Apple Unveils M2 SoC: More Transistors, More Memory
10-06-2022 | By Robin Mitchell
Recently, Apple announced their latest SoC, the M2, which promises performance increases across the board thanks to its custom Silicon and advanced semiconductor technology. The M2 also features an AI Neural Engine designed for improved performance. This is a significant upgrade from their previous M1 SoC, which was built on an ASCI FPGA and CPLD design, unlike the M2, which is based on ARM technology. What features does the M2 incorporate, how does it compare to the M1, and how does the development of the M2 demonstrate the success of custom SoCs?
What features does the M2 incorporate?
This week, Apple unveiled their latest flagship SoC, the M2, at the Worldwide Developers Conference that is set to power the next generation of Apple technology. While the release of the M2 has been anticipated (due to the success of the M1), it is only now that the specifications for the M2 are known.
To start, the M2 SoC utilises 5nm node technology and has an overall transistor count of 20 billion. The M2 incorporates up to 8 CPU cores and up to 10 GPU cores that both use a combined unified memory (i.e., the same memory space is used for the CPU and GPU). Additionally, the M2 also integrates a 16-core neural engine designed to efficiently execute complex AI tasks, and the neural engine's performance has increased by 40% (compared to the previous M1).
As with the M1, the M2 integrates the CPU and memory into a single package for improved performance and space efficiency. The M2 supports up to 24GB of LPDDR5 RAM and has a total memory bandwidth of 100GB/s. Furthermore, the M2 also integrates a media engine that can decode 8K HVEC AND H.264 video and support multiple ProRes streams in 4K and 8K.
The first products to debut the new processor will be the MacBook Air and MacBook Pro, which are expected to be released to the public in July 2022.
How does the M2 compare to the M1?
Back in November 2020, Apple announced the development of their first SoC, the M1, which saw a radical change in Apple computer architecture, moving from x86/x64 to ARM. While Apple had already developed multiple SoCs before the M1, these were designed for use in mobile devices, not desktop PC applications.
RISC CPUs have historically been useful for low-energy computing, but applications requiring high-performance computing almost always use a CISC CPU (i.e. AMD / Intel). Thus, Apple utilising the ARM instruction set in a high-performance environment took the computing world by surprise. More shockingly, the M1 proved to be an extremely capable platform whose performance per watt outright beat any other mobile processor available on the market.
But how does the M2 compare to the M1? Interestingly, both processors utilise 5nm node technology, but the M2 differs because it uses second-generation 5nm. This often translates into increased transistor density, reduced energy consumption, and higher silicon yields.
With regards to CPU core count, the M1 actually supports more cores, and the M1 Pro has improved CPU power over the M2, but when looking at performance per watt, the M2 provides as much as a 25% improvement. Essentially, the highest tier M1 will be able to process more instructions per second, but the M2 would be able to do the same number of instructions using less energy. Additionally, the neural engine has been improved by 40%, and the CPU overall has an improved speed performance of 18%.
However, the GPU shows one of the most significant improvements with an overall speed increase of 35%, and the memory bandwidth is double of the M1, suggesting that the architects of the M2 focused their attention on the slowest system components internal to the SoC.
Overall, the M2 shows significant improvements over the M1 while still continuing the use of different cores (performance and low-energy), unified memory, and neural engines for accelerating AI tasks.
How does the development of the M2 demonstrate the success of SoCs?
Historically, the vast majority of electronic consumer products have utilised commercial off-the-shelf components for a multitude of reasons. Firstly, off-the-shelf components have been tried and tested by their manufacturer, giving a design some degree of reliability that would otherwise not be possible with a custom silicon device.
Secondly, the costs involved with custom silicon are astronomical compared to off-the-shelf commercial devices. While larger companies have the funds to hire semiconductor engineers, research new techniques, and order millions of chips, smaller companies will certainly not have the same capabilities.
Thirdly, the development time of a device using off-the-shelf components is significantly shorter than one using a custom silicon device. Depending on the complexity of a design, a working circuit can be prototyped in a matter of weeks, while a custom chip may require months of waiting for initial prototypes.
However, many manufacturers have used programmable hardware devices such as CPLDs and FPGAs. While these are not custom semiconductor devices, their programmability allows for unique logic circuits to be designed, and the mass manufacture of these devices makes them a far more economical option for custom logic. Manufacturers looking for custom logic designs have also utilised ULAs (uncommitted logic arrays) that can be thought of as one-time programmable FPGAs. Instead of an engineer designing individual transistors, logic components are arranged in a grid that only needs to be connected together on interconnect layers.
While the use of off-the-shelf devices has served the engineering community well, the plateau of single-thread CPU performance combined with the changing nature of computing tasks is seeing off-the-shelf parts struggling to meet consumer demands. Additionally, the demand for low-energy computing demonstrates how off-the-shelf CPUs cannot provide the best solution, especially if portions of the CPU are being entirely unused.
Thus, the use of a custom SoC allows for engineers to only integrate hardware that is needed, utilise advanced energy techniques to shut down all areas of an SoC not in use, to use a combination of different processor types to switch between performance and energy modes and integrate unique digital circuitry aimed at offloading complex tasks not suited for CPUs (i.e. graphic and AI tasks).
The release of the M2 not only demonstrates the commercial success of the M1 but also demonstrates how companies may start to develop their own SoCs for custom applications.
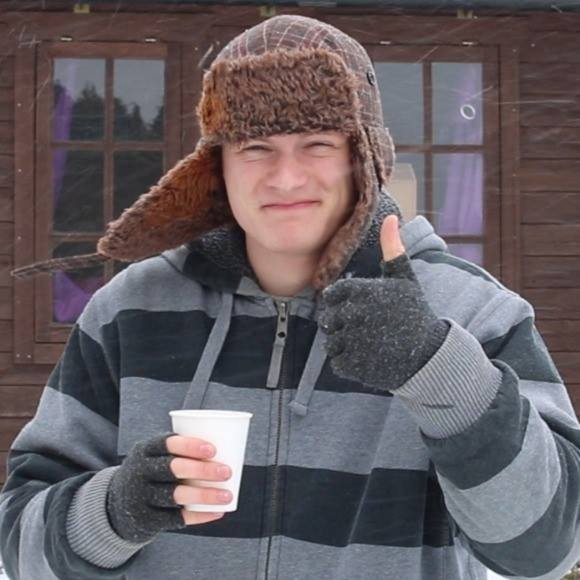