LLMs Actively Enhancing Catalysts in the Chemical Industry
05-03-2024 | By Liam Critchley
Key things to know:
- Heterogeneous catalysis plays a pivotal role in enabling chemical reactions essential for sustainable chemical development and the creation of green energy solutions.
- The integration of AI and Large Language Models (LLMs) is emerging as a transformative approach to streamline the catalysis design process, making it quicker, more efficient, and autonomous.
- Challenges in catalyst design are compounded by the academic pressure to publish, leading to an overwhelming volume of literature that hampers efficient literature review and innovation.
- Standardisation of synthesis protocols and leveraging data science, particularly transformer-based LLMs, can significantly reduce literature analysis time and improve the efficiency of discovering new catalysts.
Introduction
Catalysis is a key area of chemical research and chemical manufacturing that enables many reactions to take place―including reactions that wouldn’t otherwise work without the catalyst. Heterogeneous catalysts are the most useful type of catalysts within the chemical industry and are materials/chemicals that are in a different material phase to the reactants―with the catalyst commonly being a solid material while the reactants are liquids.
Heterogeneous catalysis is now at the forefront of sustainable chemical development and is helping to create new sustainable chemicals and technologies―such as carbon-neutral chemicals and green energy carriers from renewable feedstocks. Catalyst design is centred around exploring and refining synthetic processes to create unique and tailored catalyst architectures that have specific reactivities and will work with specific chemicals.
Keeping pace with all the scientific literature that comes out is a time-intensive process and is a landscape that is ever-changing. Automating this process is seen as the next logical step for scientists and is an attractive prospect for saving time and rapidly identifying new catalytic opportunities. This is where AI, and specifically learning language models (LLMs)―popularised recently through OpenAI and ChatGPT―can come in to help, but it is an area that has not taken off so much yet. Nevertheless, there is interest and research going into how LLMs can help to make the catalysis design process quicker, more efficient, and more autonomous.
The Challenges of Catalyst Design Lies in Current Academic Working Environments
Catalyst design is seen as a cross between art and science because there are certain subtleties that are required in the preparation methods. This takes the form of expert help in using trained chemists, but because each chemist has their own ways of working, preparing, and reacting chemicals, the result is a diverse range of protocols and catalyst formulations. These methods and catalysts are then put out into the literature for other scientists to replicate for scientific integrity and use should they wish to.
As it stands, performing literature reviews―separate papers or documents on a topic based on what papers have been published in academic journals in and around that subject―is the foundation for designing reaction-specific catalysts because it is in these papers where all the synthetic, characterisation and chemical protocol information is currently held (and that’s true for all branches of chemistry and other scientific areas). Reviewing the literature enables researchers to gain a vital understanding of different synthetic strategies and suitable catalytic active sites, as well as preventing the researcher from undertaking previously tried research.
The Evolving Academic Landscape and Its Impact on Research
However, the dynamic in academia has shifted in the last few decades. With the ever-growing number of PhD-qualified scientists entering the job market each year, coupled with fewer permanent academic positions, it has led to the situation that is known as ‘publish or perish’, which basically means if you don’t publish a lot of academic papers, then you’ll struggle to keep up with your peers for promotion and permanent contracts. This change in working dynamics has meant that the number of papers being published is increasing each year, so for those trying to perform literature reviews on the current state-of-the-art catalysis, it has become a daunting task that is unsustainable at the current rate of publication growth.
Understanding the complexity and the vast potential of LLMs, as highlighted by NVIDIA, underscores the transformative power these models hold for scientific research. LLMs, through their advanced deep learning algorithms, can recognise, summarise, translate, predict, and generate content from vast datasets, making them particularly suited for tackling the overwhelming volume of literature in catalysis research. Their ability to process and analyse data non-sequentially allows for a more nuanced understanding of catalytic processes, potentially unveiling novel catalyst designs and synthetic methods previously obscured by the sheer volume of data.
The challenge in this space involves a range of areas, from formulating new catalyst designs to applying for research grants to identifying opportunities to publishing novel intellectual property (IP) for new patents. Given the rise of publications in the catalysis space, traditional literature searches are now taking too long (especially when there is pressure on researchers to perform many other tasks) and can take several weeks or months to complete. This is highly impractical, and like many things in modern-day society, AI is there to lend a helping hand to speed up the process and create a more streamlined design process.
Turning to Data Science
Data science has become a cornerstone of modern-day science and technology and has started to be utilised in catalysis research. Text mining processes have gained a lot of traction to extract information autonomously from the main sections of material science and organic chemistry publications.
On the one hand, entity recognition models have been used to extract material properties from different chemical structures in the literature, and natural language processing and deep learning capture synthesis protocols and store the information in databases.
LLMs have become popularised this last year with the explosion of ChatGPT being used as a tool to quickly source information. While using LLMs has become an advanced research and information tool, it’s thought they could have an impact across the sciences as well. It’s thought that LLMs could be used to read hundreds of synthetic processes to provide patterns and identify any unexplored areas―and at a much faster rate than any human could. It’s also thought that the LLMs could generate data for training machine learning algorithms to screen for reaction-specific catalysts and, therefore, drive computer-assisted synthesis planning to accelerate innovation in catalyst discovery and design.
The application of transformer-based LLMs in catalysis, as explored in the study published in Nature Communications, represents a significant leap towards automating synthesis protocol extraction. This approach not only streamlines the literature review process but also sets a new standard for data reporting and protocol standardisation in heterogeneous catalysis. By converting prose descriptions into action sequences with associated parameters, the model facilitates a more efficient replication of synthesis processes, thereby accelerating the pace of innovation in catalyst design.
Fig. 2: Methodology for Text Mining in SAC Research Literature.
Fig. 2: Overview of the Process for Mining SAC Research Literature. This figure illustrates the journey from identifying SAC-related articles published between 2010-2021 to the manual categorization of experimental reports. Papers were acquired in json or txt formats with permissions from publishers. The annotation framework was established through the manual marking of 127 synthesis paragraphs, totalling 936 sentences, with 33 predefined action terms. These sentences were then allocated into training, validation, and testing segments (80:10:10 ratio) for the development of the ACE model to a level of satisfactory performance. The model's analytical output sheds light on synthesis and application trends, demonstrating its adaptability to various heterogeneous catalyst families and underscoring the importance of standardized synthesis protocols for future text-mining initiatives.
Using Transformer LLMs in Catalyst Design
Researchers have now used a transformer LLM to extract synthetic processes automatically in heterogeneous catalysis. Transformer models are a neural network architecture that can learn both context and meaning by tracking patterns in ordered data. Transformer algorithms use multiple layers that all work together to understand the input data and predict the output.
This study looked at a specific type of catalyst known as single-atom heterogeneous catalysts (SACs)―which is a class of catalytic materials that are gaining a lot of interest due to their atomic-scale structures, high metal utilisation, and unique reactivity. They have become the fastest-growing family of catalytic materials for a range of catalytic applications in recent years. However, they are a very broad class of materials with a range of synthetic processes, material properties and material compositions, so it makes it very difficult to track the field manually.
The transformer model (known as ACE) developed by the researchers relies on an encoder-decoder architecture. The ACE model was developed from a previous model and fine-tuned based on synthesis paragraphs and sentences from organic chemistry papers. The model converts the SAC protocols in action sequences with appropriate parameters and covering all synthetic parameters for the replication of the synthesis. These action sequences are then used to provide outputs based on synthetic trends and applications―such as electrocatalytic processes like oxygen and carbon dioxide reduction reactions.
The model was found to be adaptable beyond SACs and could provide accurate predictions for other families of catalytic materials. The utilisation of LLMs has the potential to significantly reduce the literature analysis time, but the model also highlights that a lack of standardisation in reporting synthetic protocols affects text mining efficiency and machine reading capabilities. The model has been released by the researchers as an open-source web application to help researchers accelerate their synthesis planning.
The challenges of data standardisation and machine readability, as discussed in the Nature Communications article, highlight a critical barrier to the broader application of LLMs in catalysis research. The proposed guidelines for writing synthesis protocols not only aim to enhance the efficiency of text mining but also serve as a foundation for developing more sophisticated and accurate models in the future. Implementing these guidelines could lead to significant improvements in the machine's ability to understand and process scientific literature, thereby unlocking new possibilities for automated catalyst design and discovery.
For the models to become more robust in the future, the researchers have proposed guidelines in their paper that could help to improve machine readability. After implementing these guidelines, the researchers compared the original model to the guideline-modified model and found a significant improvement in performance, which demonstrated the value of standardising synthesis protocols in furthering the automation of catalyst design.
Looking to the Future
The insights from the Nature Communications study into the adaptability of the ACE model for various catalytic materials underscore the importance of machine readability in the advancement of catalyst research. By standardising synthesis protocols, researchers can not only improve the accuracy and efficiency of LLMs but also foster a more collaborative and innovative research environment. This standardisation is pivotal for the future of catalysis research, enabling a seamless integration of AI technologies to expedite discovery and design processes.
If the catalysis research community and chemical industry are to benefit from the continued advances in data-driven technologies, then more effort needs to be directed towards data collection, curation, and reporting practices. These efforts can be initiated on small scales within individual research groups, but large-scale adoption and a paradigm shift from the status quo will only happen through national and international initiatives where multiple parties from the catalysis and AI fields come together to change the standard approach.
Bringing together both sides of the coin will not only help create new scientific applications for LLMs but also help automate the catalysis discovery and design process. Following on from the developments in their latest paper, the researchers are looking to make the ACE model generative in nature by standardising the guidelines in this study through prompt engineering in GPT-4 and the subsequent retraining of the model.
References:
Large Language Models Explained: https://www.nvidia.com/en-us/glossary/data-science/large-language-models/
Suvarna M. et al., Language models and protocol standardisation guidelines for accelerating synthesis planning in heterogeneous catalysis, Nature Communications, 14, (2023), 7964.
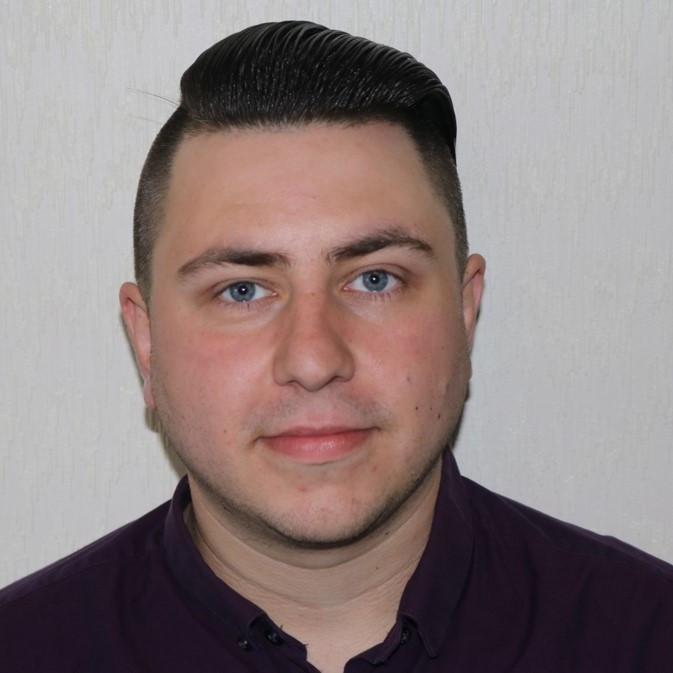