How TacticAI Masters AI Football Tactics Efficiently
07-05-2024 | By Liam Critchley
Key Things to Know:
- Strategic Importance of AI in Football: AI is enhancing football tactics by enabling deeper analytical insights and predictions, particularly in managing set pieces like corner kicks, which offer a higher degree of control over game outcomes.
- Impact on Tactical Decisions: Advanced AI tools, such as TacticAI, assist coaches in real-time decision-making by predicting player movements and potential outcomes, significantly influencing the tactical aspects of the game.
- Collaboration with Liverpool FC: The TacticAI program, developed in conjunction with Liverpool FC, focuses on optimising strategies around corner kicks, demonstrating significant improvements in tactical planning and execution.
- Broader Applications: While currently focused on football, the principles and technologies used in TacticAI have potential applications across various sports, enhancing strategic planning and player performance through data-driven approaches.
Football (soccer for the Americans reading this) is the most popular sport globally and attracts a lot of money each year. While you’d be hard-pressed to find someone who doesn’t have a basic understanding of what football is and how it works, it essentially boils down to two teams of 11 players trying to score more goals than the other team.
Like many team sports, football is a highly strategic and tactical sport, and while fitness, individual skill and talent, and a little bit of luck all play a part on the field, off-the-field tactical decisions also play a major part—and tactical changes throughout a game can swing the momentum of a game and completely change the outcome. There have been many instances over the years (and it happens every year in the Premier League, English Cups and European Cups) where a team that is significantly worse on paper has caused a major upset due to the ‘better’ team being out-coached and out-strategised.
Naturally, players also need to perform to a certain standard to win, but tactical decisions by the management team often dictate how individuals will play as well. Many areas of modern-day sport have become data-driven affairs, and the reliance on post-match analyses to identify tactical patterns and playing styles from rival teams has become a vital part of every professional team’s mid-week training. This data is also used to determine the key player formations and choices for each match, as well as for developing counter-tactics for the next opponent. Given the reliance on data and data analysis, it was only going to be a matter of time before AI was implemented to try and provide more detailed and autonomous insights for football managers and their management teams.
Set Pieces the Easiest Route for AI
Margins are tight in professional football, and clubs employ a range of on- and off-field tactics based on the expertise of their coaching team and analysts that study the opposition and devise potential counterattacks against a team’s strength.
There have been various attempts to develop AI methods that can improve tactical coaching decisions. There’s a range of data types that can be used to build AI models, including tracking sensors, logistic regression algorithms, and neural network architectures. Previous efforts to use AI have been able to use video footage to predict where the shot will go, forecast off-screen movement, and identify player actions to determine whether a match is in play or not.
However, in the grand scheme of tactics, these areas are not the most useful pieces of information that you can get from an analysis. The difficulty with using AI to plan football tactics is that the game is highly dynamic—players are constantly moving, and any preset plans are always imperfect due to the fluid nature of the game and the constant back-and-forth between the teams with unexpected events. This is then compounded by changes in player fatigue and fitness over time that cause changes in a player’s ability to move and position quickly and efficiently compared to early in the game. There are also external factors, such as pitch quality, weather, and the reaction of the opposing team, that all influence the decisions and actions on the field.
Given the dynamic nature of the game, it is difficult for AI to predict what will happen throughout the course of the game, as there is very little control over the players' on-field decisions once the game starts. On the other hand, set pieces, such as corners, penalties, and free kicks, are a more static process that’s accompanied by a break in play and a chance for the players to tactically set up their positioning and movement. Because of this, these pieces offer a much higher degree of control for predicting potential outcomes using AI.
Corner kicks have particularly gathered a lot of interest for a couple of reasons. First off, the player’s positioning is practised and pre-agreed based on the team they are playing and is based on the team’s strengths vs the opponent’s weaknesses. Secondly, corner kicks occur very frequently (over 10 a game on average), so the impact of creating a more robust tactical decision with AI is potentially much higher than set pieces such as penalties. They are also always taken from the same two positions (left and right corners), so there is very little variation in ball placement, which helps give AI algorithms more control.
The frequency and strategic importance of corner kicks make them an ideal focus for AI-driven analysis. As highlighted in research from Nature Communications, leveraging AI can lead to more precise predictions of set-piece outcomes, enhancing a team's ability to prepare and execute strategies tailored to exploit the opponent's weaknesses. This is particularly effective in planning for corner kicks, where the controlled environment allows for detailed predictive modelling.
TacticAI Program Trialled in Conjunction with Liverpool FC
Liverpool F.C. (LFC) are one of the biggest football clubs in the world today and has been for a long time with a great historical record (this coming from a Liverpool fan - so long as we ignore the end of the 2023-24 season). The researcher's AI football assistant program, TacticAI, has been developed and evaluated in conjunction with experts at LFC. Focusing on improving corner kicks was proposed as the key development area due to having a much higher degree of control over the environmental factors and inputs.
TacticAI incorporates both predictive and generative approaches, enabling the coaches to sample and explore different player setups for each corner kick routine and select the setup with the best chance of success. The data for these algorithms is taken from raw, spatio-temporal player tracking data, and represents the data for each corner kick in the form a graph. TacticAI was able to predict receivers and shot attempts, recommending the best players and pre-corner formations.
TacticAI also used geometric deep learning to produce player representations that cover several symmetries of a football pitch. This was an important part of the algorithm because there was limited data to work with, but the geometric deep learning ensured that the player representations were identically computed in many geometric reflections—so all these different points didn’t need to be learned from extra raw data.
The AI model used the position and velocity estimates of all the players, both at the time of execution and in the subsequent aftermath. From this, tracking and event was generated. Player tracking data based on video footage would theoretically increase the reach of the training data substantially, however, it would also introduce a lot of noise into the data, so the researchers stayed away from this kind of data at this time.
The TacticAI assistant was able to answer various predictive questions about the potential outcomes of a corner, including who will be the first receiver of the ball after it has been kicked and the probability of a shot happening if a particular corner setup is chosen. The AI assistant can also be used as a retrieval system for mining similar corner kick situations (using similar players and scenarios). This generative approach was able to suggest adjustments to player positions and velocities to maximise the chance of a shot taking place.
Figure 1 provides a clear visualisation of the process to further understand how AI can enhance football tactics, especially during corner kicks. Part A shows how each player's position and actions during a corner are mapped onto a graph model, laying the groundwork for strategic analysis. Part B then details how TacticAI processes these graphs, using a neural network to predict outcomes and suggest tactical adjustments. This visual aid underscores the sophistication and potential impact of integrating AI into sports analytics.
While TacticAI's focus is currently on corner kicks, the principles applied here have broader applications across various sports and tactical situations. As AI technology continues to evolve, its integration into sports analysis and strategic planning offers exciting possibilities for enhancing team performance and game understanding, paving the way for a new era of data-driven sports strategy.
The tactical variations implemented by the AI were found to be indistinguishable from human-led tactics and, according to the study, were favoured over existing tactics 90% of the time. While the study has been focused exclusively on corner kicks, the approach could be applied to other set pieces, as well as other team sports that have situations where play stops before a tactical play—a short corner in field hockey or a lineout in rugby union, for example. Perhaps it could also be used down the line for developing offensive and defensive routes in American Football (just Football for the Americans), as those formations often start from a static position (if you ignore pre-snap motions) and are all about player movement.
The world is shifting towards a more data-driven world, and AI and advanced data analyses are starting to penetrate many areas of society today. While the focus of this study was corner kicks, it lays the initial foundations towards developing the next-generation AI assistant for football teams.
Reference:
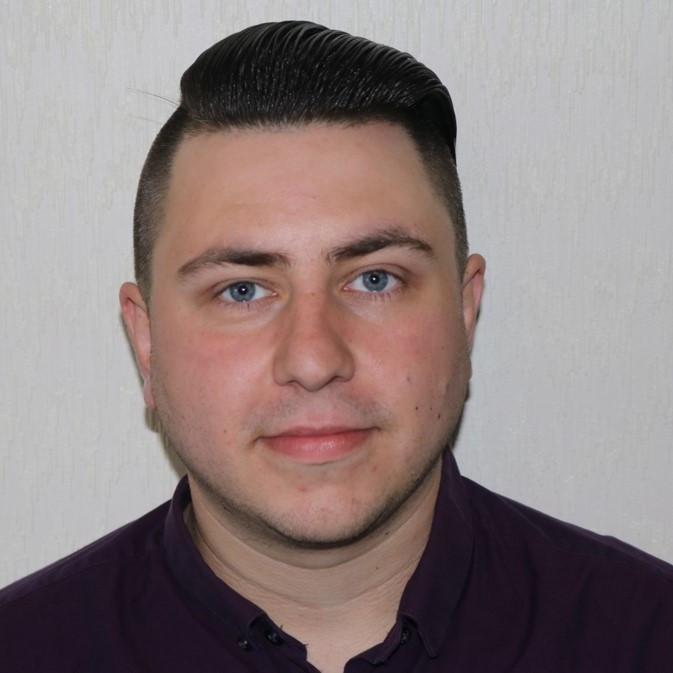