AI to train AI: Tesla fires hundreds of data labellers
11-07-2022 | By Robin Mitchell
A few weeks ago, Elon Musk stated that employees had to return to the office and that some would be let go due to more challenging economic times. But while Tesla is perusing self-driving vehicles, it just fired hundreds of people who are essential for its development which leads us to wonder if AI is being used to train AI. What challenges do self-driving vehicles face with regards to learning, what is Tesla doing, and could this be a sign of increased use of synthetic data?
What challenges do self-driving vehicles face?
Despite more than three decades of effort by researchers and engineers, self-driving cars are still a fantasy. Some have been able to get close, with one example being Waymo which runs a small self-driving taxi service, but even this has severe restrictions such as limited roads that it can use, unable to navigate around unexpected blockages and the need for frequent maintenance.
The reason why self-driving vehicles continue to present challenges comes from the need to develop an AI that is highly observant of its environment and able to categorise anything around it. Humans, for example, can be placed in any environment and understand their surroundings entirely, identifying people, trees, road markings, rocks, obstructions, and directions from other humans. Even though AI has become efficient at recognising different animals and objects, combining all of this into a single AI that can categorise everything around them requires massive amounts of processing power.
This need for categorising everything also comes with a second challenge: learning how to identify objects. Just like how humans learn, AI requires experiences and data to improve itself, and the more data that an AI is exposed to, the better it operates. But getting enough data for self-driving vehicles is challenging considering that self-driving vehicles are not allowed to operate on roads alone, nor are they allowed to make mistakes.
As such, self-driving AIs are often restricted to designated paths that experience slight variation or private racetracks that do not represent real-life driving conditions.
Tesla fires 200 staff working on Autopilot
Recently, Tesla announced that it has let go over 200 staff involved with their autopilot program, whose main job is to categorise data. Specifically, raw data (such as video and images) requires human categorisation before it can be fed into an AI training model; otherwise, the AI wouldn’t understand what it is looking at. Considering that a large amount of data is needed for AI to improve itself, it goes without saying that large teams of humans are required to process and feed such data into the AI.
Considering that the Autopilot program is Tesla’s second most famous product, it is therefore unusual that Tesla is letting go of a significant portion of its data categorisers. The exact reason why this layoff has happened is not apparent and varies depending on who is asked. Some reports mention that Tesla is looking to shift work to other offices, while others will report that increased cost of living and manufacturing difficulties are pushing Tesla to save money. At the same time, there are reports of more investigations by authorities into Tesla self-driving systems already in use by motorists after numerous accidents caused by the reckless use of what is essentially an advanced adaptive cruise control.
However, it is also possible that Tesla is shifting towards synthetic data to train their AI. Unlike real-world data, synthetic data is artificially created data from simulations that can be made hype realistic. The advantage of synthetic data is that it can provide an AI with plentiful amounts of information already digitised and categorised by the simulation that generated the data. This removes the need for humans entirely in the AI learning cycle while reducing the amount of real-world data needed.
Could this be a sign of synthetic data becoming popular?
If an AI can be trained on synthetic data and still operate reliably, then it is likely that synthetic data will overtake real-world generated data very quickly.
As data is one of the most valuable assets in the world, training an AI can be expensive. If, however, an AI could be trained on synthetic data, then the barriers of entry to reliable AI could be significantly lowered, especially since simulations are considerably easier to generate compared to AI.
However, engineers should exercise extreme caution when using synthetic data due to the fact that it is generated in a simulation and not from real-world events. Any mistake in the underlying simulation will trickle over into the AI. Furthermore, it is not always possible to generate every likely scenario that an AI may face in the real world, and data from the real world is nowhere near as precise as data gathered from simulations.
But there are many applications where synthetic data could be highly advantageous. For example, AIs designed to interpret characters (Optical Character Recognition) could be tied into an AI that generates unique handwriting styles. As the input text to the handwriting generator is stored as digital information, a feedback loop between two AIs could be formed whereby the output of one feeds the input to another. If left alone, the OCR AI would eventually be able to recognise most handwriting styles without ever needing human input.
Overall, the large amounts of data needed to train AI will push engineers towards synthetic data, and engineers that use synthetic data will need to be cautious about how that data was generated and how reliable the underlying models are.
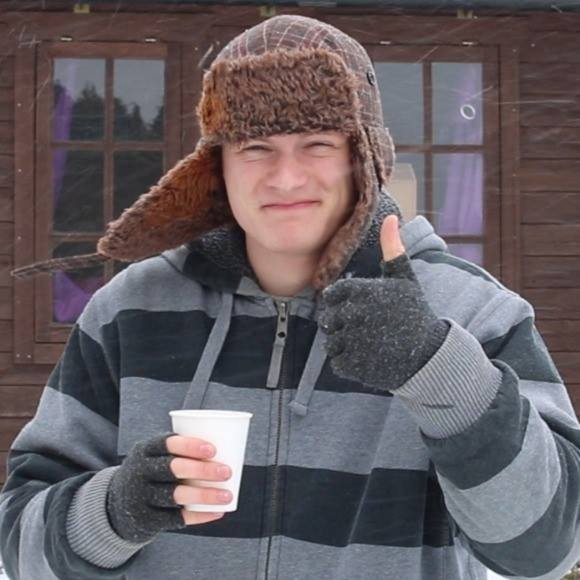