Deep Learning's Role in EV Battery Fault Detection
02-11-2023 | By Liam Critchley
As companies and governments head towards achieving net-zero emissions and decarbonisation of our transportation systems, electric vehicles (EVs) are becoming a more common sight in society. EVs that use Li-ion batteries are the most widely adopted vehicles due to the existing ecosystem in place for Li-ion batteries after years of commercial adoption. While different Li-ion systems had to be designed specifically for vehicles, the existing ecosystem has helped to accelerate their development and adoption―and the performance is much better than other systems, and they are more economically feasible.
While different Li-ion systems had to be designed specifically for vehicles, the existing ecosystem has helped to accelerate their development and adoption―and the performance is much better than other systems, and they are more economically feasible.
Li-ion Battery Safety Concerns
However, there have been a number of instances over the last few years where the safety of Li-ion batteries has been thrown into question due to several EV battery fires making the news. The challenge with EV fires is that they last longer and are more unpredictable, so EV manufacturers need to perform frequent and expensive inspections to ensure that the batteries going out into vehicles are safe for use.
As we delve deeper into the intricacies of EV batteries and their associated challenges, the following visual representation provides a comprehensive overview. Highlighting data from three prominent manufacturers, the image captures the essence of vehicle travel patterns, charging modes, and the complexities involved in fault detection. Let's take a closer look.
Challenges in Detecting Faults within EV Data Sets.
a Data from three manufacturers, Dahu, Socea, and Naobop, shows each vehicle's travel distance and time elapsed. b Real-world charging patterns vary, with fast and regular charging modes. c Traditional metrics like cell voltage and temperature variations don't clearly differentiate normal from abnormal EVs; an AUROC close to 0.5 implies random predictions. d Close examination of LiB charging from two EVs reveals no straightforward fault detection metric. (Click to Enlarge)
One of the best ways to prevent EV battery failures and save on costs is through early prediction. The ability to evaluate the safety conditions of Li-ion batteries at an early stage can reduce the chance of cell failure and further battery development. While there have been a lot of developments in AI algorithms in the last few years for battery research, detecting anomalies in battery systems has remained a challenge. This is because the anomaly detection methods are not validated or tailored for realistic battery settings because EV batteries have complex failure mechanisms, and there’s a lack of real-world testing frameworks with large-scale datasets to work with. Recent research has set out to change this.
Challenges with Data Driven Testing for EV Batteries
EV batteries are complex nonlinear systems, so designing AI algorithms that can understand these failure mechanisms is difficult―this extends to short-circuiting, physical damage, overcharge/over-discharge, and thermal abuse. Current testing approaches involve both experimental testing and data-driven analyses.
Existing algorithms require a lot of extra testing because any validations are done in small-scale lab experiments. In contrast, the success of data-driven and AI-heavy approaches in other areas―such as in gaming, molecular modelling of biomolecules and machine vision―has been because the algorithms have had access to large and real-world datasets, so they can give more accurate predictions.
The significance of real-world data cannot be understated. It provides a more accurate representation of actual scenarios and conditions, allowing for better training and validation of AI models.
Many of the algorithms in place today for battery testing rely on information that’s not available in real-world settings. To develop more effective fault detection algorithms for EV batteries, more practical social factors need to be accounted for. Existing studies have not showcased what AI algorithms―especially deep learning―can do using publicly available EV charging datasets, and these datasets do not account for how practical factors can and should dictate algorithm design.
Current anomaly detection models only make use of observational data from existing battery management systems. With the data that is available in the public databases, only a few fault detection algorithms can be utilised due to limited information regarding the vehicles―and model parameters such as open circuit voltage and internal resistance are often missing. To address this problem, a practical deep-learning framework is required that is specifically designed to detect faults in Li-ion batteries.
Using Deep Learning with a New Framework
Researchers have developed a realistic deep-learning framework for detecting anomalies in EV batteries. It has been designed to be compatible with real-world battery deployments and uses a dynamical encoder-decoder that is tailored for dynamic systems. Deep learning models, especially when tailored for specific applications like EV battery safety, can provide insights that are not possible with traditional methods. By analysing vast amounts of data, these models can detect subtle patterns and anomalies that might be missed otherwise. The algorithms have been trained on EV battery management systems and were configured using social and financial factors―including fault and inspection costs and fault rate that configure the model towards the best economic performance.
The anomaly detection algorithm was tested on datasets comprised of 690,000 charging snippets from 347 EVs. The scale of the data utilised in this study allowed the algorithms to overcome some of the challenges of other data-driven fault detection methods―including existing deep learning methods.
After training the deep learning model―known as dynamical autoencoder for anomaly detection (DyAD)―, it was deployed at charging stations, with the fault detection module being operated by the cloud. The model could maintain privacy due to the encoder-decoder structure of the system. This approach possessed a number of advantages, including the service provider being able to keep the model data, the EV customers could preserve sensitive information such as mileage, charging time, and location, and the data communication was reduced by sending over encoded partial data.
Conclusion
The model that has been developed is the first one that has been tailored towards real-world EV Li-ion data and exploits the hidden Markov model of battery data. The deep learning neural network algorithm balances both EV Li-ion accident costs against inspection costs using empirical data.
The newly developed model offers several advantages. Not only does it provide more accurate fault detection, but it also helps in reducing the economic burden associated with battery failures. By accurately predicting potential failures, manufacturers can take preventive measures, thereby ensuring the safety of the users and the longevity of the batteries.
Compared to other deep learning models used already for battery testing, this new model has a couple of key differences. The first main difference is that it utilises a dynamical system formulation to partition the data features into system inputs and system responses. Any abnormalities are then detected by input-to-response mapping. In other deep learning models, the fault detection algorithms treat each dimension of the data features equally and don’t accommodate a dynamical system that has external inputs. The other main difference is that the model bridges the predictions between the vehicle system level and the Li-ion charging snippet using a robust scoring procedure.
The algorithm was tested on three datasets with 55 abnormal vehicles, i.e., EVs that had a battery fault and 292 fully working vehicles. Compared to conventional methods and other deep learning algorithms, this algorithm was much better at detecting anomalies in the batteries. The model also showed that it could lower the costs of EV battery accidents and inspections by 33-50%.
Unlike other approaches, this study has shown that deep learning models can be more effective at predicting EV Li-ion battery fault with limited anomaly samples if the algorithm has been designed and trained with the appropriate social and financial real-world data.
The advancements in deep learning and its application in EV battery safety mark a significant step forward in ensuring a sustainable and safe future for electric transportation. As the adoption of EVs continues to grow, such innovations will play a crucial role in addressing the challenges associated with battery safety and reliability.
References:
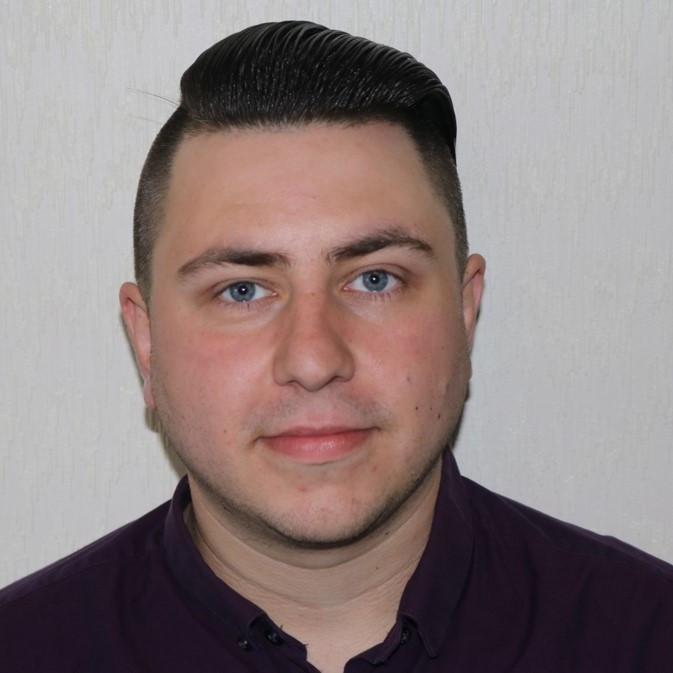