significant amount of investment and time to be put into infrastructure and policy changes.
There are also 'improve' strategies in place which focus on improving the efficiency of existing transport infrastructure to provide immediate congestion relief. One key example of improved strategies is adaptive traffic signals that optimise traffic flow using signal timings that can adjust dynamically. This reduces the need for expanded road capacity (as there often isn't space in many locations to build new lanes).
Adaptive Traffic Signal Developments
Adaptive traffic signals are now being used to improve traffic flow and reduce emissions, but the technology has been developed and progressed over multiple decades. The early approaches were heuristic methods, but newer systems work using machine learning algorithms, such as reinforcement learning.
At an intersection, each section of the intersection is defined as a traffic phase defined by intervals that are allocated to different traffic streams at a signal cycle. Efficient phase timing at the junction requires a precise allocation and cycle length to each phase. Conventional traffic signals (pre-timed signals) assign the intervals for each traffic phase based on historical averages but can't adapt to real-time fluctuations.
On the other hand, adaptive signals dynamically adjust the phase cycles based on the current traffic conditions to reduce wasted time on green traffic signals for one section of the junction where no cars are present and instead shift the green traffic signals to junction sections where more cars are waiting to cross the junction—improving traffic flow and reducing congestion.
There are two control methods for adaptive traffic signals: cycle-based methods and phase-based methods. Cycle-based methods maintain a consistent phase setting and require less data. They are also simpler for drivers to follow. Phase-based methods adjust phases in real-time so are more adaptable to the current state of traffic. However, high quality and timely data is often limited in many Chinese cities, so the pedestrian and local driving behaviours need to be carefully considered.
Multi-source traffic data is now available, however, collecting and processing this data is still often costly and unreliable. Therefore, there is still a need for signal timing updates that are more robust to data errors (and less dependent on data in general). Achieving this requires control algorithms becoming more compatible with the data that is available.
Simpler cycle-based algorithms—such as Webster's method—have already gained some traction in Chinese cities, with pilot projects in Hangzhou and Nanchang already reducing trip delays by 15%. The development to date shows that there's a lot of potential for data-driven adaptive control systems to remove long-standing traffic restrictions without increasing congestion. There's still a lot of scope for control algorithms to be developed and utilised to better manage traffic.
Data-Driven Approaches Improving Adaptive Traffic Lights
Data collection and processing technologies have advanced a lot in recent years and have had an impact on many industries. In transport management, these advanced data technologies have enabled multiple cities to implement adaptive traffic signals on a large scale, allowing real-time traffic adjustments to improve traffic management operations to reduce congestion.
A lot of work has been done on individual junctions, but the city-wide impacts—on congestion and emission reduction—of adaptive traffic lights have not been as widely studied. A new study has developed a new framework to assess and model the benefits of big data-driven adaptive traffic signals on a city-wide scale (cities with populations above 10 million and thousands of signalised intersections) to give insights into how effective these strategies are when implemented on large scales.
The researchers used simulations to model China's 100 most congested cities. The simulations were performed in OpenStreetMap and Gaode Map based on road data and traffic speed data, respectively. The simulations quantified how congestion and emission reductions occur at both individual intersections and on a city-wide basis. The simulations also identified any key socioeconomic and infrastructure factors that might reduce congestion and assess how cost-effective adaptive traffic signals are for major cities.
The simulations and models showed that the emission benefits of integrating adaptive traffic signals outweighs the cost of their installation and management—even in smaller cities such as Sanya, Yili, and Urumqi. In the 100 most congested Chinese cities that big-data enhanced adaptive traffic signals reduced vehicle trip times—by 11% during peak times and by 8% during off-peak times. It's estimated that this reduced trip time will yield a reduction in carbon dioxide emissions of 31.73 million tonnes.
From an economic perspective, the annual implementation cost was found to be around US$1.48 billion. However, the society's benefits, in monetary terms—which include carbon reduction, fuel efficiency improvements, and reduced travel times—amount to US$31.82 billion. Showing that the benefits outweigh the implementation costs.
The city-wide integration of advanced traffic management systems will rely on the adoption of advanced data collection and processing technologies. These adaptive traffic signal systems have shown a lot of promise for reducing congestion and promoting urban sustainability in many major cities and megacities. It's thought that city planners could use this framework to evaluate the various environmental and societal advantages of adaptive traffic signals in their cities, and it could potentially be used to inform policy shifts that look to meet transport emission goals and sustainability targets.
Reference:
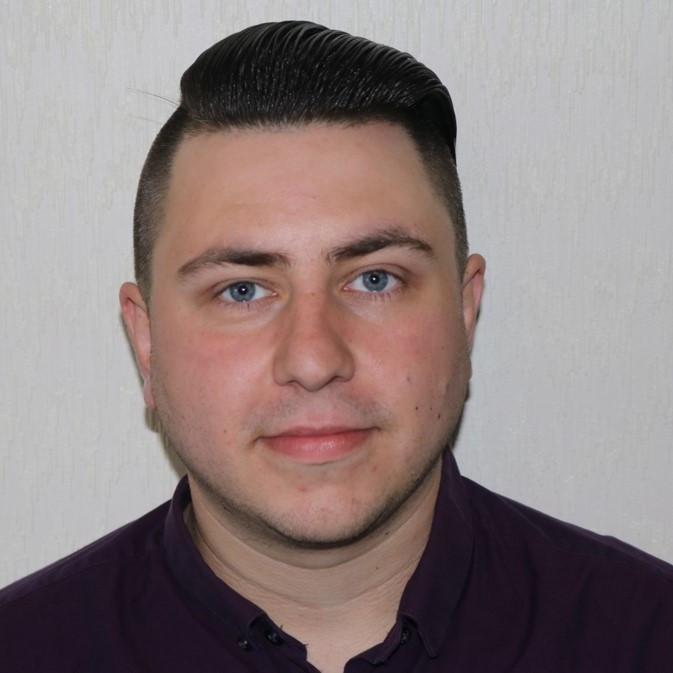