Thinking like a human is something AI will never learn
02-09-2021 | By Paul Whytock
So much has been debated about the potential power of AI (Artificial Intelligence) that there are now some humans who think it will eventually control us. I don’t believe this will ever happen, given the inherent superiority of the human mind.
In my view, the term Artificial Intelligence is a misnomer and would more accurately be called ADRs, Artificial Data Retention and Reaction Systems.
We, humans, use our brains to think. We have naturally embedded intelligence, computational power, memory, a whole range of sensory and cognitive attributes and the ability to independently think, learn, anticipate, decide and create.
In comparison, AI-powered systems rely on learning data and specific instructions that are fed into their system. Admittedly AI can imitate human behaviour and actions, but they cannot think. The bottom line is it is the ingenuity of humans that create AI systems and machines.
AI is created by pumping massive amounts of information into the neural networks that support them. They have to learn what to do, and how AI learns has become a science thanks to human intelligence.
The substantial computational resources needed to teach AI systems have powered a lot of developmental work into two types of learning processes, transfer learning and incremental learning.
Transfer learning can have speed and cost advantages. Network developing specialists can download a pre-trained, open-source deep-learning model and then customise it to suit whatever AI application they wish to create. There are many pre-trained base models to choose from, like AlexNet, Google’s Inception-v3 and Microsoft’s ResNet-50. These neural networks have already been trained on the ImageNet dataset. AI engineers only need to enhance them by further adjusting them with their own domain-specific examples.
Transfer learning negates the need for considerable computational resources. One particular trait of neural networks is they develop their reactions hierarchically, and every neural network is composed of multiple layers. Following training, each of the layers becomes tuned to recognise certain features in the input data.
According to specialists in ultra-low power high-performance artificial intelligence technology, BrainChip, transfer learning utilises knowledge established in a previously trained AI model, which is then integrated to form the basis of a new model. After taking this shortcut of using a pre-trained model, such as an open-source image or NLP dataset, new objects can be added to customise the result for the particular scenario.
However, BrainChip feels a potential weakness in this learning system is the possibility of inaccuracy. Fine-tuning the pre-trained model requires large amounts of task-specific data to add new weights or data points. It requires working with layers in the pre-trained model to get to where it has value for creating the new model. It may also require more specialised machine-learning skills, tools, and service vendors.
Repeating the Process
When used for edge AI applications, transfer learning involves sending data to the cloud for retraining. This can possibly create privacy and security risks. Once a new model is trained, the entire training process has to be repeated any time there is further information to learn. This is a frequent challenge in edge AI, where devices must constantly adapt to changes in the field.
Incremental Learning Continuity
In a nutshell, incremental learning aims to develop artificially intelligent systems that can continuously learn to address new tasks from new data while retaining the knowledge learned from previous tasks.
Many typical machine learning algorithms support incremental learning, and alternative algorithms can be adapted to facilitate incremental learning.
Some incremental learners include parameter or assumption facilities that control the relevancy of old data. Others, called stable incremental machine learning algorithms, learn representations of the training data that are not even partially forgotten over time. Fuzzy ART and TopoART are examples of this.
Incremental algorithms are very often applied to data streams or big data and can deal with issues regarding the availability of data and resource scarcity. Stock trend prediction and user profiling are some examples of data streams where new data becomes continuously available. The application of incremental learning in big data applications can increase the speed of classification and forecasting times.
It is also often used to reduce the resources used to train models because of its efficiency and ability to accommodate new and changed data inputs. One desirable aspect is that an edge device can perform incremental learning within the device itself rather than sending data to the cloud, which means it can learn continuously.
BrainChip also believes that incremental learning can begin with a minimal set of samples and grow its knowledge as more data is absorbed. The ability to evolve based on more data also results in higher accuracy.
There is also a security implication here. When retraining is done on the device’s hardware, the data and application information remains private and secure instead of the cloud retraining.
Learning is Costly
According to The MIT Centre for Collective Intelligence, recent advances in computing technology have allowed deep learning solutions to scale up to address real-world problems. Such successes have encouraged the creation of larger and larger models trained on more and more data.
Deep learning programs are computationally hungry and expensive. For example, the cost of the electricity alone that powered the servers and graphical processing units during the GPT-3 language model training is thought to be approximately $4.5million.
Moore’s Law Obsolescence
Back in the 1960s, Gordon Moore predicted the number of transistors on a chip would double every 18 months for the foreseeable future. This meant new generation chips could deliver twice the processing power for the same price. But transistors have become so small they are approaching atoms’ size, suggesting that Moore’s law may become obsolete. The computing community is working on alternative algorithmic approaches to make computation more efficient. In the meantime, training deep neural networks will remain expensive.
So there is no doubt that AI is advancing rapidly but any suggestion it will replace the human mind remains at best a suitable theme for discussion in the pub or the plot for sci-fi films featuring all-conquering robots. The best possible combination is where AI-driven machines work alongside their human controllers when it comes to achieving super-thinking and super-doing.
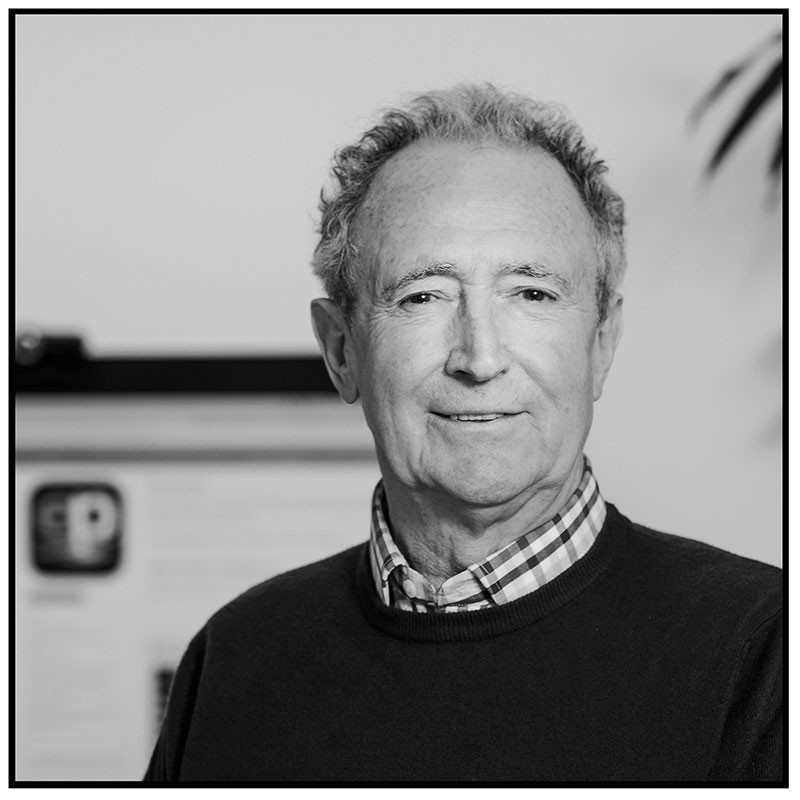