The Possibilities and Challenges of AI
04-09-2018 | By Mark Patrick
Once limited to research laboratories and supercomputers, sophisticated artificial intelligence (AI) is today becoming a key part of consumer-grade products and services, including smaller, cheaper, lower power devices and IoT products. Admittedly, in some cases, AI is employed mainly for its buzzword value, but more often it is enabling genuinely new functions and features.
Apple’s latest iPhone CPU, the A11, includes a ‘neural engine’ - two processing cores that are designed to run machine learning algorithms and provide the intelligence behind features such as Face ID unlock recognition and facial expression tracking. LG has demonstrated appliances that it says use AI - including a robotic vacuum cleaner, a refrigerator, an air conditioner and a washing machine. A US start-up, Buoy, says that its forthcoming water pump will use machine learning algorithms to optimise water flow and detect unusual situations - such as leaks - so that the water supply can be remotely or automatically shut off in an emergency.
Apple, Google, Amazon and Microsoft are among the sizeable list of companies working on a variety of AI-powered assistants - in the form of both dedicated hardware and apps - that use AI to better understand human requests and respond to them more naturally. We may not be far from the day when features of many everyday devices, such as a valve, a baby monitor, a camera, or headphones, rely to some extent on modern AI techniques that enable them to react to events and process data in a more nuanced and helpful way than their traditional dumb counterparts.
How and why is AI moving out of the lab and into the mainstream? Several trends are combining to drive this far-reaching change.
Internet, video games, and science fuelling AI revolution
The past decade has seen significant research-driven practical breakthroughs that have greatly enhanced the effectiveness of AI software. The application of deep learning techniques to training neural networks has transformed them from an interesting toy into a powerful tool that occasionally outperforms humans. The impact of this research breakthrough has been intensified by two other relatively recent developments - the vast quantity of real-world training data provided by the Internet, and powerful, low-cost, parallel processing hardware that was developed initially for 3D video game graphics but turned out to be ideal for AI.
As the benefits and practical implementations of this new AI research have filtered down from labs to industry, easier-to-use software tools have been developed, plus educational programmes and documentation have significantly improved. Developers, designers and engineers now have a better understanding of AI techniques and how to use them.
Once the value of graphics processor units (GPUs) to AI was realised, GPU makers began to work on features and software tools aimed explicitly at AI. The first wave of this trend leveraged the parallel processing capabilities of GPUs. However, the next wave includes general purpose processors running AI software, GPUs and specialised AI silicon. Apple’s new iPhone CPUs neural engine cores are one example, and both ARM and Qualcomm are working on AI-focussed processors and processor cores. These dedicated chips can naturally provide more power efficient, compact AI capabilities, suitable for mobile, IoT and embedded devices.
AI: Onboard or in the cloud?
There’s a definite trend towards putting AI onboard, when possible because this removes connectivity, latency and privacy concerns (the last is important because AI may often be processing private or personal data, such as camera and audio input). However, even when it’s not practical to build AI into a device, ubiquitous low-latency, high bandwidth Internet connectivity means almost any device can leverage the power of centralised AI in data centres. For example, mobile phone translation apps (such as Google Translate) can offload processing to central servers, personal assistant apps and devices usually do some of their processing in the cloud, and navigation apps may use a similar approach for advanced route finding.
Cloud computing providers, such as Amazon Web Services, tout the ability of virtualised GPU instances to run deep learning applications. Currently, the onus is still on the customer to provide the software that runs on these cloud services, though in a more generalised form, this trend might eventually be dubbed ‘AI as a service’. In this model, a device would send data to useful AI-based canned processing services that would be too power-intensive to run locally and receive results back in fractions of a second.
The challenges of ubiquitous AI
Companies which hope to use AI techniques in their products may be stymied by the scarcity of engineers and software developers with experience in this rapidly changing and continuously advancing field. As discussed above, this skill shortage is becoming less of an issue as time goes by - knowledge is spreading and educational resources improving. However, in the short term, hiring costs for good AI developers are likely to be higher than average, and many developers still lack practical experience.
Another significant challenge is that while AI can produce some remarkable, almost magical, results, it also brings some fundamental changes to the debugging process, produces unpredictable behaviour, and may leave manufacturers unable to guarantee that their products will always perform as expected. In fact, while any software program can go awry, AI heightens the risk that performance may be wildly outside the expected parameters.
Some developers view advanced AI as a mysterious ‘black box’ - data goes in, and decisions come out, but not even the designers fully understand how those decisions are reached, or what’s happening inside the box.
Peering inside AI’s black box
In a recent interview with IEEE Spectrum, Sameep Tandon, co-founder and CEO of self-driving vehicle software developer, Drive.ai, described the black box dilemma as “a serious problem”, but he also outlined some techniques for controlling risks, and for seeing inside the black box and debugging AI-based system. Tandon’s company builds its driving systems from separate parts or modules with distinct functions - some of which may not be AI-based - rather than creating one massive AI that drives the vehicle. This modular approach helps developers isolate and debug problematic components.
Also, the company often tests its systems with severely limited input data. For example, some image recognition tests may block most parts of a scene to focus on the system’s reaction to one detail - an isolation approach with some similarity to traditional debugging. Finally, Drive.ai combines this technique with extensive use of simulation, to test a vast range of minor variations on driving scenarios that have given their AI problems - looking for unusual behaviour and training the system to perform optimally.
Although there’s obviously room for improvement, the nature of AI, where applications may be ‘trained’ or ‘grown’ in a learning process more than they are ‘written’ or ‘constructed’ like conventional programs, means the problem of unanticipated behaviour may always remain a difficult one. For safety-critical applications, it might be necessary to add redundancy - two or more separate programs or devices that ‘vote’ to decide the best course of action, or at least monitor each other’s behaviour and warn or shut down when peculiarities are detected.
Like any fundamental shift in technology, AI promises revolutionary changes, but to make the most of its potential, designers and engineers also need to learn and to change their approach to developing products. And in fact, even end users will get more out of the powerful new tools that AI can offer them if they can learn to adapt to this new technology, rather than continuing to treat AI-enhanced products like simple old-fashioned devices. This change in perception will require well thought out design and marketing, plus strong end-user education.
Read more about artificial intelligence: Cobots – Bridging the AI Gap for Industrial Automation
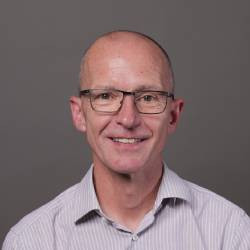