Machine Learning Predicts Osteoarthritis Risk Effectively
15-05-2024 | By Liam Critchley
Key Things to Know:
- Global Impact: Approximately 528 million people worldwide are affected by osteoarthritis, with cases increasing due to aging populations and rising obesity rates.
- Economic Burden: Osteoarthritis contributes to significant healthcare and social care costs, amounting to 1-2.5% of the gross national product (GNP) in some countries.
- Advancements in Diagnosis: Cutting-edge research, including machine learning models using UK Biobank data, is enhancing early diagnosis by identifying key biomarkers, potentially transforming disease management.
- Importance of Lifestyle: Dietary and lifestyle changes play a critical role in managing and preventing osteoarthritis, underscoring the need for public health initiatives to promote healthy living.
Osteoarthritis is a common chronic condition that affects many people around the world. It is a chronic degenerative joint disease that affects the quality of life of patients who suffer from the disease. It is estimated that around 528 million people live with osteoarthritis around the world, and it is a problem that is increasing within modern-day society.
The number of cases of osteoarthritis has been on the rise over the last few decades. Globally, the number of patients with osteoarthritis has risen by 48% from 1990 to 2019, and it is expected to increase further in the coming years. This is due to there being a larger ageing population that will start to develop the disease as they get older, but also, the diet of modern-day society has meant that there are a lot higher obesity rates around the world, and this environmental factor is suspected to cause an increase in osteoarthritis cases in the coming years.
Recent insights into the pathogenesis of osteoarthritis suggest that not only age and lifestyle but also genetic predispositions significantly impact the risk and severity of the condition. A study from the UK Biobank has utilised advanced machine learning tools to analyse comprehensive datasets, including genomics and proteomics, which allowed researchers to identify specific biomarkers linked to increased susceptibility to osteoarthritis. This emphasises the importance of a holistic approach to understanding and managing osteoarthritis, encompassing both environmental and genetic factors.
A comprehensive approach, incorporating dietary modifications and lifestyle changes, is crucial in managing and preventing osteoarthritis. Public health initiatives aimed at promoting these changes can significantly reduce the disease's burden. Recent studies have highlighted the potential of specific dietary interventions in managing osteoarthritis symptoms. Incorporating anti-inflammatory foods and maintaining an overall balanced diet can play a significant role in reducing disease progression.
Dietary and Lifestyle Interventions for Osteoarthritis
Like any disease, osteoarthritis poses a significant financial burden, as it means that there are higher healthcare expenditures and social care costs that need to be accounted for. It’s thought the economic impact of osteoarthritis alone costs anywhere from 1 – 2.5% of gross national product (GNP) in some countries, and the average annual cost for treating and helping patients with osteoarthritis is estimated to be between $700 - $15,600 depending on the country—with costs varying a lot between Asia, Europe, North America and Oceania based on how rich countries are and how much their health services cost for locals.
Osteoarthritis is also one of those diseases for which there are no curative therapies or treatments. Given that it’s a gradual health decline, many patients do not realise that they have the disease until it is in its later stages, where the only treatment available is joint replacement surgery.
The financial impact of late-stage osteoarthritis diagnosis is substantial, not only in terms of healthcare costs but also in lost productivity and personal hardship. Proactive measures, including early diagnostic interventions, could significantly mitigate these costs. Recent developments in diagnostic technology have shown promising results in identifying early markers of osteoarthritis through non-invasive methods, offering hope for earlier and more effective management strategies.
There are different strategies that can be employed to help reduce the strain on the joints and ensure a higher quality of life for longer, but because many people don’t realise that they have the disease until the latter stages, these coping strategies are no longer useful.
There’s an interest in developing diagnostic tools to identify and diagnose patients earlier on in the disease cycle so that better preventative and disease-modifying strategies can be employed. Early diagnosis is key to managing osteoarthritis effectively. Advances in biomarker research and machine learning technologies are paving the way for innovative diagnostic tools that can detect the disease at its early stages, enabling timely interventions.
The Need to Better Understand Biomarkers
One option that has been mentioned a lot and is now used in many healthcare diagnostic platforms and research sectors, is the use of machine learning. Machine learning has the ability to spot trends and learn what data points in a sample show that a person is likely to have a certain disease or is at risk of having a particular disease. It’s been widely researched in the health sector, including for detecting cancer and congenital heart disease (CHD), and it’s thought that it could also be used to detect if patients are at risk of developing osteoarthritis long before it gets to the latter stages of the disease cycle.
However, understanding the risk of patients at the fundamental level to predict a patient’s risk and susceptibility to osteoarthritis relies on the ability to detect specific biomarkers. This is where there’s a challenge because the understanding of biomarkers that drive osteoarthritis risk has remained limited. There is, therefore, a need to better understand osteoarthritis pathogenesis, but it’s not simple because osteoarthritis is a complex disease that has many biological mechanisms and disease phenotypes that underpin it.
The Challenge of Biomarker Complexity in Osteoarthritis
Understanding the diverse biological mechanisms of osteoarthritis requires a multidisciplinary approach. Recent studies, leveraging large-scale data analysis, have begun to uncover the intricate interactions between various biomarkers and the disease's progression. This research highlights the need for integrated healthcare strategies that combine clinical, genetic, and lifestyle data to predict and manage osteoarthritis more effectively.
The integration of multi-omics data plays a pivotal role in refining the predictive models for osteoarthritis. Below is a visual representation of how diverse data types were systematically combined to improve predictive accuracy.
A Overview of data integration strategy for multi-omics data. B Blueprint for the predictive machine learning model including setup phases for training and validation. C Framework for detecting osteoarthritis risk biomarkers across population, precision, and personalized risk levels through the use of interpretable AI techniques (SHAP).
It’s thought that machine learning might be able to navigate this complex biological landscape to provide accurate patient-specific prediction models. By leveraging extensive data sets, including genomics and proteomics, machine learning can uncover previously unknown risk factors and patterns, offering a deeper understanding of osteoarthritis pathogenesis and progression. However, current attempts have been very limited in their scope, and there’s a need to predict osteoarthritis for a range of joint types, whereas many studies have just looked at a single joint—for example, focusing solely on the knee joint.
Machine learning also thrives on large amounts of data to provide accurate predictions and models, but studies to date have mostly used limited data sets that make it harder to accurately predict risk outcomes.
Research utilising comprehensive patient data to model disease progression across multiple joints has further highlighted machine learning's potential in transforming osteoarthritis management. This approach not only predicts the onset of osteoarthritis but also its progression, enabling tailored intervention strategies that could significantly delay the need for surgeries such as joint replacements, thus reducing the long-term burden on healthcare systems.
Many different elements are required to make an accurate prediction of osteoarthritis risk—including genetics, clinical biomarkers, and other environmental factors—so researchers have been looking to use more relevant data and larger data sets so that machine learning algorithms can make more accurate predictions surrounding the clinical risk, and preventative strategies can be put in place.
Studying Biomarkers with Machine Learning Highlights Risk of Osteoarthritis
Researchers have now developed a machine learning model that can predict the individual five-year risk of osteoarthritis diagnosis, as well as identifying any risk biomarkers. The researchers integrated retrospective lifestyle and biomarker data from the UK Biobank to build the model. The Biobank data contained 19,120 patients with osteoarthritis.
The model used a range of data from different sources to build a complete picture of osteoarthritis risk. This included electronic health records (EHR), clinical biomarkers, self-reported questionnaire data, genomics, proteomics, and metabolomics data from different subsets of individuals. The machine learning model itself quantifies the impact of risk biomarkers with respect to the predicted osteoarthritis at both an individual and population level.
The integration of the different types of data (multi-modal data) enabled different subgroups of osteoarthritis, with different risk biomarker profiles, to be identified. 14 different subgroups were identified with risk profiles, and older age, BMI and prescription of non-steroidal anti-inflammatory drugs were found to be associated with the most osteoarthritis risk.
The subgroups were validated using an independent subpopulation of the UK biobank using data up to 11 years ahead of formal diagnosis, with 88% of patients being uniquely assigned to one of the subgroups.
The osteoarthritis risk profiles for individuals were characterised in the model by personalised biomarkers. Omics integration into the model showed a predictive importance for key osteoarthritis genes and pathways, as well as osteoarthritis-specific biomarkers.
This latest study has used large-scale predictive models to diagnose osteoarthritis based on a range of risk biomarkers. Machine learning has enabled personable and modifiable risk biomarkers to be identified, which opens the possibility of patients receiving bespoke osteoarthritis preventative strategies. Integrating these machine learning models into clinical practice could revolutionise osteoarthritis treatment, providing personalised care plans tailored to individual risk profiles and improving patient outcomes.
The outcomes of the study have the potential to advance early screening, prevention, and treatment of osteoarthritis to reduce the presence and progression of the disease.
References:
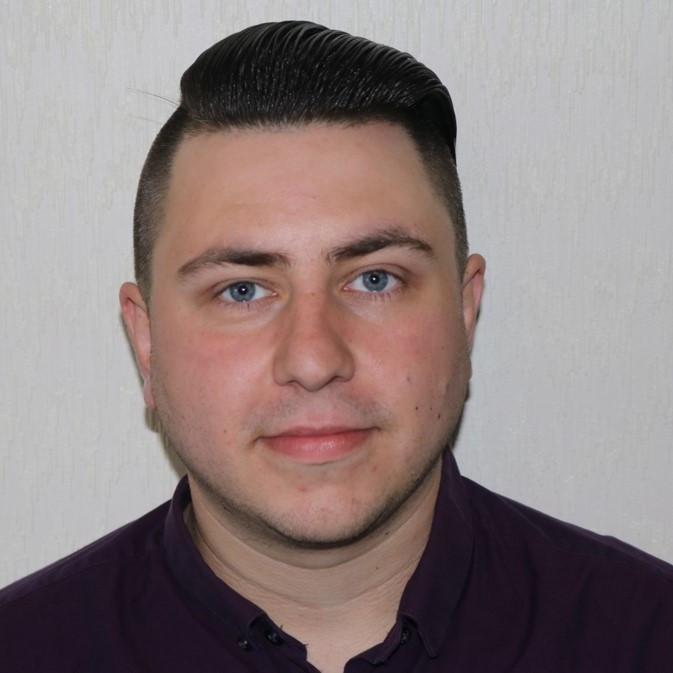